At the 2018 Conference on Empirical Methods in Natural Language Processing (EMNLP), Amazon researchers and their colleagues at the University of Sheffield and Imperial College London will host the first Workshop on Fact Extraction and Verification, which will explore how computer systems can learn to recognize false assertions online.
The workshop’s organizers envision it as the first in a series of annual workshops, which will both catalyze and publicize research on automatic fact verification. The inaugural workshop, on Nov. 1, 2018, features four talks by invited speakers, along with three oral presentations and 11 poster presentations of original research.
The organizers will also announce the final results of the FEVER challenge, in which participating teams used a dataset created by the Amazon and Sheffield researchers to build fact verification systems. (FEVER, the name of the dataset, is an acronym for fact extraction and verification.) The top four finishers will give short talks during the workshop, and the remaining participants will present posters.
Amazon has also sponsored five 1,000-euro grants for workshop attendees, to help cover travel costs and other expenses related to either the workshop or the broader EMNLP conference.
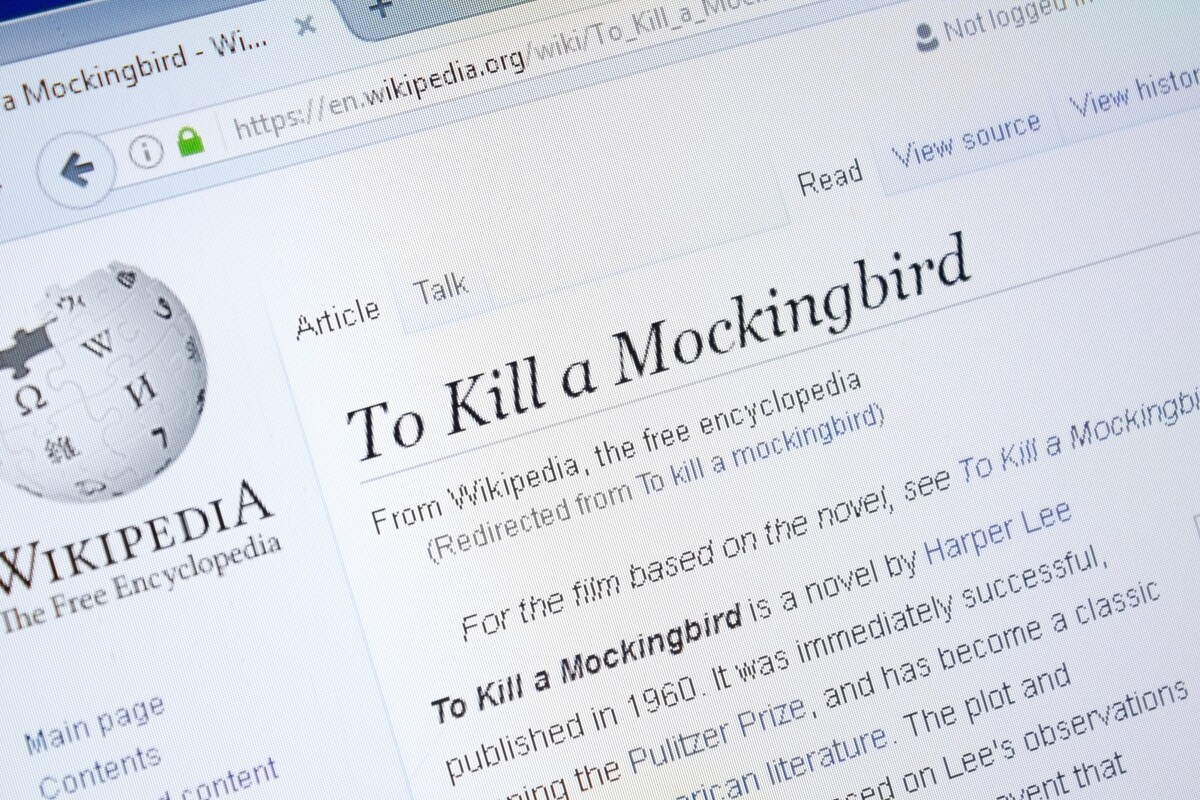
The FEVER dataset comprises 185,000 assertions of fact, together with sentences from Wikipedia entries that either substantiate or refute them. The FEVER challenge participants used the dataset to train machine learning systems to scour Wikipedia for sentences that either validate or invalidate arbitrary claims.
Although the participants’ systems were trained on hand-selected Wikipedia extracts, during testing they were able to pull evidence from anywhere on Wikipedia. Many of the evidentiary sentences they identified were not yet part of the FEVER dataset. Evaluating the systems’ performance requires manually assessing the validity of those sentences.
So far, 22 human annotators have reviewed more than 1,000 such sentences and added them to the FEVER database. Currently, the leading teams are the ones from the University of North Carolina, University College London, and Technical University Darmstadt.
“We are going to keep annotating data up until the workshop,” says Arpit Mittal, a senior machine learning scientist with the Alexa Information Domain group and one of FEVER’s developers. “Then we will announce the final results during the workshop.”
In April, when the Amazon and Sheffield researchers released the FEVER data, they also issued a paper describing a baseline system that they’d trained on the data. Although the top-finishing teams in the contest improved significantly on the baseline system’s performance, “we were very surprised to see that most of the teams had systems that had the same components that we had in our baseline system,” Mittal says.
Those components are (1) a document retrieval module, which attempts to identify relevant Wikipedia articles on the basis of the words contained in the target claim; (2) a sentence selection module, which finds sentences within the retrieved Wikipedia pages that share words with the claim; and (3) a textual-entailment module, which learns which of the selected sentences best predict the final assessment of the claim’s validity.
Most of the variation in the contest entries, Mittal says, occurred in the final component, the textual-entailment module. In that respect, the leading entry, from the University of North Carolina, had a few unique features. For example, it used as an input information from WordNet, a huge database that catalogues semantic similarities between words, which may have helped it identify evidentiary sentences whose meanings are related to the target claims’ but use a somewhat different vocabulary. It also used the confidence scores of the second component — the sentence selector — as an input to the third.
The textual-entailment module of the second-leading system, from University College London, had some distinguishing traits, too. All the other entries treated the evidence for a given claim as a single block, no matter how many sentences it comprised. The UCL system, by contrast, used each sentence in the block to predict the claim’s validity, then pooled the results.
But, Mittal observes, teams that used very similar techniques often had widely divergent results. “The teams that managed to combine different components together and tune them well, so that there was a better synergy between components, got the best scores,” he says.