Neural machine translation systems are often optimized to perform well for specific text genres or domains, such as newspaper articles, user manuals, or customer support chats.
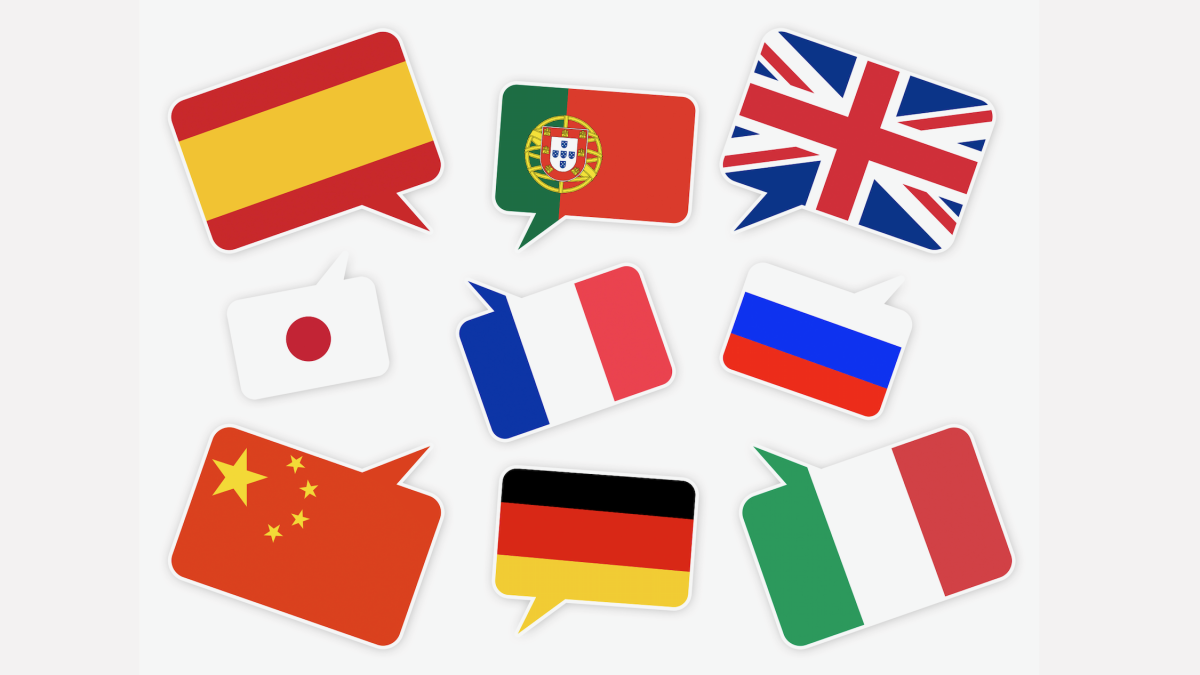
In industrial settings with hundreds of language pairs to serve, however, a single translation system per language pair, which performs well across different text domains, is more efficient to deploy and maintain. Additionally, service providers may not know in advance which domains customers will be interested in.
At this year’s Conference on Empirical Methods in Natural Language Processing (EMNLP), we are presenting a new approach to multidomain adaptation for neural translation models, or adapting an existing model to new domains while maintaining translation quality in the original domain. Our approach provides a better trade-off between performance on old and new tasks than its predecessors do.
We combine two domain-adaptation techniques known as elastic weight consolidation and data mixing, and our paper draws a theoretical connection between them that explains why they work well together.
Both are techniques for preventing catastrophic forgetting, where a model forgets the task it had originally learned when trying to learn a new task. Elastic weight consolidation (EWC) constrains the way the model’s parameters are updated, while data mixing is a data-driven strategy that exposes the translation system to old and new data at the same time.
We show in our experiments that EWC combined with data mixing yields strong improvements on the original task, according to BLEU score, a common machine translation quality metric based on word overlap with a reference translation. Relative to EWC on its own, our system improves performance on existing tasks by 2 BLEU points for a German-to-English translation system and 0.8 BLEU points for English to French, while maintaining comparable performance on the new tasks. On the other hand, combination with EWC improves over data mixing on its own by providing a parameter to control the performance on original versus new tasks.
A more intuitive loss function
Imagine we have a translation system that has learned to translate news articles, political debates, and user manuals, and we want to adapt it to handle customer support chats and medical reports. So we expose our already trained system to chat and medical-translation examples.
During adaptation, we do not want the model to forget how to translate news articles, political debates, and user manuals. Elastic weight consolidation encourages updates to the model parameters in such a way that existing knowledge stored in the parameters will be preserved. How much knowledge will be preserved versus how much new information will be taken in is controlled by a hyperparameter, λ.
For data mixing, the translation examples from chats and medical reports will be complemented by a sample from the existing news, political-debate, and user manuals data. The mixing ratio is typically 1:1 but can be varied to shift the balances between old and new tasks.
Our work provides a theoretical analysis that shows how these two very different strategies are connected. A machine learning algorithm learns based on a loss function — an equation that states the goal of the learning process. We can derive a loss function for the combination of EWC and data mixing that has a better intuitive motivation than the original loss function for EWC.
The details are in the paper, but the basic idea is that the standard EWC loss function assumes that the tasks being learned are conditionally independent — that good performance on one task is unrelated to good performance on the other.
With translation, this is unlikely to be the case: representations useful for one task may very well be useful for the other. So we relax the assumption of conditional independence, assuming instead that there is some subset of the training data for one task that captures general information about the problem space useful for the second task. Then we derive a loss fuction that incorporates our new assumption.
The term we add to the loss function is equivalent to mixing a sample of the existing data into the new data. So our analysis provides a theoretical foundation for our intuition that combining EWC and data mixing should work better than using either on its own.
Which learning strategy to choose? Both!
We experimented with EWC, data mixing, and their combination on publicly available data sets for German to English (DE→EN) and English to French (EN→FR) translation systems. The figures below show our results as measured by mean BLEU scores on news articles (representing old tasks) and mean BLEU scores on three new domains per language pair. The baseline score represents our current translation system and “adapt” represents a system that is updated naively, without consideration for the performance on the old tasks.
Our results show that although EWC succeeds in mitigating catastrophic forgetting, as seen by the reduced drop in BLEU on news articles, this comes at a considerable cost in terms of new-domain quality.
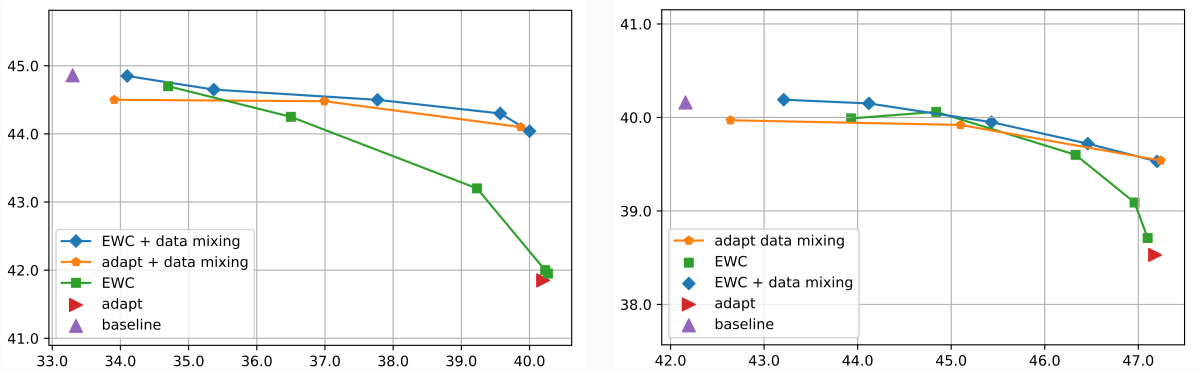
In comparison, data mixing with a 1:1 ratio of old and new data allows for high quality on the new domains while retaining substantially higher performance on old tasks (rightmost point on data-mixing curve). However, even increasing the ratio of old to new data to 100:1 doesn’t recover the baseline performance on the old task (translation of news articles). Thanks to the strength parameter λ, the combination of EWC and data mixing yields the overall best performance.
Multidomain adaptation is a relevant area of research for Amazon Translate, the real-time machine translation service from AWS that supports translation between hundreds of languages for a growing and diverse set of customer use cases and domains. This paper complements our previously published work that introduced a multidomain adaptation strategy with model distillation.