In 2019, the U.S. National Science Foundation (NSF) and Amazon announced a collaboration — the Fairness in AI program — to strengthen and support fairness in artificial intelligence and machine learning.
To date, in two rounds of proposal submissions, NSF has awarded 21 research grants in areas such as ensuring fairness in AI algorithms and the systems that incorporate them, using AI to promote equity in society, and developing principles for human interaction with AI-based systems.
In June of 2021, Amazon and the NSF opened the third round of submissions with a focus on theoretical and algorithmic foundations; principles for human interaction with AI systems; technologies such as natural language understanding and computer vision; and applications including hiring decisions, education, criminal justice, and human services.
Now Amazon and NSF are announcing the recipients of 13 selected projects from that latest call for submissions.
The awardees, who collectively will receive up to $9.5 million in financial support, have proposed projects that address unfairness and bias in artificial intelligence and machine learning technologies, develop principles for human interaction with artificial intelligence systems, and theoretical frameworks for algorithms, and improve accessibility of speech recognition technology.
“We are thrilled to share NSF’s selection of thirteen Fairness in AI proposals from talented researchers across the United States,” said Prem Natarajan, Alexa AI vice president of Natural Understanding. “The increasing prevalence of AI in our everyday lives calls for continued multi-sector investments into advancing their trustworthiness and robustness against bias. Amazon is proud to have partnered with the NSF for the past three years to support this critically important research area.”
Amazon, which provides partial funding for the program, does not participate in the grant-selection process.
“These awards are part of NSF's commitment to pursue scientific discoveries that enable us to achieve the full spectrum of artificial intelligence potential at the same time we address critical questions about their uses and impacts," said Wendy Nilsen, deputy division director for NSF's Information and Intelligent Systems Division.
More information about the Fairness in AI program is available on NSF website, and via their program update. Below is the list of the 2022 awardees, and an overview of their projects.
- An interpretable AI framework for care of critically ill patients involving matching and decision trees
“This project introduces a framework for interpretable, patient-centered causal inference and policy design for in-hospital patient care. This framework arose from a challenging problem, which is how to treat critically ill patients who are at risk for seizures (subclinical seizures) that can severely damage a patient's brain. In this high-stakes application of artificial intelligence, the data are complex, including noisy time-series, medical history, and demographic information. The goal is to produce interpretable causal estimates and policy decisions, allowing doctors to understand exactly how data were combined, permitting better troubleshooting, uncertainty quantification, and ultimately, trust. The core of the project's framework consists of novel and sophisticated matching techniques, which match each treated patient in the dataset with other (similar) patients who were not treated. Matching emulates a randomized controlled trial, allowing the effect of the treatment to be estimated for each patient, based on the outcomes from their matched group. A second important element of the framework involves interpretable policy design, where sparse decision trees will be used to identify interpretable subgroups of individuals who should receive similar treatments.”
- Principal investigator: Cynthia Rudin
- Co-principal investigators: Alexander Volfovsky, Sudeepa Roy
- Organization: Duke University
- Award amount: $625,000
- Fair representation learning: fundamental trade-offs and algorithms
“Artificial intelligence-based computer systems are increasingly reliant on effective information representation in order to support decision making in domains ranging from image recognition systems to identity control through face recognition. However, systems that rely on traditional statistics and prediction from historical or human-curated data also naturally inherit any past biased or discriminative tendencies. The overarching goal of the award is to mitigate this problem by using information representations that maintain its utility while eliminating information that could lead to discrimination against subgroups in a population. Specifically, this project will study the different trade-offs between utility and fairness of different data representations, and then identify solutions to reduce the gap to the best trade-off. Then, new representations and corresponding algorithms will be developed guided by such trade-off analysis. The investigators will provide performance limits based on the developed theory, and also evidence of efficacy in order to obtain fair machine learning systems and to gain societal trust. The application domain used in this research is face recognition systems. The undergraduate and graduate students who participate in the project will be trained to conduct cutting-edge research to integrate fairness into artificial intelligent based systems.”
- Principal investigator: Vishnu Boddeti
- Organization: Michigan State University
- Award amount: $331,698
- A new paradigm for the evaluation and training of inclusive automatic speech recognition
“Automatic speech recognition can improve your productivity in small ways: rather than searching for a song, a product, or an address using a graphical user interface, it is often faster to accomplish these tasks using automatic speech recognition. For many groups of people, however, speech recognition works less well, possibly because of regional accents, or because of second-language accent, or because of a disability. This Fairness in AI project defines a new way of thinking about speech technology. In this new way of thinking, an automatic speech recognizer is not considered to work well unless it works well for all users, including users with regional accents, second-language accents, and severe disabilities. There are three sub-projects. The first sub-project will create black-box testing standards that speech technology researchers can use to test their speech recognizers, in order to test how useful their speech recognizer will be for different groups of people. For example, if a researcher discovers that their product works well for some people, but not others, then the researcher will have the opportunity to gather more training data, and to perform more development, in order to make sure that the under-served community is better-served. The second sub-project will create glass-box testing standards that researchers can use to debug inclusivity problems. For example, if a speech recognizer has trouble with a particular dialect, then glass-box methods will identify particular speech sounds in that dialect that are confusing the recognizer, so that researchers can more effectively solve the problem. The third sub-project will create new methods for training a speech recognizer in order to guarantee that it works equally well for all of the different groups represented in available data. Data will come from podcasts and the Internet. Speakers will be identified as members of a particular group if and only if they declare themselves to be members of that group. All of the developed software will be distributed open-source.”
- Principal investigator: Mark Hasegawa-Johnson
- Co-principal investigators: Zsuzsanna Fagyal, Najim Dehak, Piotr Zelasko, Laureano Moro-Velazquez
- Organization: University of Illinois at Urbana-Champaign
- Award amount: $500,000
- A normative economic approach to fairness in AI
“A vast body of work in algorithmic fairness is devoted to preventing artificial intelligence (AI) from exacerbating societal biases. The predominant viewpoints in this literature equates fairness with lack of bias or seeks to achieve some form of statistical parity between demographic groups. By contrast, this project pursues alternative approaches rooted in normative economics, the field that evaluates policies and programs by asking "what should be". The work is driven by two observations. First, fairness to individuals and groups can be realized according to people’s preferences represented in the form of utility functions. Second, traditional notions of algorithmic fairness may be at odds with welfare (the overall utility of groups), including the welfare of those groups the fairness criteria intend to protect. The goal of this project is to establish normative economic approaches as a central tool in the study of fairness in AI. Towards this end the team pursues two research questions. First, can the perspective of normative economics be reconciled with existing approaches to fairness in AI? Second, how can normative economics be drawn upon to rethink what fairness in AI should be? The project will integrate theoretical and algorithmic advances into real systems used to inform refugee resettlement decisions. The system will be examined from a fairness viewpoint, with the goal of ultimately ensuring fairness guarantees and welfare.”
- Principal investigator: Yiling Chen
- Co-principal investigator: Ariel Procaccia
- Organization: Harvard University
- Award amount: $560,345
- Advancing optimization for threshold-agnostic fair AI systems
“Artificial intelligence (AI) and machine learning technologies are being used in high-stakes decision-making systems like lending decision, employment screening, and criminal justice sentencing. A new challenge arising with these AI systems is avoiding the unfairness they might introduce and that can lead to discriminatory decisions for protected classes. Most AI systems use some kinds of thresholds to make decisions. This project aims to improve fairness-aware AI technologies by formulating threshold-agnostic metrics for decision making. In particular, the research team will improve the training procedures of fairness-constrained AI models to make the model adaptive to different contexts, applicable to different applications, and subject to emerging fairness constraints. The success of this project will yield a transferable approach to improve fairness in various aspects of society by eliminating the disparate impacts and enhancing the fairness of AI systems in the hands of the decision makers. Together with AI practitioners, the researchers will integrate the techniques in this project into real-world systems such as education analytics. This project will also contribute to training future professionals in AI and machine learning and broaden this activity by including training high school students and under-represented undergraduates.”
- Principal investigator: Tianbao Yang
- Co-principal investigators: Qihang Lin, Mingxuan Sun
- Organization: University of Iowa
- Award amount: $500,000
- Toward fair decision making and resource allocation with application to AI-assisted graduate admission and degree completion
“Machine learning systems have become prominent in many applications in everyday life, such as healthcare, finance, hiring, and education. These systems are intended to improve upon human decision-making by finding patterns in massive amounts of data, beyond what can be intuited by humans. However, it has been demonstrated that these systems learn and propagate similar biases present in human decision-making. This project aims to develop general theory and techniques on fairness in AI, with applications to improving retention and graduation rates of under-represented groups in STEM graduate programs. Recent research has shown that simply focusing on admission rates is not sufficient to improve graduation rates. This project is envisioned to go beyond designing "fair classifiers" such as fair graduate admission that satisfy a static fairness notion in a single moment in time, and designs AI systems that make decisions over a period of time with the goal of ensuring overall long-term fair outcomes at the completion of a process. The use of data-driven AI solutions can allow the detection of patterns missed by humans, to empower targeted intervention and fair resource allocation over the course of an extended period of time. The research from this project will contribute to reducing bias in the admissions process and improving completion rates in graduate programs as well as fair decision-making in general applications of machine learning.”
- Principal investigator: Furong Huang
- Co-principal investigators: Min Wu, Dana Dachman-Soled
- Organization: University of Maryland, College Park
- Award amount: $625,000
- BRMI — bias reduction in medical information
“This award, Bias Reduction In Medical Information (BRIMI), focuses on using artificial intelligence (AI) to detect and mitigate biased, harmful, and/or false health information that disproportionately hurts minority groups in society. BRIMI offers outsized promise for increased equity in health information, improving fairness in AI, medicine, and in the information ecosystem online (e.g., health websites and social media content). BRIMI's novel study of biases stands to greatly advance the understanding of the challenges that minority groups and individuals face when seeking health information. By including specific interventions for both patients and doctors and advancing the state-of-the-art in public health and fact checking organizations, BRIMI aims to inform public policy, increase the public's critical literacy, and improve the well-being of historically under-served patients. The award includes significant outreach efforts, which will engage minority communities directly in our scientific process; broad stakeholder engagement will ensure that the research approach to the groups studied is respectful, ethical, and patient-centered. The BRIMI team is composed of academics, non-profits, and industry partners, thus improving collaboration and partnerships across different sectors and multiple disciplines. The BRIMI project will lead to fundamental research advances in computer science, while integrating deep expertise in medical training, public health interventions, and fact checking. BRIMI is the first large scale computational study of biased health information of any kind. This award specifically focuses on bias reduction in the health domain; its foundational computer science advances and contributions may generalize to other domains, and it will likely pave the way for studying bias in other areas such as politics and finances.”
- Principal investigator: Shiri Dori-Hacohen
- Co-principal investigators: Sherry Pagoto, Scott Hale
- Organization: University of Connecticut
- Award amount: $392,994
- A novel paradigm for fairness-aware deep learning models on data streams
“Massive amounts of information are transferred constantly between different domains in the form of data streams. Social networks, blogs, online businesses, and sensors all generate immense data streams. Such data streams are received in patterns that change over time. While this data can be assigned to specific categories, objects and events, their distribution is not constant. These categories are subject to distribution shifts. These distribution shifts are often due to the changes in the underlying environmental, geographical, economic, and cultural contexts. For example, the risks levels in loan applications have been subject to distribution shifts during the COVID-19 pandemic. This is because loan risks are based on factors associated to the applicants, such as employment status and income. Such factors are usually relatively stable, but have changed rapidly due to the economic impact of the pandemic. As a result, existing loan recommendation systems need to be adapted to limited examples. This project will develop open software to help users evaluate online fairness-in algorithms, mitigate potential biases, and examine utility-fairness trade-offs. It will implement two real-world applications: online crime event recognition from video data and online purchase behavior prediction from click-stream data. To amplify the impact of this project in research and education, this project will leverage STEM programs for students with diverse backgrounds, gender and race/ethnicity. This project includes activities including seminars, workshops, short courses, and research projects for students.”
- Principal investigator: Feng Chen
- Co-principal investigators: Latifur Khan, Xintao Wu, Christan Grant
- Organization: University of Texas at Dallas
- Award amount: $392,993
- A human-centered approach to developing accessible and reliable machine translation
“This Fairness in AI project aims to develop technology to reliably enhance cross-lingual communication in high-stakes contexts, such as when a person needs to communicate with someone who does not speak their language to get health care advice or apply for a job. While machine translation technology is frequently used in these conditions, existing systems often make errors that can have severe consequences for a patient or a job applicant. Further, it is challenging for people to know when automatic translations might be wrong when they do not understand the source or target language for translation. This project addresses this issue by developing accessible and reliable machine translation for lay users. It will provide mechanisms to guide users to recognize and recover from translation errors, and help them make better decisions given imperfect translations. As a result, more people will be able to use machine translation reliably to communicate across language barriers, which can have far-reaching positive consequences on their lives."
- Principal investigator: Marine Carpuat
- Co-principal investigators: Niloufar Salehi, Ge Gao
- Organization: University of Maryland, College Park
- Award amount: $392,993
- AI algorithms for fair auctions, pricing, and marketing
“This project develops algorithms for making fair decisions in AI-mediated auctions, pricing, and marketing, thus advancing national prosperity and economic welfare. The deployment of AI systems in business settings has thrived due to direct access to consumer data, the capability to implement personalization, and the ability to run algorithms in real-time. For example, advertisements users see are personalized since advertisers are willing to bid more in ad display auctions to reach users with particular demographic features. Pricing decisions on ride-sharing platforms or interest rates on loans are customized to the consumer's characteristics in order to maximize profit. Marketing campaigns on social media platforms target users based on the ability to predict who they will be able to influence in their social network. Unfortunately, these applications exhibit discrimination. Discriminatory targeting in housing and job ad auctions, discriminatory pricing for loans and ride-hailing services, and disparate treatment of social network users by marketing campaigns to exclude certain protected groups have been exposed. This project will develop theoretical frameworks and AI algorithms that ensure consumers from protected groups are not harmfully discriminated against in these settings. The new algorithms will facilitate fair conduct of business in these applications. The project also supports conferences that bring together practitioners, policymakers, and academics to discuss the integration of fair AI algorithms into law and practice.”
- Principal investigator: Adam Elmachtoub
- Co-principal investigators: Shipra Agrawal, Rachel Cummings, Christian Kroer, Eric Balkanski
- Organization: Columbia University
- Award amount: $392,993
- Using explainable AI to increase equity and transparency in the juvenile justice system’s use of risk scores
“Throughout the United States, juvenile justice systems use juvenile risk and need-assessment (JRNA) scores to identify the likelihood a youth will commit another offense in the future. This risk assessment score is then used by juvenile justice practitioners to inform how to intervene with a youth to prevent reoffending (e.g., referring youth to a community-based program vs. placing a youth in a juvenile correctional center). Unfortunately, most risk assessment systems lack transparency and often the reasons why a youth received a particular score are unclear. Moreover, how these scores are used in the decision making process is sometimes not well understood by families and youth affected by such decisions. This possibility is problematic because it can hinder individuals’ buy-in to the intervention recommended by the risk assessment as well as mask potential bias in those scores (e.g., if youth of a particular race or gender have risk scores driven by a particular item on the assessment). To address this issue, project researchers will develop automated, computer-generated explanations for these risk scores aimed at explaining how these scores were produced. Investigators will then test whether these better-explained risk scores help youth and juvenile justice decision makers understand the risk score a youth is given. In addition, the team of researchers will investigate whether these risk scores are working equally well for different groups of youth (for example, equally well for boys and for girls) and identify any potential biases in how they are being used in an effort to understand how equitable the decision making process is for demographic groups based on race and gender. The project is embedded within the juvenile justice system and aims to evaluate how real stakeholders understand how the risk scores are generated and used within that system based on actual juvenile justice system data.”
- Principal investigator: Trent Buskirk
- Co-principal investigators: Kelly Murphy
- Organization: Bowling Green State University
- Award amount: $392,993
- Breaking the tradeoff barrier in algorithmic fairness
“In order to be robust and trustworthy, algorithmic systems need to usefully serve diverse populations of users. Standard machine learning methods can easily fail in this regard, e.g. by optimizing for majority populations represented within their training data at the expense of worse performance on minority populations. A large literature on "algorithmic fairness" has arisen to address this widespread problem. However, at a technical level, this literature has viewed various technical notions of "fairness" as constraints, and has therefore viewed "fair learning" through the lens of constrained optimization. Although this has been a productive viewpoint from the perspective of algorithm design, it has led to tradeoffs being centered as the central object of study in "fair machine learning". In the standard framing, adding new protected populations, or quantitatively strengthening fairness constraints, necessarily leads to decreased accuracy overall and within each group. This has the effect of pitting the interests of different stakeholders against one another, and making it difficult to build consensus around "fair machine learning" techniques. The over-arching goal of this project is to break through this "fairness/accuracy tradeoff" paradigm.”
- Principal investigator: Aaron Roth
- Co-principal investigator: Michael Kearns
- Organization: University of Pennsylvania
- Award amount: $392,992
- Advancing deep learning towards spatial fairness
“The goal of spatial fairness is to reduce biases that have significant linkage to the locations or geographical areas of data samples. Such biases, if left unattended, can cause or exacerbate unfair distribution of resources, social division, spatial disparity, and weaknesses in resilience or sustainability. Spatial fairness is urgently needed for the use of artificial intelligence in a large variety of real-world problems such as agricultural monitoring and disaster management. Agricultural products, including crop maps and acreage estimates, are used to inform important decisions such as the distribution of subsidies and providing farm insurance. Inaccuracies and inequities produced by spatial biases adversely affect these decisions. Similarly, effective and fair mapping of natural disasters such as floods or fires is critical to inform live-saving actions and quantify damages and risks to public infrastructures, which is related to insurance estimation. Machine learning, in particular deep learning, has been widely adopted for spatial datasets with promising results. However, straightforward applications of machine learning have found limited success in preserving spatial fairness due to the variation of data distribution, data quantity, and data quality. The goal of this project is to develop a new generation of learning frameworks to explicitly preserve spatial fairness. The results and code will be made freely available and integrated into existing geospatial software. The methods will also be tested for incorporation in existing real systems (crop and water monitoring).”
- Principal investigator: Xiaowei Jia
- Co-principal investigators: Sergii Skakun, Yiqun Xie
- Organization: University of Pittsburgh
- Award amount: $755,098
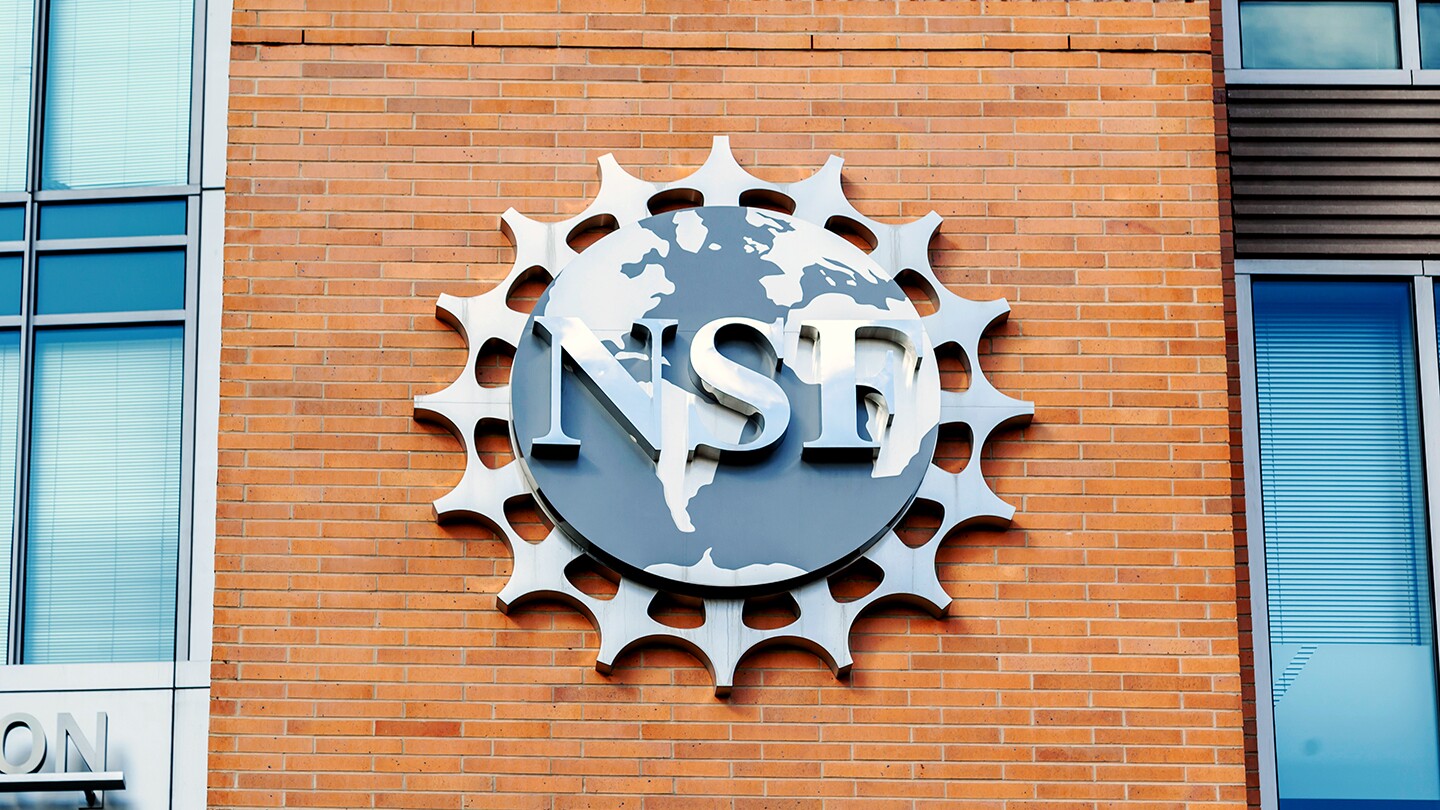
JHVEPhoto — stock.adobe.com
U.S. National Science Foundation, in collaboration with Amazon, announces latest Fairness in AI grant projects
Thirteen new projects focus on ensuring fairness in AI algorithms and the systems that incorporate them.
Research areas