Amazon’s 45-plus papers at the annual meeting of the North American chapter of the Association for Computational Linguistics, which begins next week, sorted by research area.
Continual learning
Lifelong pretraining: Continually adapting language models to emerging corpora
Xisen Jin, Dejiao Zhang, Henghui Zhu, Wei Xiao, Shang-Wen Li, Xiaokai Wei, Andrew O. Arnold, Xiang Ren
Local-to-global learning for iterative training of production SLU models on new features
Yulia Grishina, Daniil Sorokin
Overcoming catastrophic forgetting during domain adaptation of seq2seq language generation
Dingcheng Li, Zheng Chen, Eunah Cho, Jie Hao, Xiaohu Liu, Xing Fan, Chenlei (Edward) Guo, Yang Liu
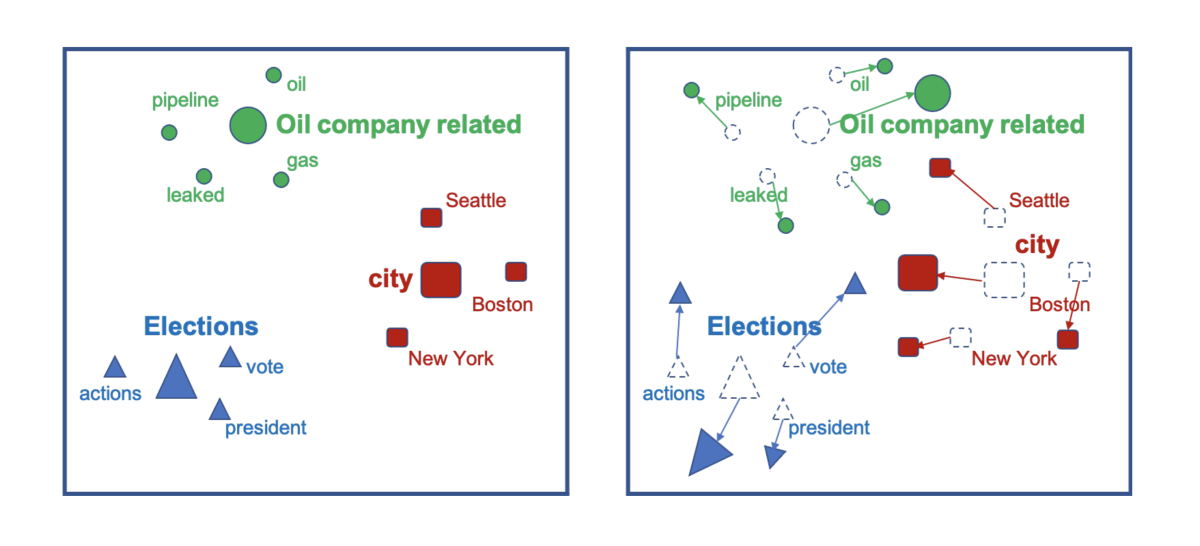
Temporal generalization for spoken language understanding
Judith Gaspers, Anoop Kumar, Greg Ver Steeg, Aram Galstyan
Data augmentation
Constraining word alignments with posterior regularization for label transfer
Kevin Martin Jose, Thomas Gueudré
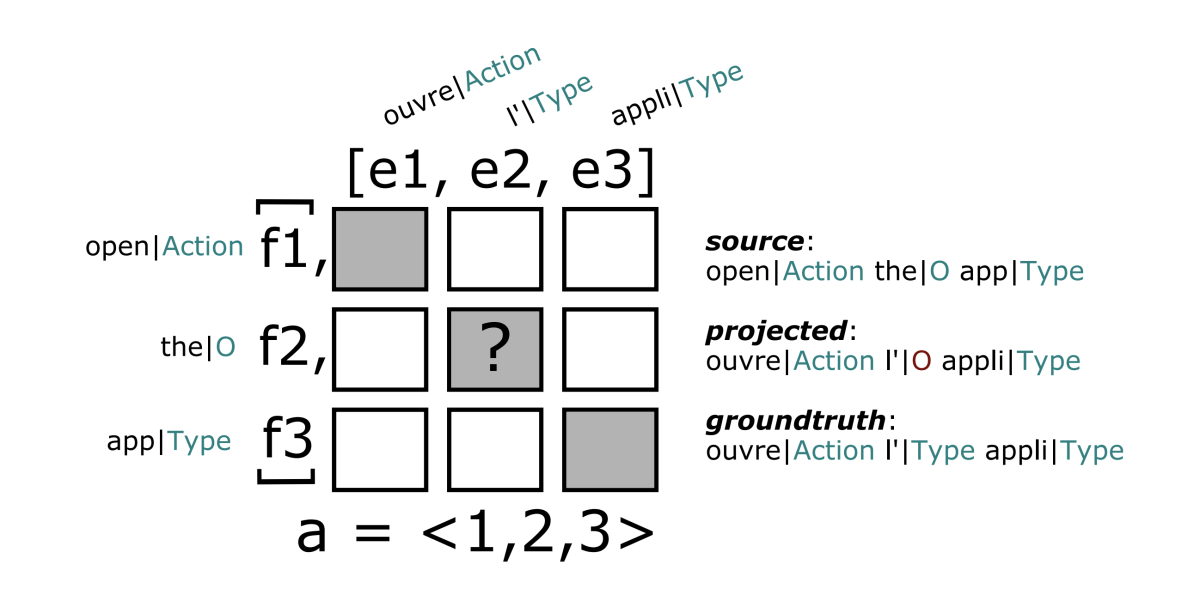
Controlled data generation via insertion operations for NLU
Manoj Kumar, Haidar Khan, Yuval Merhav, Wael Hamza, Anna Rumshisky, Rahul Gupta
Efficient semi supervised consistency training for natural language understanding
George Leung, Joshua Tan
Learning to generate examples for semantic processing tasks
Danilo Croce, Simone Filice, Giuseppe Castellucci, Roberto Basili
Dialogue
Learning dialogue representations from consecutive utterances
Zhihan Zhou, Dejiao Zhang, Wei Xiao, Nicholas Dingwall, Xiaofei Ma, Andrew O. Arnold, Bing Xiang
Massive-scale decoding for text generation using lattices
Jiacheng Xu, Siddhartha Reddy Jonnalagadda, Greg Durrett
Entity linking, resolution, and typing
Contrastive representation learning for cross-document coreference resolution of events and entities
Benjamin Hsu, Graham Horwood
Improving entity disambiguation by reasoning over a knowledge base
Tom Ayoola, Joseph Fisher, Andrea Pierleoni
ReFinED: An efficient zero-shot-capable approach to end-to-end entity linking
Tom Ayoola, Shubhi Tyagi, Joseph Fisher, Christos Christodoulopoulos, Andrea Pierleoni
Instilling type knowledge in language models via multi-task QA
Shuyang Li, Mukund Sridhar, Chandana Satya Prakash, Jin Cao, Wael Hamza, Julian McAuley
Explainable AI
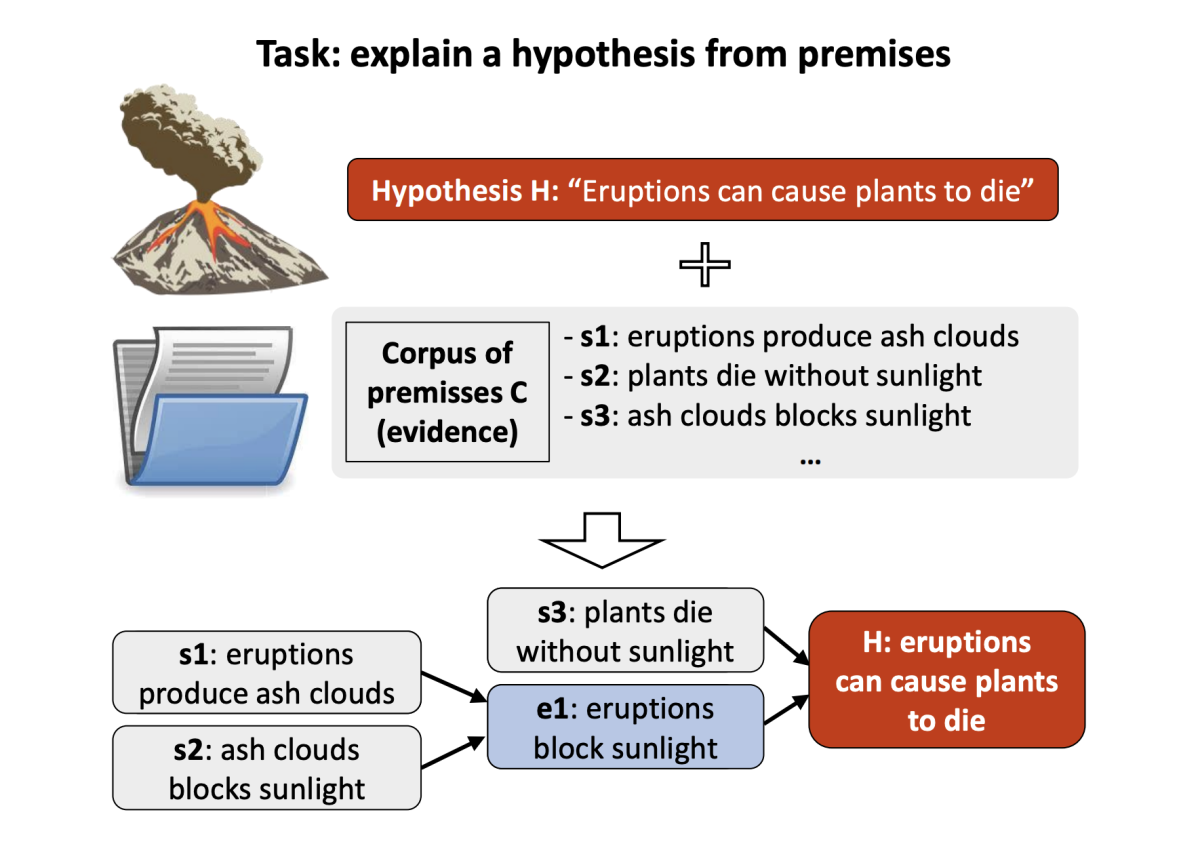
Entailment tree explanations via iterative retrieval-generation reasoner
Danilo Neves Ribeiro, Shen Wang, Xiaofei Ma, Rui Dong, Xiaokai Wei, Henry Zhu, Xinchi Chen, Zhiheng Huang, Peng Xu, Andrew O. Arnold, Dan Roth
Locally aggregated feature attribution on natural language model understanding
Sheng Zhang, Jin Wang, Haitao Jiang, Rui Song
Extreme multilabel classification
Augmenting training data for massive semantic matching models in low-traffic e-commerce stores
Ashutosh Joshi, Shankar Vishwanath, Choon Hui Teo, Vaclav Petricek, Vishy Vishwanathan, Rahul Bhagat, Jonathan May
Extreme zero shot learning for extreme text classification
Yuanhao Xiong, Wei-Cheng Chang, Cho-Jui Hsieh, Hsiang-Fu Yu, Inderjit S. Dhillon
Federated learning
Federated learning with noisy user feedback
Rahul Sharma, Anil Ramakrishna, Ansel MacLaughlin, Anna Rumshisky, Jimit Majmudar, Clement Chung, Salman Avestimehr, Rahul Gupta
Keyword spotting
AB/BA analysis: A framework for estimating keyword spotting recall improvement while maintaining audio privacy
Raphael Petegrosso, Vasistakrishna Baderdinni, Thibaud Senechal, Benjamin L. Bullough
Machine translation
CoCoA-MT: A dataset and benchmark for contrastive controlled MT with application to formality
Maria Nadejde, Anna Currey, Benjamin Hsu, Xing Niu, Marcello Federico, Georgiana Dinu
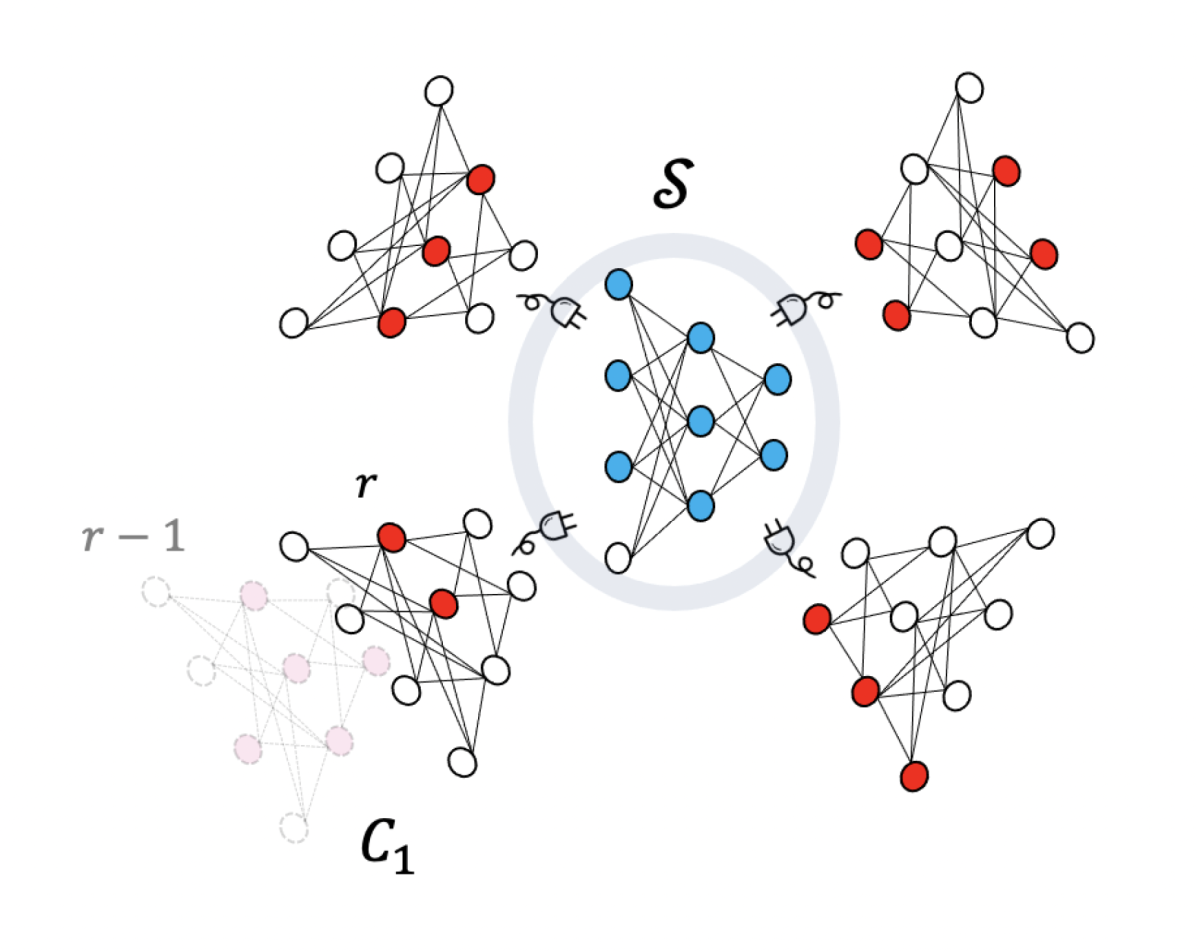
The devil is in the details: On the pitfalls of vocabulary selection in neural machine translation
Tobias Domhan, Eva Hasler, Ke Tran, Sony Trenous, Bill Byrne, Felix Hieber
Training mixed-domain translation models via federated learning
Peyman Passban, Tanya G. Roosta, Rahul Gupta, Ankit Chadha, Clement Chung
Multitask learning
Asynchronous convergence in multi-task learning via knowledge distillation from converged tasks
Weiyi Lu, Sunny Rajagopalan, Priyanka Nigam, Jaspreet Singh, Xiaodi Sun, Yi Xu, Belinda Zeng, Trishul Chilimbi
Exploring the role of task transferability in large-scale multi-task learning
Vishakh Padmakumar, Leonard Lausen, Miguel Ballesteros, Sheng Zha, He He, George Karypis
Named-entity recognition
Dynamic gazetteer integration in multilingual models for cross-lingual and cross-domain named entity recognition
Besnik Fetahu, Anjie Fang, Oleg Rokhlenko, Shervin Malmasi
NER-MQMRC: Formulating named entity recognition as multi question machine reading comprehension
Anubhav Shrimal, Avi Jain, Kartik Mehta, Promod Yenigalla
Question answering
Answer consolidation: Formulation and benchmarking
Wenxuan Zhou, Qiang Ning, Heba Elfardy, Kevin Small, Muhao Chen
Paragraph-based transformer pre-training for multi-sentence inference
Luca Di Liello, Siddhant Garg, Luca Soldaini, Alessandro Moschitti
PerKGQA: Question answering over personalized knowledge graphs
Ritam Dutt, Kasturi Bhattacharjee, Rashmi Gangadharaiah, Dan Roth, Carolyn Penstein Rosé
Product answer generation from heterogeneous sources: A new benchmark and best practices
Xiaoyu Shen, Gianni Barlacchi, Marco Del Tredici, Weiwei Cheng, Adria de Gispert, Bill Byrne
Recommender systems
CERES: Pretraining of graph-conditioned transformer for semi-structured session data
Rui Feng, Chen Luo, Qingyu Yin, Bing Yin, Tuo Zhao, Chao Zhang
Self-learning
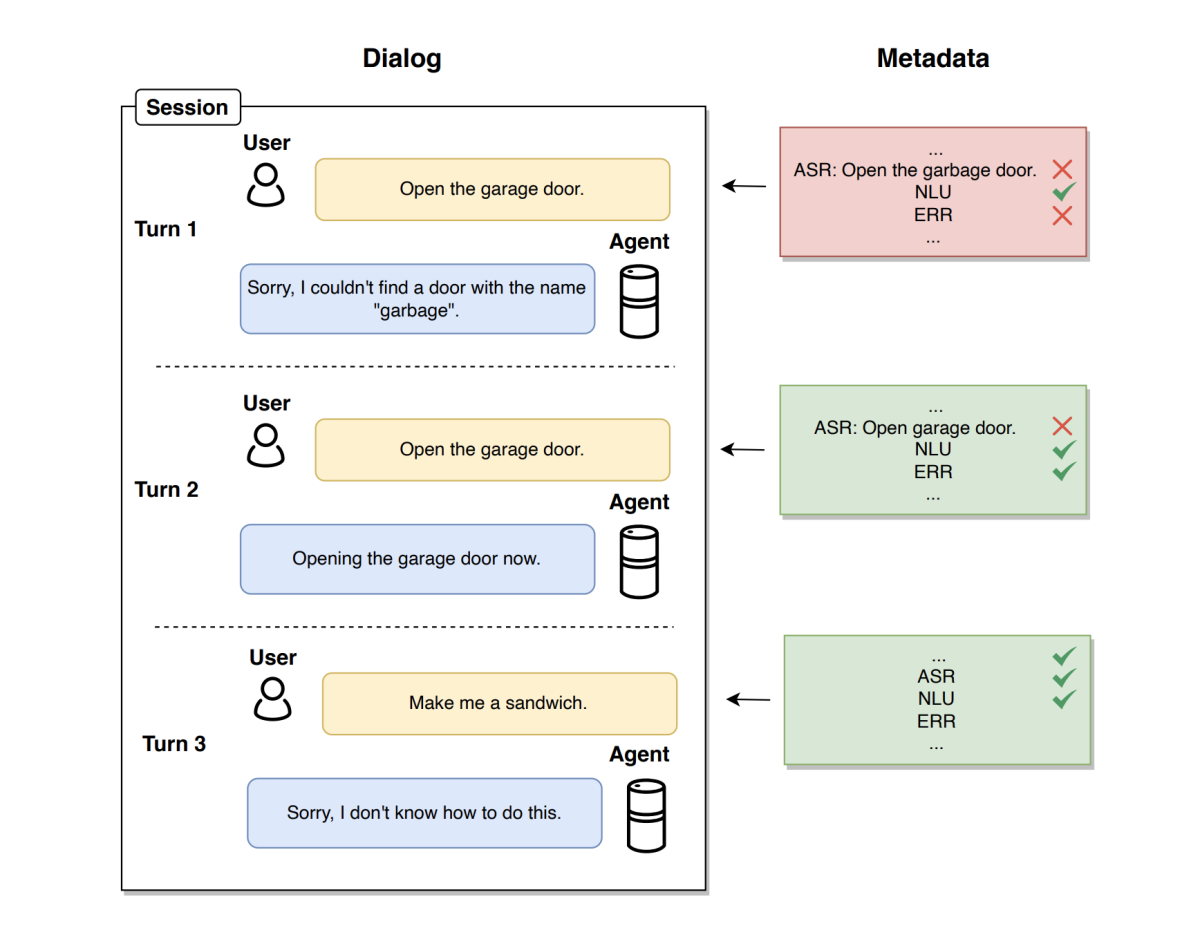
FPI: Failure point isolation in large-scale conversational assistants
Rinat Khaziev, Usman Shahid, Tobias Röding, Rakesh Chada, Emir Kapanci, Pradeep Natarajan
Scalable and robust self-learning for skill routing in large-scale conversational AI systems
Mohammad Kachuee, Jinseok Nam, Sarthak Ahuja, Jin-Myung Won, Sungjin Lee
Self-aware feedback-based self-learning in large-scale conversational AI
Pragaash Ponnusamy, Clint Solomon Mathialagan, Gustavo Aguilar, Chengyuan Ma, Chenlei (Edward) Guo
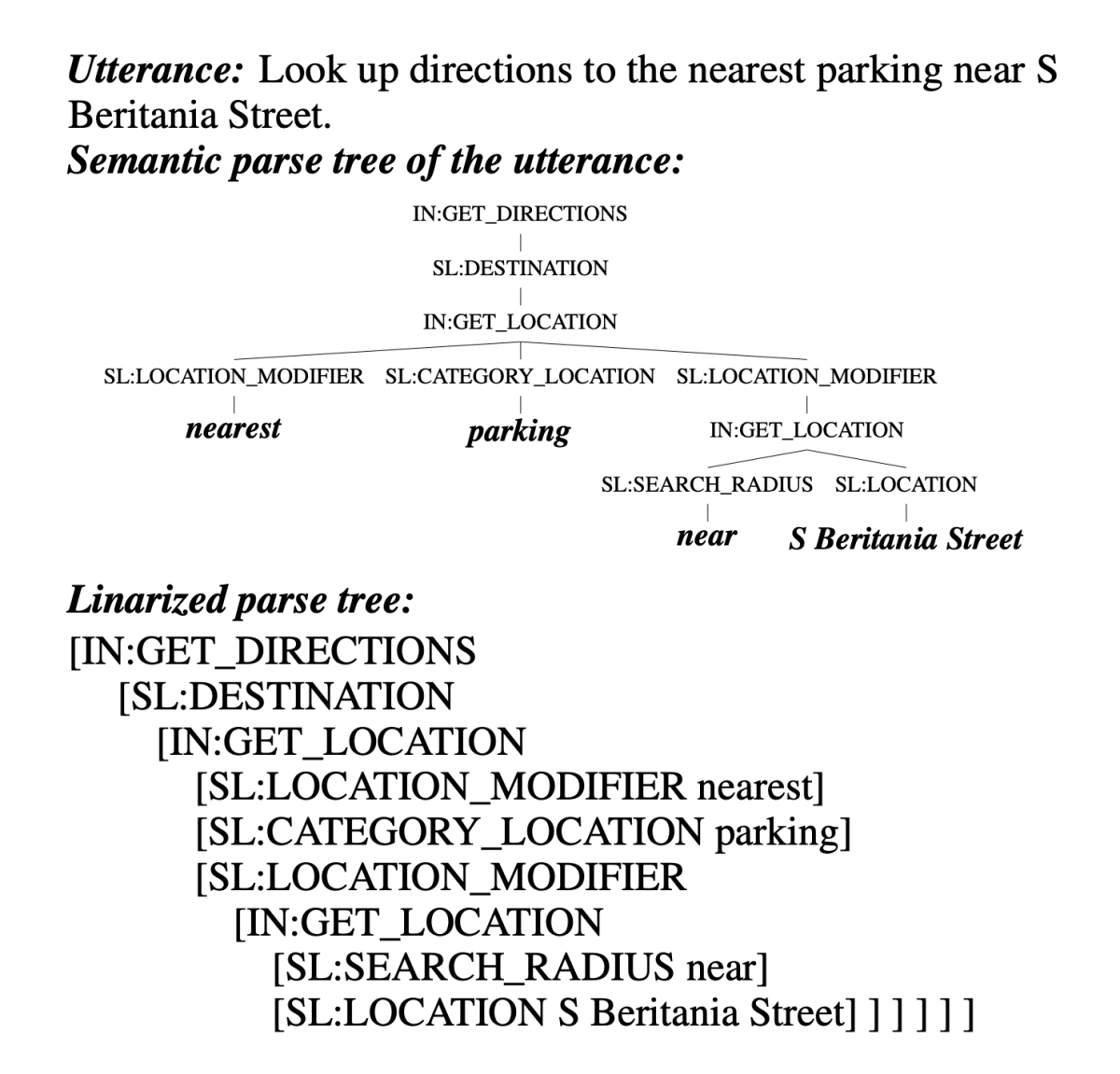
Semantic parsing
Compositional task oriented parsing as abstractive question answering
Wenting Zhao, Konstantine Arkoudas, Weiqi Sun, Claire Cardie
SeqZero: Few-shot compositional semantic parsing with sequential prompts and zero-shot models
Jingfeng Yang, Haoming Jiang, Qingyu Yin, Danqing Zhang, Bing Yin, Diyi Yang
Task adaptation
Attention fusion: A light yet efficient late fusion mechanism for task adaptation in NLU
Jin Cao, Chandana Satya Prakash, Wael Hamza
Empowering parameter-efficient transfer learning by recognizing the kernel structure in attention
Yifan Chen, Devamanyu Hazarika, Mahdi Namazifar, Yang Liu, Di Jin, Dilek Hakkani-Tür
Text mining
Distantly supervised aspect clustering and naming for e-commerce reviews
Prateek Sircar, Aniket Chakrabarti, Deepak Gupta, Anirban Majumdar
Efficient few-shot fine-tuning for opinion summarization
Arthur Bražinskas, Ramesh Nallapati, Mohit Bansal, Markus Dreyer
FactGraph: Evaluating factuality in summarization with semantic graph representations
Leonardo F. R. Ribeiro, Mengwen Liu, Iryna Gurevych, Markus Dreyer, Mohit Bansal
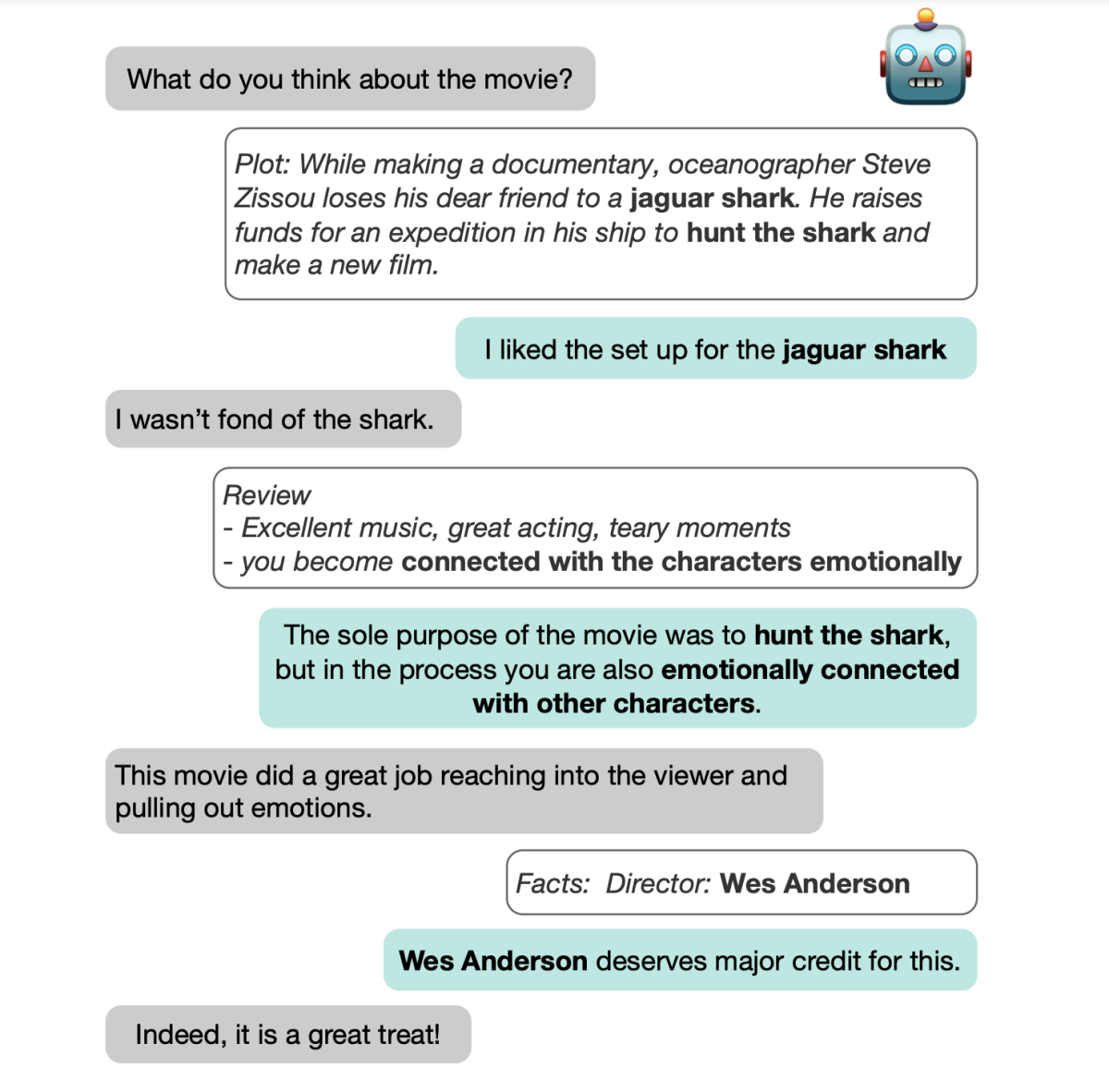
Enhanced knowledge selection for grounded dialogues via document semantic graphs
Sha Li, Madhi Namazifar, Di Jin, Mohit Bansal, Heng Ji, Yang Liu, Dilek Hakkani-Tür
Retrieval-augmented multilingual keyphrase generation with retriever-generator iterative training
Yifan Gao, Qingyu Yin, Zheng Li, Rui Meng, Tong Zhao, Bing Yin, Irwin King, Michael R. Lyu
What do users care about? Detecting actionable insights from user feedback
Kasturi Bhattacharjee, Rashmi Gangadharaiah, Kathleen McKeown, Dan Roth
Text-to-speech
Empathic machines: using intermediate features as levers to emulate emotions in text-to-speech systems
Saiteja Kosgi, Sarath Sivaprasad, Niranjan Pedanekar, Anil Nelakanti, Vineet Gandhi