Like the field of conversational AI in general, Amazon’s papers at this year’s meeting of the Association for Computational Linguistics (ACL) are dominated by work on large language models (LLMs). The properties that make LLMs’ outputs so extraordinary — such as their linguistic fluency and semantic coherence — are also notoriously difficult to quantify; as such, model evaluation has emerged as a particular area of focus. But Amazon’s papers explore a wide range of LLM-related topics, from applications such as code synthesis and automatic speech recognition to problems of LLM training and deployment, such as continual pretraining and hallucination mitigation. Papers accepted to the recently inaugurated Proceedings of the ACL are marked with asterisks.
Code synthesis
Fine-tuning language models for joint rewriting and completion of code with potential bugs
Dingmin Wang, Jinman Zhao, Hengzhi Pei, Samson Tan, Sheng Zha
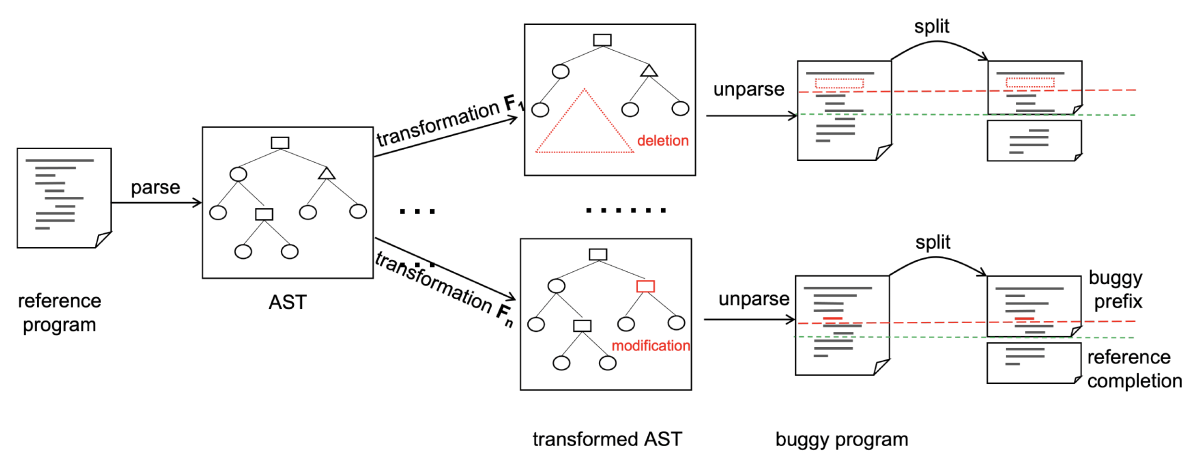
Continual pretraining
Efficient continual pre-training for building domain specific large language models*
Yong Xie, Karan Aggarwal, Aitzaz Ahmad
Data quality
A shocking amount of the web is machine translated: Insights from multi-way parallelism*
Brian Thompson, Mehak Dhaliwal, Peter Frisch, Tobias Domhan, Marcello Federico
Document summarization
The power of summary-source alignments
Ori Ernst, Ori Shapira, Aviv Slobodkin, Sharon Adar, Mohit Bansal, Jacob Goldberger, Ran Levy, Ido Dagan
Hallucination mitigation
Learning to generate answers with citations via factual consistency models
Rami Aly, Zhiqiang Tang, Samson Tan, George Karypis
Intent classification
Can your model tell a negation from an implicature? Unravelling challenges with intent encoders
Yuwei Zhang, Siffi Singh, Sailik Sengupta, Igor Shalyminov, Hwanjun Song, Hang Su, Saab Mansour
Irony recognition
MultiPICo: Multilingual perspectivist irony corpus
Silvia Casola, Simona Frenda, Soda Marem Lo, Erhan Sezerer, Antonio Uva, Valerio Basile, Cristina Bosco, Alessandro Pedrani, Chiara Rubagotti, Viviana Patti, Davide Bernardi
Knowledge grounding
Graph chain-of-thought: Augmenting large language models by reasoning on graphs
Bowen Jin, Chulin Xie, Jiawei Zhang, Kashob Kumar Roy, Yu Zhang, Zheng Li, Ruirui Li, Xianfeng Tang, Suhang Wang, Yu Meng, Jiawei Han
MATTER: Memory-augmented transformer using heterogeneous knowledge sources*
Dongkyu Lee, Chandana Satya Prakash, Jack G. M. FitzGerald, Jens Lehmann
Tree-of-traversals: A zero-shot reasoning algorithm for augmenting black-box language models with knowledge graphs
Elan Markowitz, Anil Ramakrishna, Jwala Dhamala, Ninareh Mehrabi, Charith Peris, Rahul Gupta, Kai-Wei Chang, Aram Galstyan
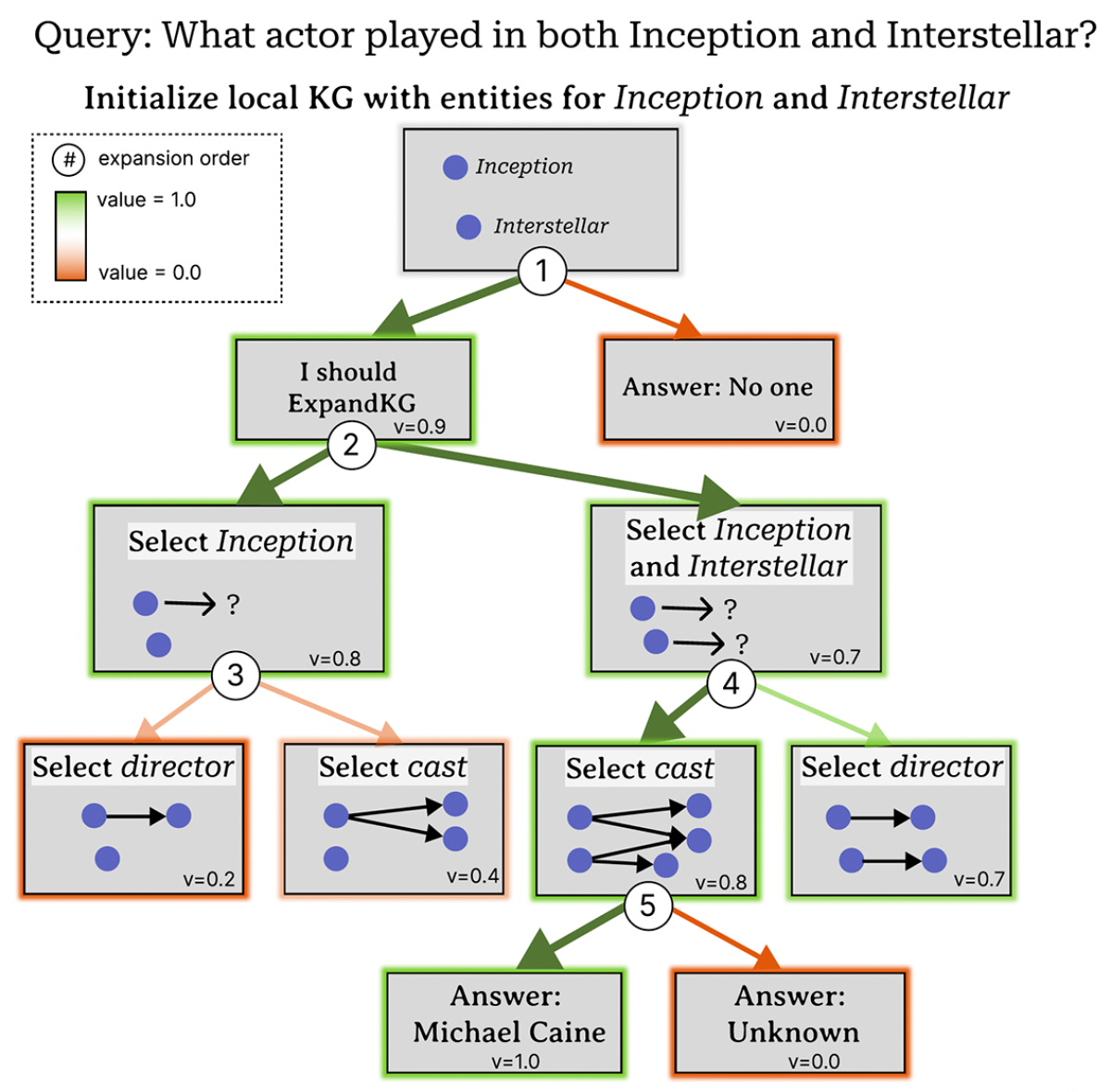
LLM decoding
BASS: Batched attention-optimized speculative sampling*
Haifeng Qian, Sujan Gonugondla, Sungsoo Ha, Mingyue Shang, Sanjay Krishna Gouda, Ramesh Nallapati, Sudipta Sengupta, Anoop Deoras
Machine translation
Impacts of misspelled queries on translation and product search
Greg Hanneman, Natawut Monaikul, Taichi Nakatani
The fine-tuning paradox: Boosting translation quality without sacrificing LLM abilities
David Stap, Eva Hasler, Bill Byrne, Christof Monz, Ke Tran
Model editing
Propagation and pitfalls: Reasoning-based assessment of knowledge editing through counterfactual tasks
Wenyue Hua, Jiang Guo, Marvin Dong, Henghui Zhu, Patrick Ng, Zhiguo Wang
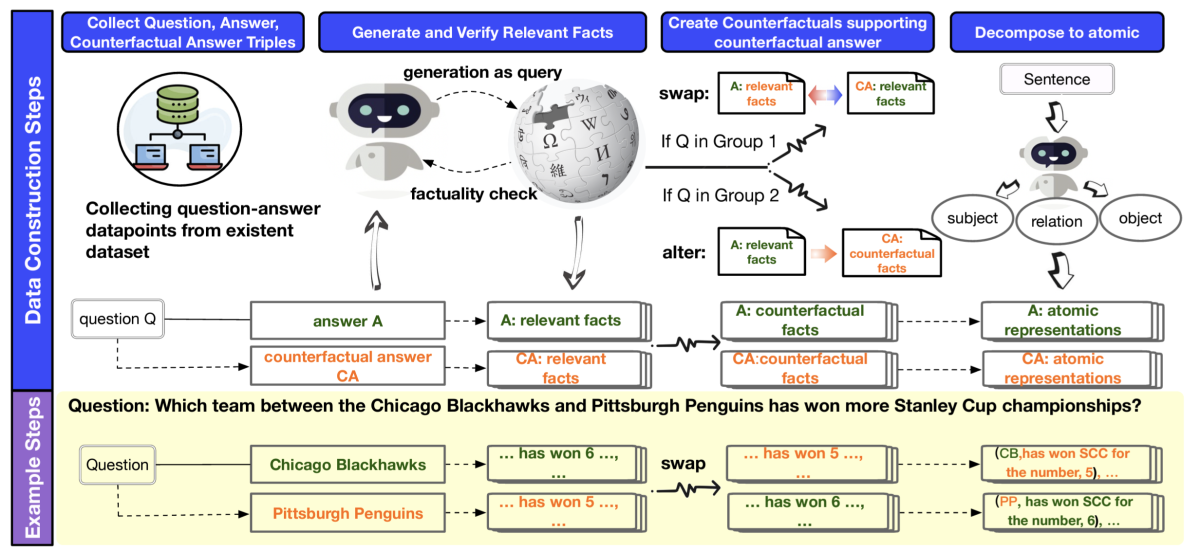
Model evaluation
Bayesian prompt ensembles: Model uncertainty estimation for black-box large language models
Francesco Tonolini, Jordan Massiah, Nikolaos Aletras, Gabriella Kazai
ConSiDERS—the-human evaluation framework: Rethinking human evaluation for generative large language models
Aparna Elangovan, Ling Liu, Lei Xu, Sravan Bodapati, Dan Roth
Factual confidence of LLMs: On reliability and robustness of current estimators
Matéo Mahaut, Laura Aina, Paula Czarnowska, Momchil Hardalov, Thomas Müller, Lluís Marquez
Fine-tuned machine translation metrics struggle in unseen domains
Vilém Zouhar, Shuoyang Ding, Anna Currey, Tatyana Badeka, Jenyuan Wang, Brian Thompson
Measuring question answering difficulty for retrieval-augmented generation
Matteo Gabburo, Nicolaas Jedema, Siddhant Garg, Leonardo Ribeiro, Alessandro Moschitti
Model robustness
Extreme miscalibration and the illusion of adversarial robustness
Vyas Raina, Samson Tan, Volkan Cevher, Aditya Rawal, Sheng Zha, George Karypis
Multimodal models
CaMML: Context-aware multimodal learner for large models
Yixin Chen, Shuai Zhang, Boran Han, Tong He, Bo Li
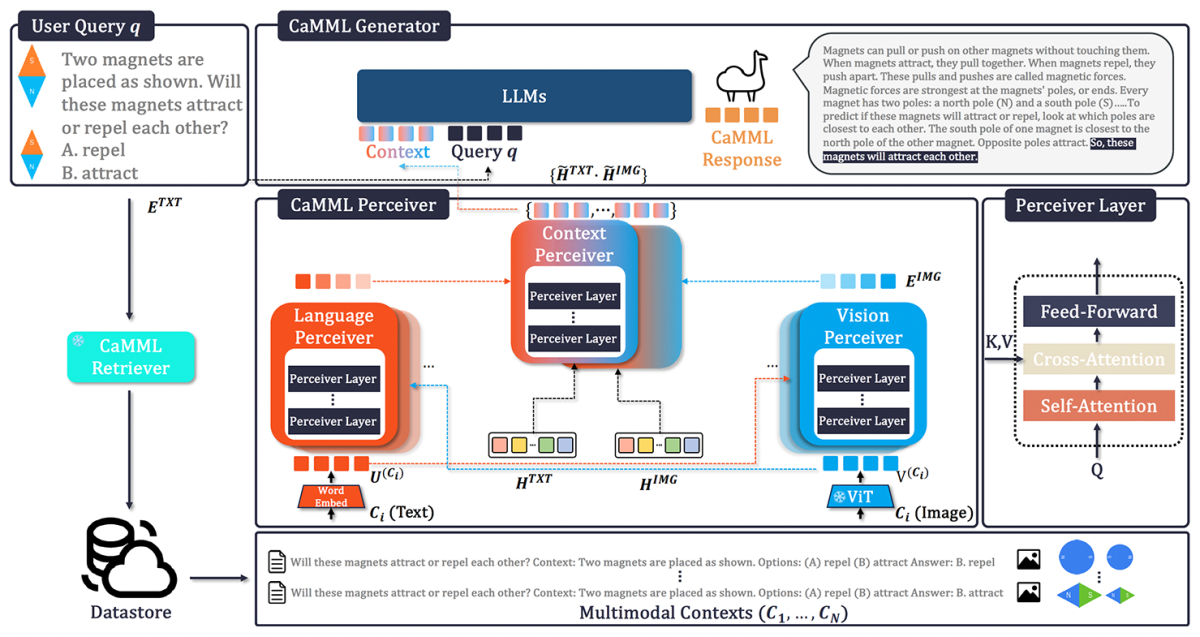
Multi-modal retrieval for large language model based speech recognition
Jari Kolehmainen, Aditya Gourav, Prashanth Gurunath Shivakumar, Yi Gu, Ankur Gandhe, Ariya Rastrow, Grant Strimel, Ivan Bulyko
REFINESUMM: Self-refining MLLM for generating a multimodal summarization dataset
Vaidehi Patil, Leonardo Ribeiro, Mengwen Liu, Mohit Bansal, Markus Dreyer
Ordinal classification
Exploring ordinality in text classification: A comparative study of explicit and implicit techniques
Siva Rajesh Kasa, Aniket Goel, Sumegh Roychowdhury, Karan Gupta, Anish Bhanushali, Nikhil Pattisapu, Prasanna Srinivasa Murthy
Question answering
Beyond boundaries: A human-like approach for question answering over structured and unstructured information sources*
Jens Lehmann, Dhananjay Bhandiwad, Preetam Gattogi, Sahar Vahdati
MinPrompt: Graph-based minimal prompt data augmentation for few-shot question answering
Xiusi Chen, Jyun-Yu Jiang, Wei-Cheng Chang, Cho-Jui Hsieh, Hsiang-Fu Yu, Wei Wang
Synthesizing conversations from unlabeled documents using automatic response segmentation
Fanyou Wu, Weijie Xu, Chandan Reddy, Srinivasan Sengamedu, "SHS"
Reasoning
Eliciting better multilingual structured reasoning from LLMs through code
Bryan Li, Tamer Alkhouli, Daniele Bonadiman, Nikolaos Pappas, Saab Mansour
II-MMR: Identifying and improving multi-modal multi-hop reasoning in visual question answering*
Jihyung Kil, Farideh Tavazoee, Dongyeop Kang, Joo-Kyung Kim
Recommender systems
Generative explore-exploit: Training-free optimization of generative recommender systems using LLM optimizers
Besnik Fetahu, Zhiyu Chen, Davis Yoshida, Giuseppe Castellucci, Nikhita Vedula, Jason Choi, Shervin Malmasi
Towards translating objective product attributes into customer language
Ram Yazdi, Oren Kalinsky, Alexander Libov, Dafna Shahaf
Responsible AI
SpeechGuard: Exploring the adversarial robustness of multimodal large language models
Raghuveer Peri, Sai Muralidhar Jayanthi, Srikanth Ronanki, Anshu Bhatia, Karel Mundnich, Saket Dingliwal, Nilaksh Das, Zejiang Hou, Goeric Huybrechts, Srikanth Vishnubhotla, Daniel Garcia-Romero, Sundararajan Srinivasan, Kyu Han, Katrin Kirchhoff
Text completion
Token alignment via character matching for subword completion*
Ben Athiwaratkun, Shiqi Wang, Mingyue Shang, Yuchen Tian, Zijian Wang, Sujan Gonugondla, Sanjay Krishna Gouda, Rob Kwiatkowski, Ramesh Nallapati, Bing Xiang
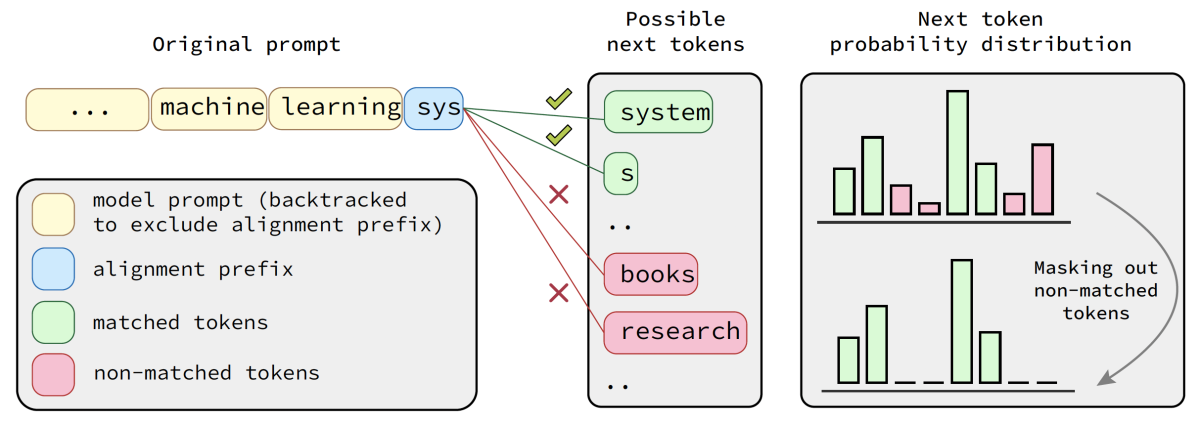