Customer-obsessed science
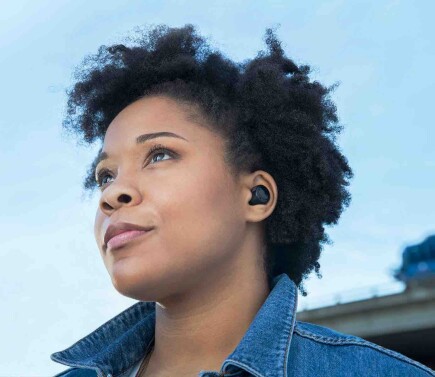
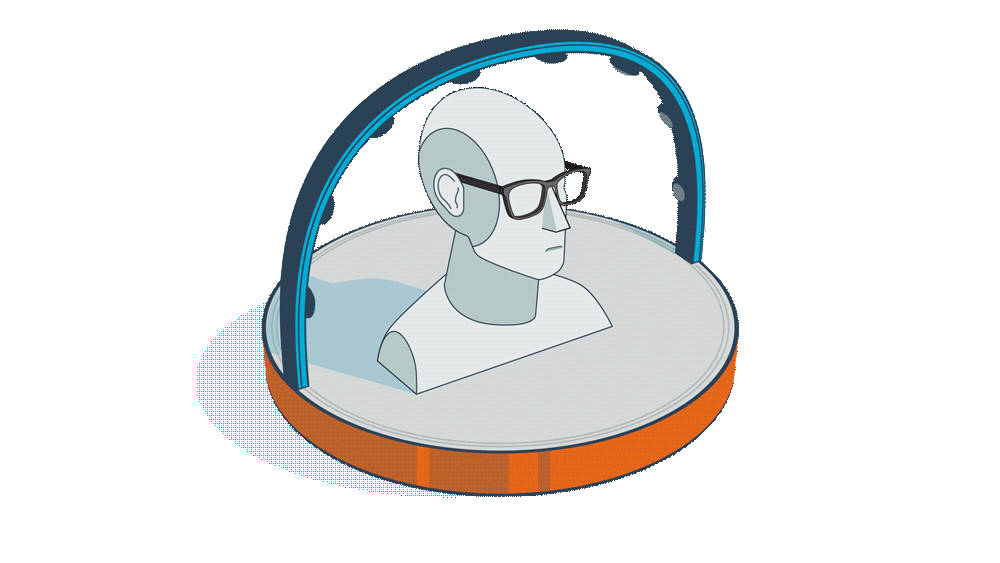
Research areas
-
February 20, 2025Using large language models to generate training data and updating models through both fine tuning and reinforcement learning improves the success rate of code generation by 39%.
-
-
-
December 24, 2024
Featured news
-
2025A small subset of dimensions within language Transformers’ representation spaces emerge as "outliers" during pretraining, encoding critical knowledge sparsely. We extend previous findings on emergent outliers to Encoder-Decoder Transformers and instruction-finetuned models, and tackle the problem of distilling a student Transformer from a larger teacher Trans-former. Knowledge distillation reduces model
-
2025At Amazon, we look to our leadership principles every day to guide our decision-making. Our approach to AI development naturally follows from our leadership principle “Success and Scale Bring Broad Responsibility.” As we continue to scale the capabilities of Amazon’s frontier models and democratize access to the benefits of AI, we also take responsibility for mitigating the risks of our technology. Consistent
-
2025Recent advancements in code completion models have primarily focused on local file contexts (Ding et al., 2023b; Jimenez et al., 2024). However, these studies do not fully capture the complexity of real-world software development, which often requires the use of rapidlyevolving public libraries. To fill the gap, we introduce LIBEVOLUTIONEVAL, a detailed study requiring an understanding of library evolution
-
2025The rise of LLMs has deflected a growing portion of human-computer interactions towards LLM-based chatbots. The remarkable abilities of these models allow users to interact using long, diverse natural language text covering a wide range of topics and styles. Phrasing these messages is a time and effort consuming task, calling for an autocomplete solution to assist users. We present ChaI-TeA: Chat Interaction
-
2025The training and fine-tuning of large language models (LLMs) often involve diverse textual data from multiple sources, which poses challenges due to conflicting gradient directions, hindering optimization and specialization. These challenges can undermine model generalization across tasks, resulting in reduced downstream performance. Recent research suggests that fine-tuning LLMs on carefully selected,
Academia
View allWhether you're a faculty member or student, there are number of ways you can engage with Amazon.
View all