This year’s Interspeech — the largest conference in speech technology — will take place in Hyderabad, India, the first week of September. More than 40 Amazon researchers will be attending, including Björn Hoffmeister, the senior manager for machine learning in the Alexa Automatic Speech Recognition group. He took a few minutes to answer three questions about this year’s conference.
What do you look forward to about Interspeech?
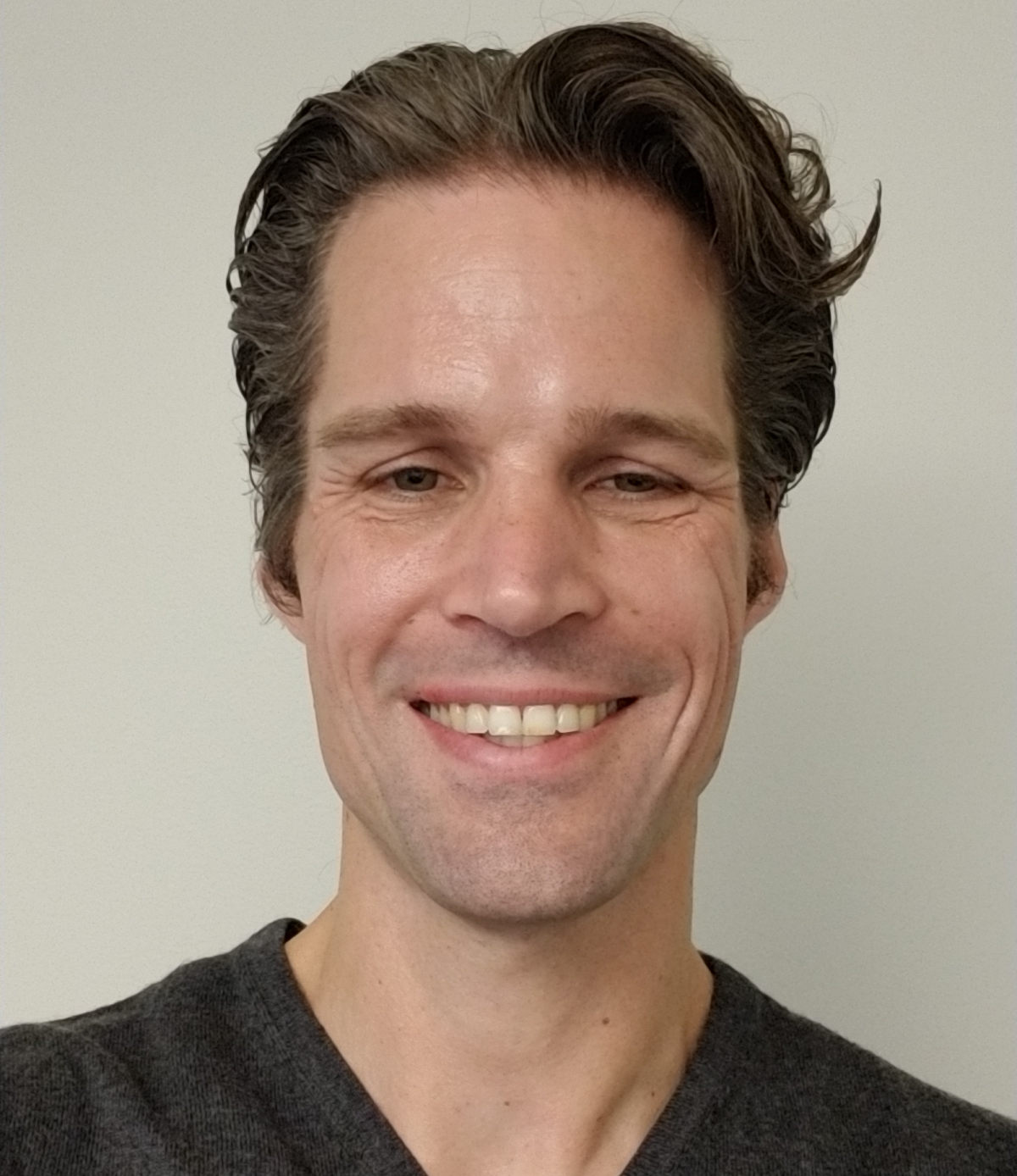
Interspeech is the biggest conference in speech technology. There is a speech community, and I’m excited to go there and see where the big ship of speech recognition is heading next.
Another thing is that I get to find a lot of small and large ideas that we can bring into our systems. It might be a small tweak that makes something run more efficiently, or it could be a new idea that kicks off a large research project.
The week of the conference also takes you out of your immediate concerns. For these few days, you’re immersed in this mode of thinking big, and this triggers a lot of very fruitful discussions centered on research. Even with other members of your own team you tend to have more research-focused conversations. And of course with colleagues at other institutions, too.
Finally, it’s a great venue for meeting the next generation of PhD students in our field. We build up relationships there, sometimes over years, which can help us attract talent. It’s the biggest conference in our field, but it’s also the biggest networking event for people working in speech technologies. I always look forward to reconnecting with the community there, and establishing new connections as well.
Does the fact that the conference is in India have a particular appeal?
For me, personally, it’s attractive because I can combine the conference with a visit to our Bangalore office. The focus of the scientists there is very well aligned with the theme of this year’s Interspeech, which is “speech research for emerging markets in multilingual societies.” There are so many languages in India, and we want to be flexible, so that people can talk to Alexa in more than one of them.
There is a combination of challenges when research moves from a language that is highly resourced, like English, to languages and dialects that don’t have huge corpora of annotated training data but still need to work together with similar accuracy.
And then there is the engineering question of how to scale the system to support several languages at once. The Bangalore team is actively investigating that.
Looking over the list of papers accepted to Interspeech, what broad trends do you see in the field?
We see that sequence-to-sequence modeling for automatic speech recognition — the idea of being all neural — that this continues. Sequence-to-sequence means to go directly from features derived from the audio signal all the way to producing the final text output, and doing that using an encoder-decoder approach, where the encoder first ingests the audio signal and then the decoder produces the text. The broad trend is making it all neural, making it all a single architecture that can be optimized jointly.
The other big trend is tackling the crosstalk problem, where multiple people are talking at the same time. It’s a very hard problem. There have always been attempts to tackle it. So far, the attempts have been working in the lab environment but only to a limited degree in production systems.
One promising approach right now is the deep-clustering approach, and deep clustering goes together with another trend that we see: instead of using a single microphone, using multiple microphones feeding directly into the big neural model. Deep clustering is already showing very promising results for one microphone, but with a microphone array, it seems to work even better. If you put those technologies together, we’re pretty optimistic that we can get the crosstalk problem under control.
The interesting thing is, since we do everything in the cloud, it’s possible to later deploy these improvements to any of our devices. They already have multiple microphones; the rest is just software.
Using contextual information is one of the other big stories. The more a device like Echo can do, the more we expect to see multiturn interactions, and in any multiturn interaction, all the previous turns provide a lot of context that we want to consider to help us to improve accuracy.
The other way to use context is that we know something about the speaker: we know the preferences of the speaker; we know the past topics the speaker was talking about. So we can use all that information as contextual clues to improve speech recognition in the first place. We are investigating that, and I saw other papers at Interspeech that are also looking into that topic.