Of Amazon’s more than 40 papers at this year’s Interspeech, automatic speech recognition and text-to-speech account for about half. But the others cover a range of topics, from acoustic watermarking and automatic dubbing to quantization and fairness.
Acoustic watermarking
Practical over-the-air perceptual acoustic watermarking
Ameya Agaskar
Audio classification
CNN-based audio event recognition for automated violence classification and rating for Prime Video content
Tarun Gupta, Mayank Sharma, Kenny Qiu, Xiang Hao, Raffay Hamid
Impact of acoustic event tagging on scene classification in a multi-task learning framework
Rahil Parikh, Harshavardhan Sundar, Ming Sun, Chao Wang, Spyros Matsoukas
Automatic dubbing
Isochrony-aware neural machine translation for automatic dubbing
Derek Tam, Surafel Melaku Lakew, Yogesh Virkar, Prashant Mathur, Marcello Federico
Prosodic alignment for off-screen automatic dubbing
Yogesh Virkar, Marcello Federico, Robert Enyedi, Roberto Barra-Chicote
Automatic speech recognition
Compute cost amortized transformer for streaming ASR
Yi Xie, Jonathan Macoskey, Martin Radfar, Feng-Ju Chang, Brian King, Ariya Rastrow, Athanasios Mouchtaris, Grant Strimel
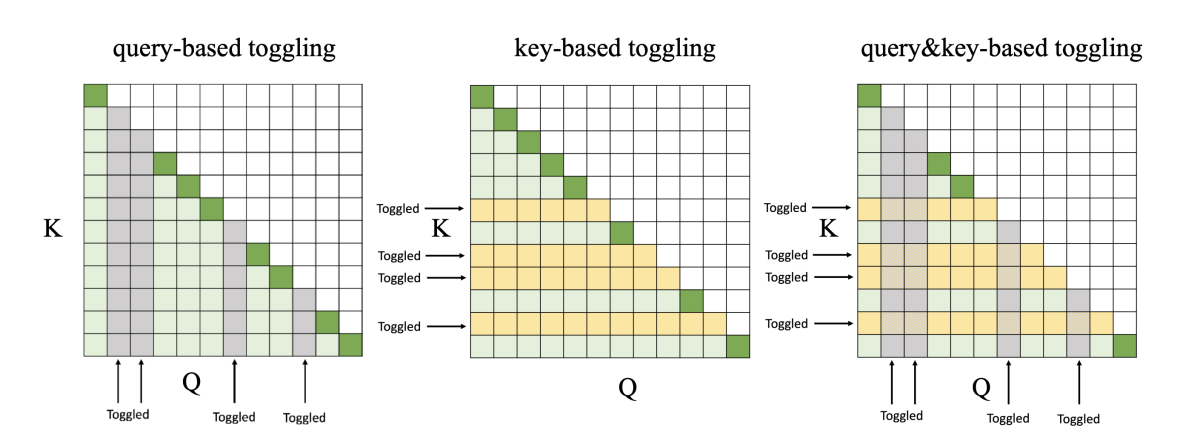
Content-context factorized representations for automated speech recognition
David M. Chan, Shalini Ghosh
ConvRNN-T: Convolutional augmented recurrent neural network transducers for streaming speech recognition
Martin Radfar, Rohit Barnwal, Rupak Vignesh Swaminathan, Feng-Ju Chang, Grant Strimel, Nathan Susanj, Athanasios Mouchtaris
Directed speech separation for automatic speech recognition of long-form conversational speech
Rohit Paturi, Sundararajan Srinivasan, Katrin Kirchhoff, Daniel Garcia-Romero
Domain prompts: Towards memory and compute efficient domain adaptation of ASR systems
Saket Dingliwa, Ashish Shenoy, Sravan Bodapati, Ankur Gandhe, Ravi Teja Gadde, Katrin Kirchhoff
Incremental learning for RNN-Transducer based speech recognition models
Deepak Baby, Pasquale D'Alterio, Valentin Mendelev
Knowledge distillation via module replacing for automatic speech recognition with recurrent neural network transducer
Kaiqi Zhao, Hieu Duy Nguyen, Animesh Jain, Nathan Susanj, Athanasios Mouchtaris, Lokesh Gupta, Ming Zhao
Learning to rank with BERT-based confidence models in ASR rescoring
Ting-Wei Wu, I-FAN CHEN, Ankur Gandhe
Reducing geographic disparities in automatic speech recognition via elastic weight consolidation
Viet Anh Trinh, Pegah Ghahremani, Brian King, Jasha Droppo, Andreas Stolcke, Roland Maas
RefTextLAS: Reference text biased listen, attend, and spell model for accurate reading evaluation
Phani Sankar Nidadavolu, Na Xu, Nick Jutila, Ravi Teja Gadde, Aswarth Abhilash Dara, Joseph Savold, Sapan Patel, Aaron Hoff, Veerdhawal Pande, Kevin Crews, Ankur Gandhe, Ariya Rastrow, Roland Maas
RNN-T lattice enhancement by grafting of pruned paths
Mirek Novak, Pavlos Papadopoulos
Using data augmentation and consistency regularization to improve semi-supervised speech recognition
Ashtosh Sapru
Dialogue
Contextual acoustic barge in classification for spoken dialog systems
Dhanush Bekal, Sundararajan Srinivasan, Sravan Bodapati, Srikanth Ronanki, Katrin Kirchhoff
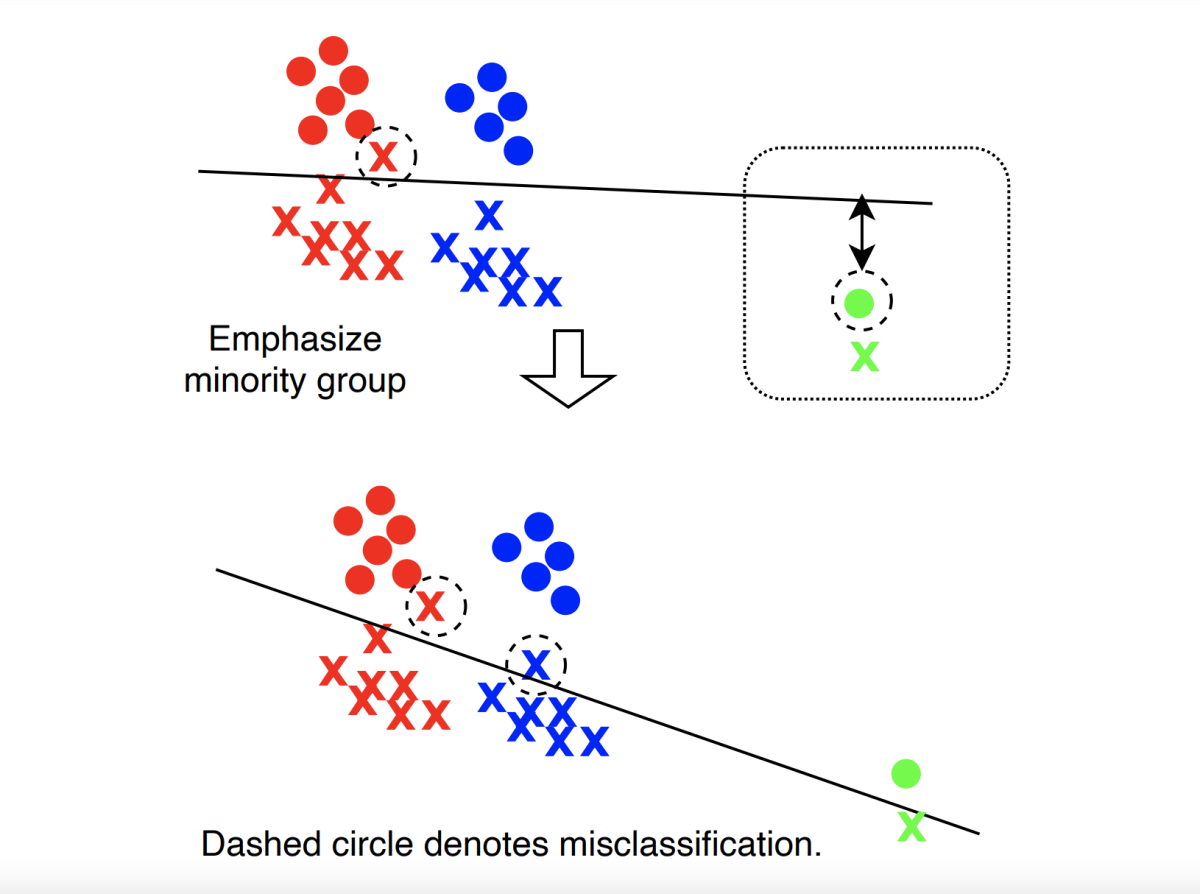
Fairness
Toward fairness in speech recognition: Discovery and mitigation of performance disparities
Pranav Dheram, Murugesan Ramakrishnan, Anirudh Raju, I-Fan Chen, Brian King, Katherine Powell, Melissa Saboowala, Karan Shetty, Andreas Stolcke
Keyword spotting
Latency control for keyword spotting
Christin Jose, Joe Wang, Grant Strimel, Mohammad Omar Khursheed, Yuriy Mishchenko, Brian Kulis
Language identification
A multimodal strategy for singing language identification
Wo Jae Lee, Emanuele Coviello
Multidevice processing
Challenges and opportunities in multi-device speech processing
Gregory Ciccarelli, Jarred Barber, Arun Nair, Israel Cohen, Tao Zhang
Multiparty speech
Separator-transducer-segmenter: Streaming recognition and segmentation of multi-party speech
Ilya Sklyar, Anna Piunova, Christian Osendorfer
Natural-language understanding
Phonetic embedding for ASR robustness in entity resolution
Xiaozhou Zhou, Ruying Bao, William M. Campbell
Quantization
Squashed weight distribution for low bit quantization of deep models
Nikko Ström, Haidar Khan, Wael Hamza
Sub-8-bit quantization aware training for 8-bit neural network accelerator with on device speech recognition
Kai Zhen, Hieu Duy Nguyen, Raviteja Chinta, Nathan Susanj, Athanasios Mouchtaris, Tariq Afzal, Ariya Rastrow
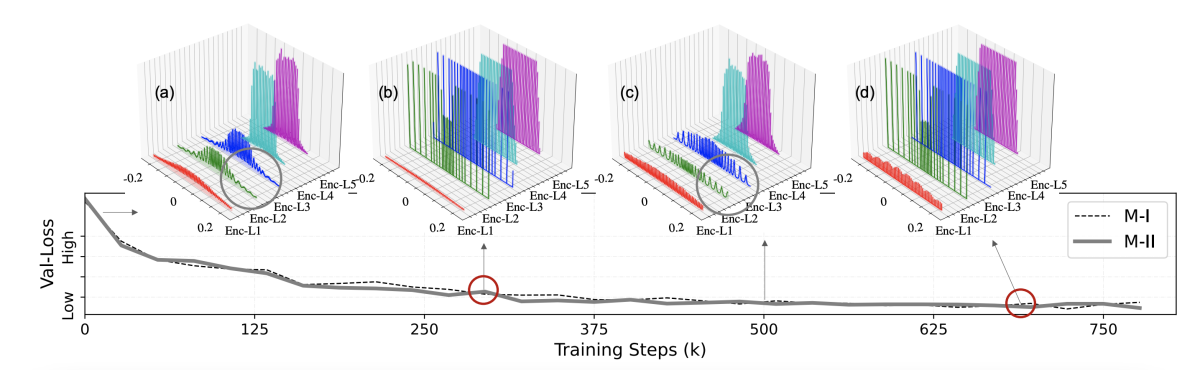
Signal processing
Clock skew robust acoustic echo cancellation
Karim Helwani, Erfan Soltanmohammadi, Michael M. Goodwin, Arvindh Krishnaswamy
Real-time packet loss concealment with mixed generative and predictive model
Jean-Marc Valin, Ahmed Mustafa, Christopher Montgomery, Timothy B. Terriberry, Michael Klingbeil, Paris Smaragdis, Arvindh Krishnaswamy
Speaker identification/verification
Adversarial reweighting for speaker verification fairness
Minho Jin, Chelsea J.-T. Ju, Zeya Chen, Yi Chieh Liu, Jasha Droppo, Andreas Stolcke
Graph-based multi-view fusion and local adaptation: Mitigating within household confusability for speaker identification
Long Chen, Yixiong Meng, Venkatesh Ravichandran, Andreas Stolcke
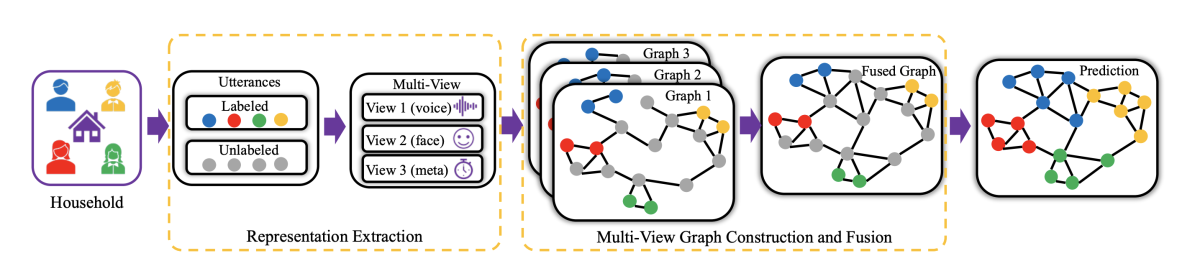
Spoken-language understanding
Learning under label noise for robust spoken language understanding systems
Anoop Kumar, Pankaj Sharma, Aravind Illa, Sriram Venkatapathy, Subhrangshu Nandi, Pritam Varma, Anurag Dwarakanath, Aram Galstyan
On joint training with interfaces for spoken language understanding
Anirudh Raju, Milind Rao, Gautam Tiwari, Pranav Dheram, Bryan Anderson, Zhe Zhang, Chul Lee, Bach Bui, Ariya Rastrow
Text-to-speech
Automatic evaluation of speaker similarity
Kamil Deja, Ariadna Sanchez, Julian Roth, Marius Cotescu
CopyCat2: A single model for multi-speaker TTS and many-to-many fine-grained prosody transfer
Sri Karlapati, Penny Karanasou, Mateusz Lajszczak, Ammar Abbas, Alexis Moinet, Peter Makarov, Ray Li, Arent van Korlaar, Simon Slangen, Thomas Drugman
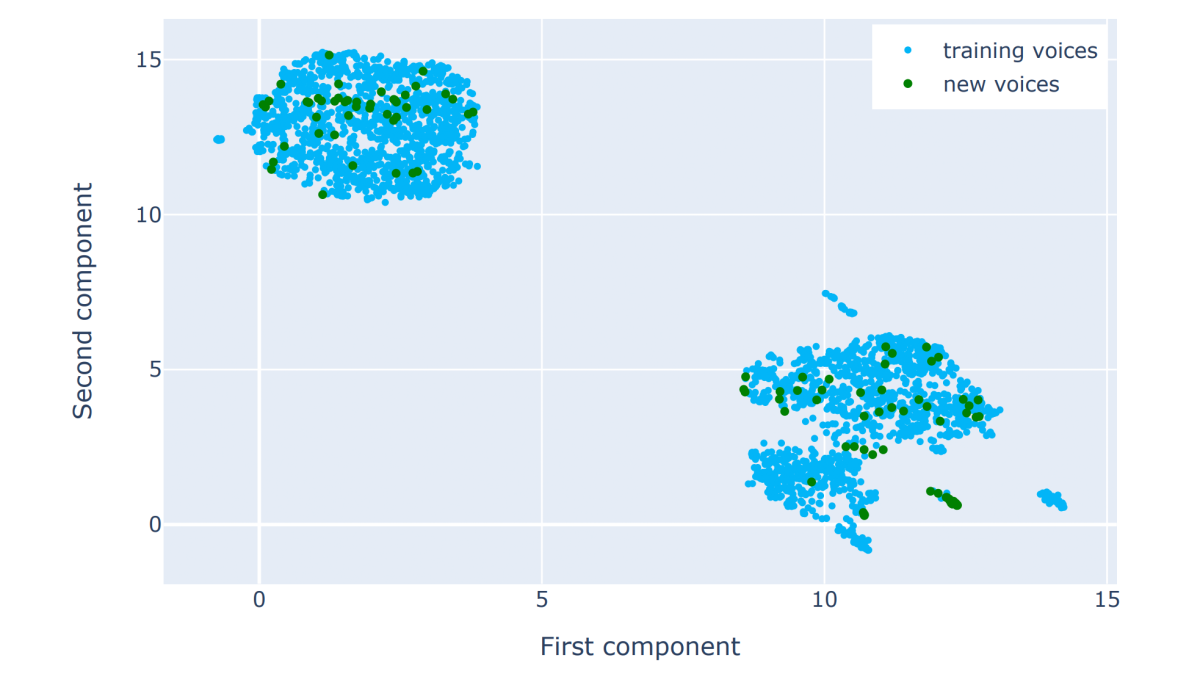
Creating new voices using normalizing flows
Piotr Biliński, Tom Merritt, Abdelhamid Ezzerg, Kamil Pokora, Sebastian Cygert, Kayoko Yanagisawa, Roberto Barra-Chicote, Daniel Korzekwa
Cross-lingual style transfer with conditional prior VAE and style loss
Dino Ratcliffe, You Wang, Alex Mansbridge, Penny Karanasou, Alexis Moinet, Marius Cotescu
End-to-end LPCNet: A neural vocoder with fully-differentiable LPC estimation
Krishna Subramani, Jean-Marc Valin, Umut Isik, Paris Smaragdis, Arvindh Krishnaswamy
Expressive, variable, and controllable duration modelling in TTS
Ammar Abbas, Tom Merritt, Alexis Moinet, Sri Karlapati, Ewa Muszynska, Simon Slangen, Elia Gatti, Thomas Drugman
GlowVC: Mel-spectrogram space disentangling model for language-independent text-free voice conversion
Magdalena Proszewska, Grzegorz Beringer, Daniel Saez Trigueros, Tom Merritt, Abdelhamid Ezzerg, Roberto Barra-Chicote
L2-GEN: A neural phoneme paraphrasing approach to L2 speech synthesis for mispronunciation diagnosis
Daniel Zhang, Ashwinkumar Ganesan, Sarah Campbell, Daniel Korzekwa
Low data? No problem: low resource, language-agnostic conversational text-to-speech via F0- conditioned data augmentation
Giulia Comini, Goeric Huybrechts, Manuel Sam Ribeiro, Adam Gabrys, Jaime Lorenzo Trueba
Mix and match: An empirical study on training corpus composition for polyglot text-to-speech (TTS)
Ziyao Zhang, Alessio Falai, Ariadna Sanchez, Orazio Angelini, Kayoko Yanagisawa
Simple and effective multi-sentence TTS with expressive and coherent prosody
Peter Makarov, Ammar Abbas, Mateusz Lajszczak, Arnaud Joly, Sri Karlapati, Alexis Moinet, Thomas Drugman, Penny Karanasou
Unify and conquer: How phonetic feature representation affects polyglot text-to-speech (TTS)
Ariadna Sanchez, Alessio Falai, Ziyao Zhang, Orazio Angelini, Kayoko Yanagisawa