The rapid spread of COVID-19 demonstrates the dire need for quick and effective drug discovery. Drug repurposing is a drug discovery paradigm that uses existing drugs for new therapeutic indications. It has the advantages of significantly reducing time and cost relative to de novo drug discovery. Drug repurposing with knowledge graphs presents a promising strategy for COVID-19 treatment.
Knowledge graphs describe known relationships between real-world entities and allow for the discovery of novel relationships. They’re an ideal tool for drug repurposing, which relies on identifying novel interactions among biological entities such as proteins and compounds.
Link prediction is the process of expanding the information stored in knowledge graphs by probabilistically inferring missing links (or edges) between entities in the existing graph structure. It can be used to infer direct links between drugs and diseases or lower-level links between drugs and the cellular products associated with disease — for instance, between a compound and the protein it inhibits.
To accelerate research on drug repurposing, a team of AWS researchers and our collaborators from the University of Minnesota, the Ohio State University, and Hunan University have created and open-sourced the Drug Repurposing Knowledge Graph (DRKG), along with a set of machine learning tools that can be used to prioritize drugs for repurposing studies.
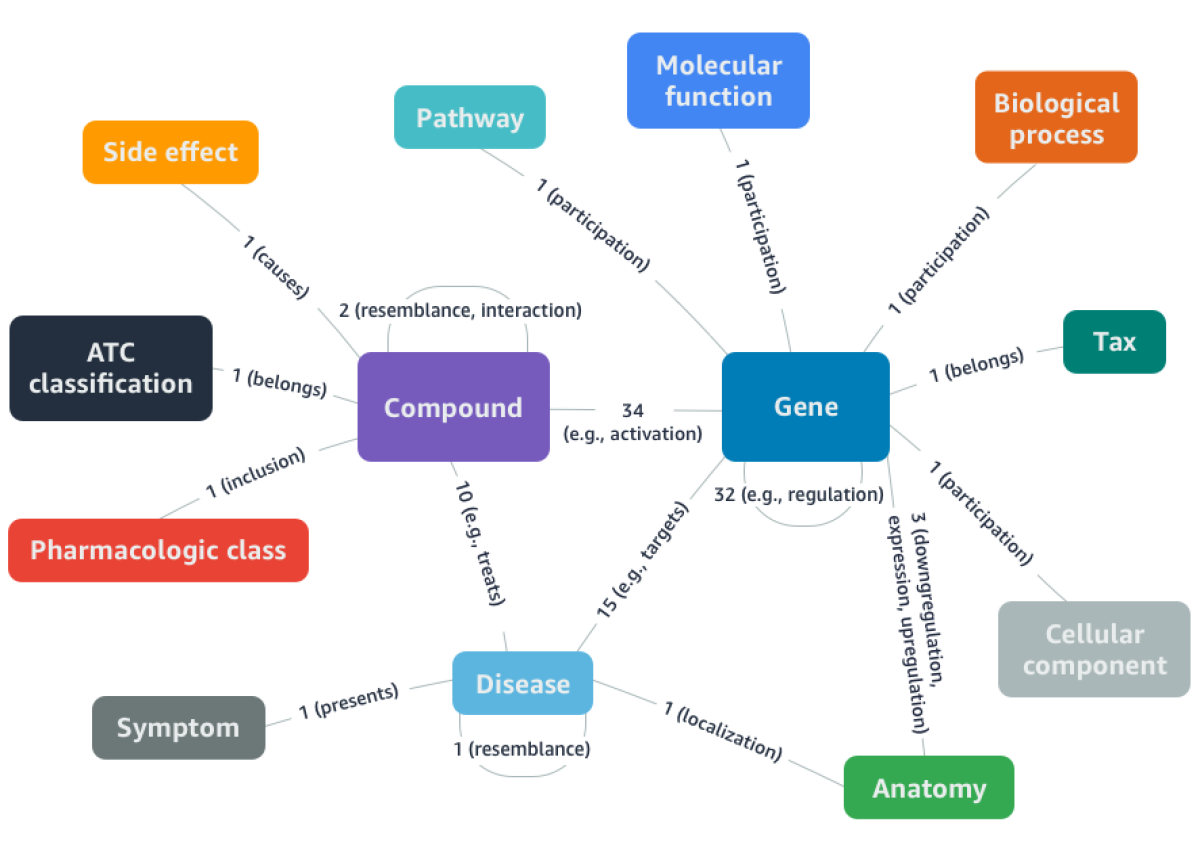
In experiments, we used machine learning methods to search DRKG for drugs with the potential to treat COVID-19. Of the 41 drugs our analysis identified, 11 are or have been under clinical trials for COVID-19.
DRKG is a comprehensive biological knowledge graph that relates human genes, chemical compounds, biological processes, drug side effects, diseases, and symptoms. It curates and normalizes data from six publicly available databases as well as information from recent publications related to COVID-19.
DRKG includes nearly 100,000 entities of more than a dozen types and nearly 6,000,000 relationships of more than 100 types. It captures interactions between entities that are related to the genetic signature of COVID-19 or to components of existing drugs and viruses.
The associated machine learning tools use state-of-the-art deep-graph-learning methods (DGL-KE) that take advantage of distributed graph operations (from popular deep-learning libraries such as PyTorch and MXNet) to predict the likelihood that a drug can treat a disease or bind to a protein associated with the disease.
When tested against the human proteins associated with COVID-19, these tools assigned high probabilities to many of the COVID-19 drug candidates currently in clinical trials. Both DRKG and the machine learning tools are publicly available on GitHub. This should help make computational drug repurposing for COVID-19 and other diseases (e.g., Alzheimer’s disease) more efficient and effective.
The AWS team that helped develop DRKG includes Vassilis Ioannidis, Xiang Song, Saurav Manchanda, Mufei Li, Xiaoqin Pan, Da Zheng, and George Karypis.