Amazon recently announced the launch of AutoGluon, a new open-source library for developers building applications involving machine learning with image, text, or tabular data sets. With AutoGluon, developers can harness the power of deep learning for building applications by writing just a few lines of code.
“We developed AutoGluon to truly democratize machine learning, and make the power of deep learning available to all developers,” says Jonas Mueller, AWS applied scientist.
Advancing the state of the art in deep learning
Deep learning, a subset of machine learning, is loosely inspired by the structure of the human brain. Deep learning algorithms typically comprise multiple layers that are used to learn useful representations of input data. For example, in a deep learning model for image recognition, lower layers could be used to detect more basic features like colors or edges, while higher layers can be used to identify more complex features like digits or objects.
Traditionally, deploying deep learning models to achieve state-of-the-art performance has required extensive expertise. Even though the use of deep learning today is still primarily limited to a finite number of experts, there have been advances in simplifying deep learning and making it more accessible to technical professionals over the last decade.
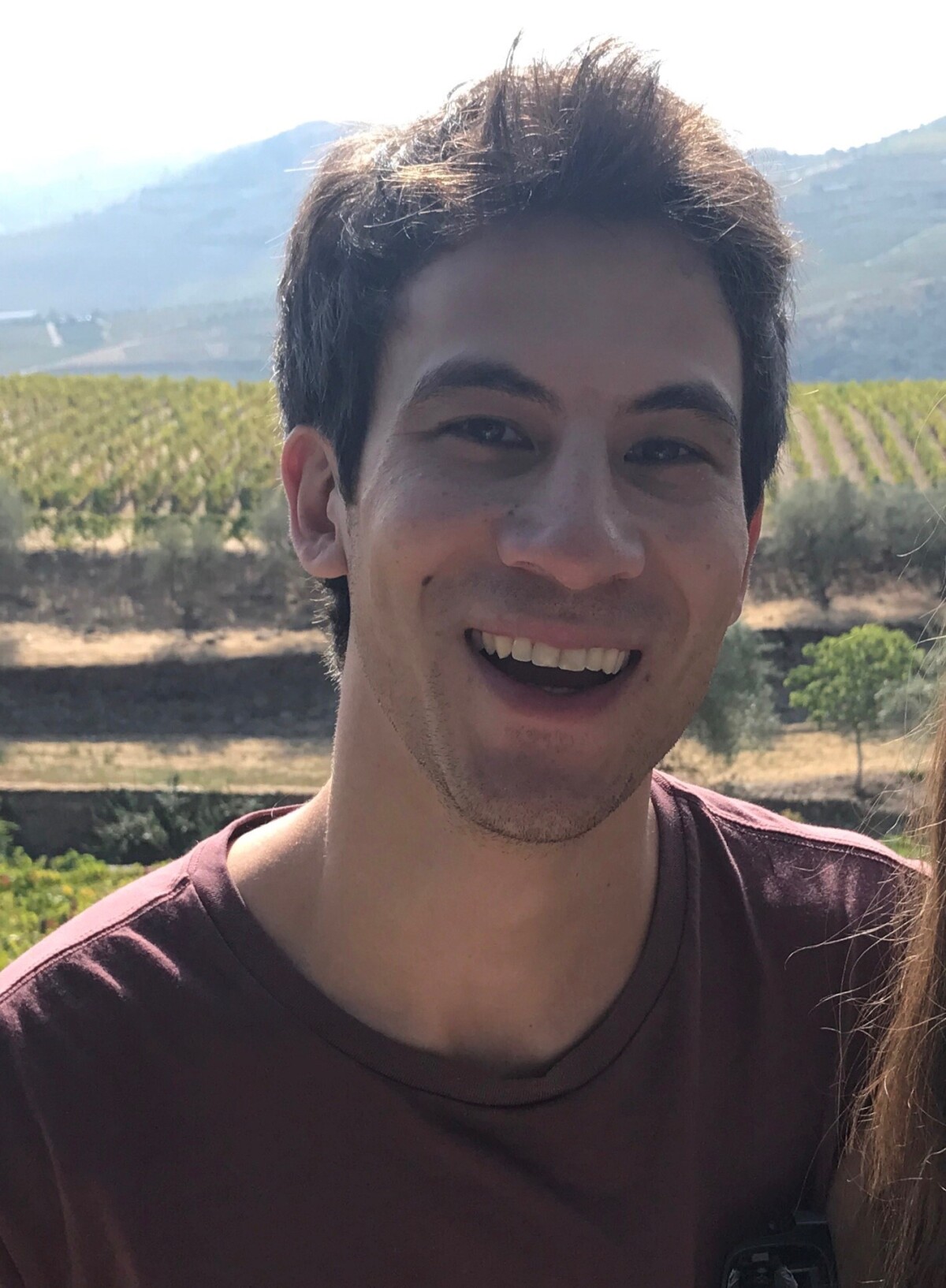
For example, developers had to initially invest a considerable amount of time and expertise to calculate gradients necessary for training their deep learning models. Gradients are vectors that demonstrate the most efficient parameter updates to minimize errors on the examples in the training data. Software libraries like Theano automated the calculation of gradients of even highly complex neural networks, and allowed developers to leverage increasingly sophisticated neural architectures via boilerplate code.
More recent libraries like Keras represented another shift forward in democratizing deep learning. Keras, which is an abstraction of TensorFlow, allowed developers to specify parameters like the number of inputs, the number of layers in a deep learning model, and the definition of individual network layers with a few lines of code, removing much of the boilerplate code that was necessary in the existing libraries at the time.
However, even with these advancements, deep-learning experts and developers today must still grapple with many cumbersome issues, including hyperparameter tuning, data pre-processing, neural-architecture search, and decisions related to leveraging transfer learning.
Hyperparameters represent the considerable number of choices developers must make in constructing a neural network. These involve selections like the number of layers in the neural network, how these layers should be connected (i.e., the architecture of the network), and how the network should be trained. Data pre-processing, which involves classifying the data and formatting vectors correctly, can be a highly cumbersome process, as well. Neural-architecture search involves automating architecture engineering, which enables developers to find the best design for their machine learning models. All of these decisions require considerable expertise and present obstacles in making deep learning more accessible.
We developed AutoGluon to truly democratize machine learning, and make the power of deep learning available to all developers.
AutoGluon automates many of these decisions for developers so that they can produce a high-performance neural-network model with as few as three lines of code. There’s no need for developers to manually experiment with the hundreds of individual choices that must be made while designing a deep learning model. Rather, they can simply specify when they would like to have their trained model ready. In response, AutoGluon leverages the available compute resources to find the strongest model within its allotted run-time.
“Due to the inherently opaque nature of deep learning, many of the choices made by deep-learning experts are based on ad hoc intuition, rather than a rigorous scientific understanding of how individual choices affect desired outcomes,” says Mueller. “AutoGluon solves this problem, as all choices are automatically tuned within default ranges that are known to perform well for the particular task and model.”
Getting started with AutoGluon
The AutoGluon website features numerous tutorials for developers to leverage deep learning for tabular, text, and image data (covering both basic tasks like classification/regression as well as more advanced tasks like object detection). For more-experienced developers, the AutoGluon website features customization instructions on how to use AutoGluon APIs for automatically improving predictive performance in customized applications.
The release of AutoGluon is especially meaningful to Mueller, who joined Amazon after completing his PhD from MIT.
“I wanted to join a company that was truly committed to making the power of machine learning available to all. At Amazon, I’ve had the opportunity to work with incredibly talented colleagues and drive projects like AutoGluon to completion.”
Bookmark the AutoGluon website and the AutoGluon GitHub repository to learn more and stay updated on new releases.