PLAN-Bot makes adaptable conversation a reality by allowing the user to follow personalized decisions through the completion of multiple sequential subtasks. We aim to develop a taskbot that helps customers by addressing their changing needs as they complete complex tasks
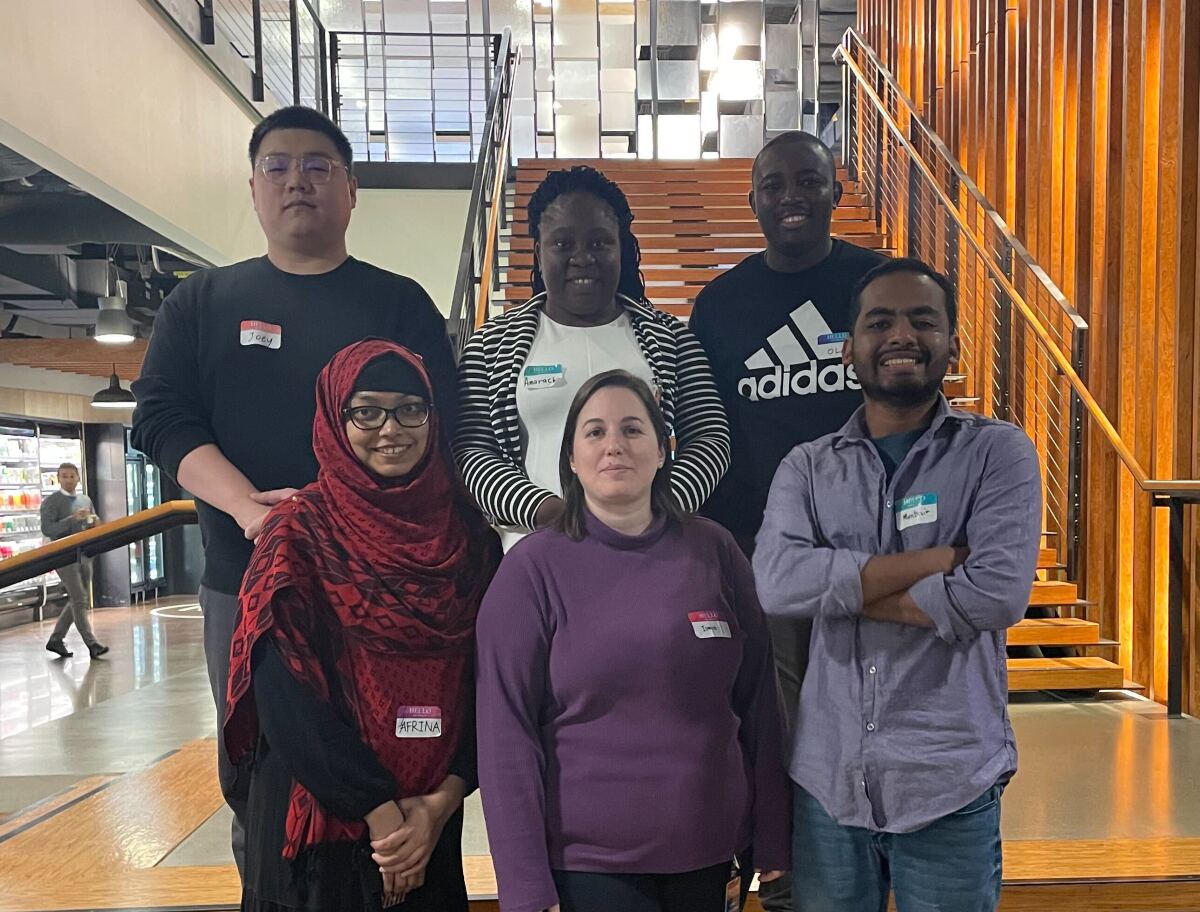
Afrina Tabassum - Team leader
Tabassum's research interests are in the area of Machine Learning, particularly designing semantic representation and generation methods for multimodal data. She is a member of the PLAN Lab and her projects include self-supervised learning and video understanding such as (1) utilizing information from multiple modalities (video, audio, text) to learn semantically-grounded multi-modal data representations, (2) improving the scalability of constrastive learning by selecting diverse hard negative examples, (3) designing permutation-equivariant concept-level representations for zero-shot video generation conditioned on text/image, (4) synthesizing zero-shot talking head videos conditioned on text, and (5) improving the robustness of constrastive training to adversarial attacks.
Muntasir Wahed
Wahed is pursuing his Ph.D. in Computer Science at Virginia Tech. Broadly He is interested in robust feature representations in machine learning. More specifically, he is working on contrastive learning, mixture-of-experts, and information retrieval. He has an excellent understanding of statistical concepts and machine learning, and a broad experience with programming languages and frameworks, especially Python and C. Additionally, he has experience working with Android and Full Stack Web Development.
Tianjiao Yu
Yu is currently a 2nd-year Phd student at Virginia Tech, working in both PLAN lab and Crowd Lab. His research mainly focuses on NLP. He is experienced in theoretical linguistics, especially in theoretical syntax and semantics. In addition to linguistics, his research also leverages crowd-sourcing concepts and frameworks to build more robust and efficient ML models. His current research focuses on diffusing semantics and affordance in multimodal settings.
Amarachi Blessing Mbakwe
Mbakwe is a 3rd-year Ph.D. student in the Department of Computer Science at Virginia Tech and a member of the PLAN lab. Her expertise lies in Scene Understanding, Human-Computer Interaction (HCI), and Natural Language Processing (NLP). Her previous work introduced an anatomy-aware model for tracking longitudinal relationships between images. Her research aims at proposing robust and explainable commonsense reasoning algorithms for multimodal data.
Makanjuola Ogunleye
Ogunleye is a 3rd-year Computer Science Ph.D. student and a member of the PLAN Lab at Virginia Tech, where he studies Artificial Intelligent agents’ behavior in cooperative and collaborative environments. Especially how agents can exploit communication and collaboration in order to perform a task effectively. This line of research has applications in building seamless Intelligent Task Assistants and Natural Language Understanding. He has a general interest in Natural Language Processing, Embodied AI, and Multi-Modal Machine Learning. In this challenge, he will work on the Hybrid Response Generator and Object State Tracking & Reasoning.
Ismini Lourentzou - Faculty advisor
Lourentzou leads the Perception and LANguage (PLAN) Lab at the Virginia Tech Department of Computer Science and is also core faculty at the Sanghani Center for Artificial Intelligence and Discovery Analytics. Her research interests include multimodal machine learning (vision + language) and learning with limited supervision, with applications to healthcare, embodied AI, language grounding, etc.