This year at the IEEE Automatic Speech Recognition and Understanding (ASRU) Workshop, Alexa researchers have two papers about training machine learning systems with minimal hand-annotated data. Both papers describe automated methods for producing training data, and both describe additional algorithms for extracting just the high-value examples from that data.
Each paper, however, gravitates to a different half of the workshop’s title: one is on speech recognition, or converting an acoustic speech signal to text, and the other is on natural-language understanding, or determining a text’s meaning.
The natural-language-understanding (NLU) paper is about adding new functions to a voice agent like Alexa when training data is scarce. It involves “self-training”, in which a machine learning model trained on sparse annotated data itself labels a large body of unannotated data, which in turn is used to re-train the model.
The researchers investigate techniques for winnowing down the unannotated data, to extract examples pertinent to the new function, and then winnowing it down even further, to remove redundancies.
The automatic-speech-recognition (ASR) paper is about machine-translating annotated data from a language that Alexa already supports to produce training data for a new language. There, too, the researchers report algorithms for identifying data subsets — both before and after translation — that will yield a more-accurate model.
Three of the coauthors on the NLU paper — applied scientists Eunah Cho and Varun Kumar and applied-scientist manager Bill Campbell — are also among the five Amazon organizers of the Life-Long Learning for Spoken-Language Systems workshop, which will take place on the first day of ASRU. The workshop focuses on the problem of continuously improving deployed conversational-AI systems.
Cho and her colleagues’ main-conference paper, “Efficient Semi-Supervised Learning for Natural Language Understanding by Optimizing Diversity”, addresses an instance of that problem: teaching Alexa to recognize new “intents”.
Enlarged intents
Alexa’s NLU models classify customer requests according to domain, or the particular service that should handle a request, and intent, or the action that the customer wants executed. They also identify the slot types of the entities named in the requests, or the roles those entities play in fulfilling the request. In the request “Play ‘Undecided’ by Ella Fitzgerald”, for instance, the domain is Music and the intent PlayMusic, and the names “Undecided” and “Ella Fitzgerald” fill the slots SongName and ArtistName.
Most intents have highly specific vocabularies (even when they’re large, as in the case of the PlayMusic intent), and ideally, the training data for a new intent would be weighted toward in-vocabulary utterances. But when Alexa researchers are bootstrapping a new intent, intent-specific data is scarce. So they need to use training data extracted from more-general text corpora.
As a first pass at extracting intent-relevant data from a general corpus, Cho and her colleagues use a simple n-gram-based linear logistic regression classifier, trained on whatever annotated, intent-specific data is available. The classifier breaks every input utterance into overlapping one-word, two-word, and three-word chunks — n-grams — and assigns each chunk a score, indicating its relevance to the new intent. The relevance score for an utterance is an aggregation of the chunks’ scores, and the researchers keep only the most relevant examples.
In an initial experiment, the researchers used sparse intent-specific data to train five different machine learning models to recognize five different intents. Then they fed unlabeled examples extracted by the regression classifier to each intent recognizer. The recognizers labeled the examples, which were then used to re-train the recognizers. On average, this reduced the recognizers’ error rates by 15%.
To make this process more efficient, Cho and her colleagues trained a neural network to identify paraphrases, which are defined as pairs of utterances that have the same domain, intent, and slot labels. So “I want to listen to Adele” is a paraphrase of “Play Adele”, but “Play Seal” is not.
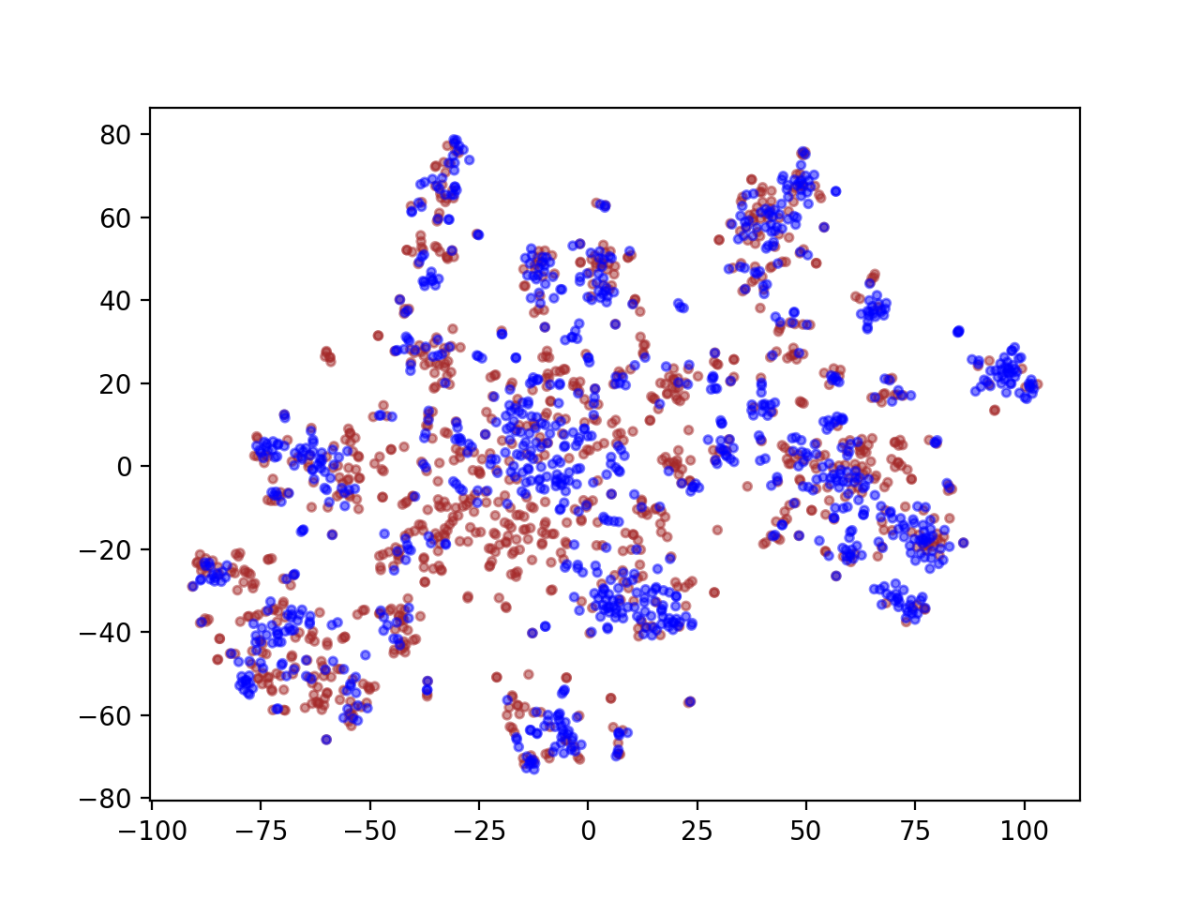
The researchers wanted their paraphrase detector to be as general as possible, so they trained it on data sampled from Alexa’s full range of domains and intents. From each sample, they produced a template by substituting slot types for slot values. So, for instance, “Play Adele in the living room” became something like “Play [artist_name] in the [device_location].” From those templates, they could generate as comprehensive a set of training pairs as they wanted — paraphrases with many different sentence structures and, as negative examples, non-paraphrases with the same sentence structures.
From the data set extracted by the logistic classifier, the paraphrase detector selects a small batch of examples that offer bad paraphrases of the examples in the intent-specific data set. The idea is that bad paraphrases will help diversify the data, increasing the range of inputs the resulting model can handle.
The bad paraphrases are added to the annotated data, producing a new augmented data set, and then the process is repeated. This method halves the amount of training data required to achieve the error rate improvements the researchers found in their first experiment.
Gained in translation
The other ASRU paper, “Language Model Bootstrapping Using Neural Machine Translation for Conversational Speech Recognition”, is from applied scientist Surabhi Punjabi, senior applied scientist Harish Arsikere, and senior manager for machine learning Sri Garimella, all of the Alexa Speech group. It investigates building an ASR system in a language — in this case, Hindi — in which little annotated training data is available.
ASR systems typically have several components. One, the acoustic model, takes a speech signal as input and outputs phonetic renderings of short speech sounds. A higher-level component, the language model, encodes statistics about the probabilities of different word sequences. It can thus help distinguish between alternate interpretations of the same acoustic signal (for instance, “Pulitzer Prize” versus “pullet surprise”).
Punjabi and her colleagues investigated building a Hindi language model by automatically translating annotated English-language training data into Hindi. The first step was to train a neural-network-based English-Hindi translator. This required a large body of training data, which matched English inputs to Hindi translations.
Here the researchers ran into a problem similar to the one that Cho and her colleagues confronted. By design, the available English-Hindi training sets were drawn from a wide range of sources and covered a wide range of topics. But the annotated English data that the researchers wanted to translate was Alexa-specific.
Punjabi and her colleagues started with a limited supply of Alexa-specific annotated data in Hindi, collected through Cleo, an Alexa skill that allows multilingual customers to help train machine learning models in new languages. Using an off-the-shelf statistical model, they embedded that data, or represented each sentence as a point in a geometric space, such that sentences with similar meanings clustered together.
Then they embedded Hindi sentences extracted from a large, general, English-Hindi bilingual corpus and measured their distance from the average embedding of the Cleo data. To train their translator, they used just those sentences within a fixed distance of the average — that is, sentences whose meanings were similar to those of the Cleo data.
In one experiment, they then used self-training to fine-tune the translator. After the translator had been trained, they used it to translate a subset of the English-only Alexa-specific data. Then they used the resulting English-Hindi sentence pairs to re-train the translator.
Like all neural translators, Punjabi and her colleagues’ outputs a list of possible translations, ranked according to the translator’s confidence that they’re accurate. In another experiment, the researchers used a simple language model, trained only on the Cleo data, to re-score the lists produced by the translator according to the probability of their word sequences. Only the top-ranked translation was added to the researchers’ Hindi data set.
In another experiment, once Punjabi and her colleagues had assembled a data set of automatically translated utterances, they used the weak, Cleo-based language model to winnow it down, discarding sentences that the model deemed too improbable. With the data that was left, they built a new, much richer language model.
Punjabi and her colleagues evaluated each of these data enrichment techniques separately, so they could measure the contribution that each made to the total error rate reduction of the resulting language model. To test each language model, they integrated it into a complete ASR system, whose performance they compared to that of an ASR system that used a language model trained solely on the Cleo data.
Each modification made a significant difference in its own right. In experiments involving a Hindi data set with 200,000 utterances, re-scoring translation hypotheses, for instance, reduced the ASR system’s error rate by as much as 6.28%, model fine-tuning by as much as 6.84%. But the best-performing language model combined all the modifications, reducing the error rate by 7.86%.
When the researchers reduced the size of the Hindi data set, to simulate the situation in which training data in a new language is particularly hard to come by, the gains were even greater. At 20,000 Hindi utterances, the error rate reduction was 13.18%, at 10,000, 15.65%.
Lifelong learning
In addition to Cho, Kumar, and Campbell, the seven organizers of the Life-Long Learning for Spoken-Language Systems Workshop include Hadrian Glaude, a machine learning scientist, and senior principal scientist Dilek Hakkani-Tür, both of the Alexa AI group.
The workshop, which addresses problems of continual improvement to conversational-AI systems, features invited speakers, including Nancy Chen, a primary investigator at Singapore’s Agency for Science, Technology, and Research (A*STAR), and Alex Waibel, a professor of computer science at Carnegie Mellon University and one of the workshop organizers. The poster session includes six papers, spanning topics from question answering to emotion recognition.