In the swiftly evolving domain of large language models (LLMs), the accurate evaluation of retrieval-augmented-generation (RAG) models is paramount. In this blog, we introduce a pioneering methodology that employs an automated exam generation process, enhanced by item response theory (IRT), to evaluate the factual accuracy of RAG models on specific tasks. Our approach is not only robust and interpretable but also cost efficient, strategically identifying model strengths and refining exams to optimize their evaluative utility. We describe our methodology in a paper we will present in July at the 2024 International Conference on Machine Learning (ICML).
Exam generation process
RAG is a method for handling natural-language queries by retrieving relevant documents and using text from them to seed the response generated by an LLM. The expectation is that factual assertions from reliable documents will curb the LLM’s tendency to “hallucinate”, or generate reasonable-sounding but false sentences.
To evaluate a RAG model on a particular task, we use an LLM to generate multiple-choice questions from a task-specific knowledge corpus. Our method is agnostic to the retriever and generative model used in both the RAG system and the exam generation task.
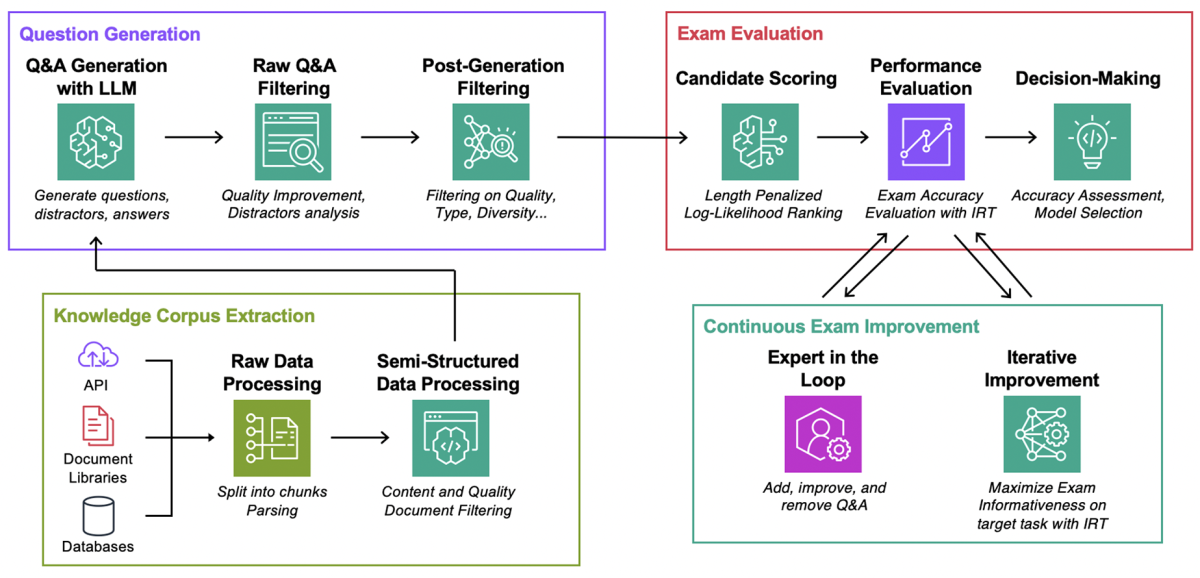
Our approach has two steps. For each document in the knowledge corpus, we use an LLM and several prompt-engineering strategies to create candidate questions. Then we use several natural-language-processing filters to remove low-quality questions along various axes, such as length, incorrectness, and self-containment.
We note an interesting asymmetry: given a document corpus, it is relatively easy for an LLM to generate a question and the correct answer, as the content of both is contained in the prompt. However, it is considerably more difficult to create high-quality incorrect answers, commonly referred to as discriminators.
To filter out degenerate questions, we use the Jaccard similarity coefficient and embedding-based similarity metrics.
Here is the prompt that we used for exam generation:
Human: Here is some documentation from {task_domain}: {documentation}.\n From this generate a difficult multi-form question for an exam. It should have 4 candidates, 1 correct answer, and explanations. Syntax should be Question: {question}\n A){candidate A}\n B){candidate B}\n C){candidate C}\n D){candidate D} Correct Answer: {correct answer}\n ### Assistant:"
In our research, we analyzed several RAG pipeline variants, including closed-book (no knowledge from the document corpus is provided to the LLM), oracle (the exam taker has access to the specific document used to generate the question-and-answer pair, in addition to the question itself and all possible candidate answers), and classical retrieval models such as MultiQA embeddings, Siamese network embeddings, and BM25. Our evaluations also extended to different scales of language models, from 7 billion parameters to 70 billion, to understand the impact of model scale on performance.
To demonstrate the practical utility of this methodology, we deployed it across a wide range of domains. These include Amazon Web Services (AWS) DevOps, where troubleshooting guides for cloud-based services tests the models' operational effectiveness; arXiv abstracts, which challenge the models' ability to parse and generate insights from dense scientific texts; StackExchange questions, which probe the models' responsiveness and accuracy; and SEC filings, where the complexity of financial reporting tests the models’ capacity to extract nuanced information from structured corporate documents. This multi-domain approach not only enhances the robustness of our evaluations but also ensures that our models are versatile and reliable across various real-world applications.
Evaluating the exam generation model
The following figure shows granular results of our evaluation method for the task of AWS DevOps troubleshooting. We report accuracy for different retrieval approaches and retriever sizes, on a percentage scale. Labels on the diameter show the AWS resources we’re using. Colors correspond to different retrieval approaches (Oracle, DPRV2, MultiQA, ClosedBook), and solid and broken lines correspond to different base LLM sizes (7B, 13B, and 70B). For instance, we observe that a small model such as Mistral-7B with MultiQA embeddings has an accuracy of around 80% for the AWS resource Relational Database Service (RDS).
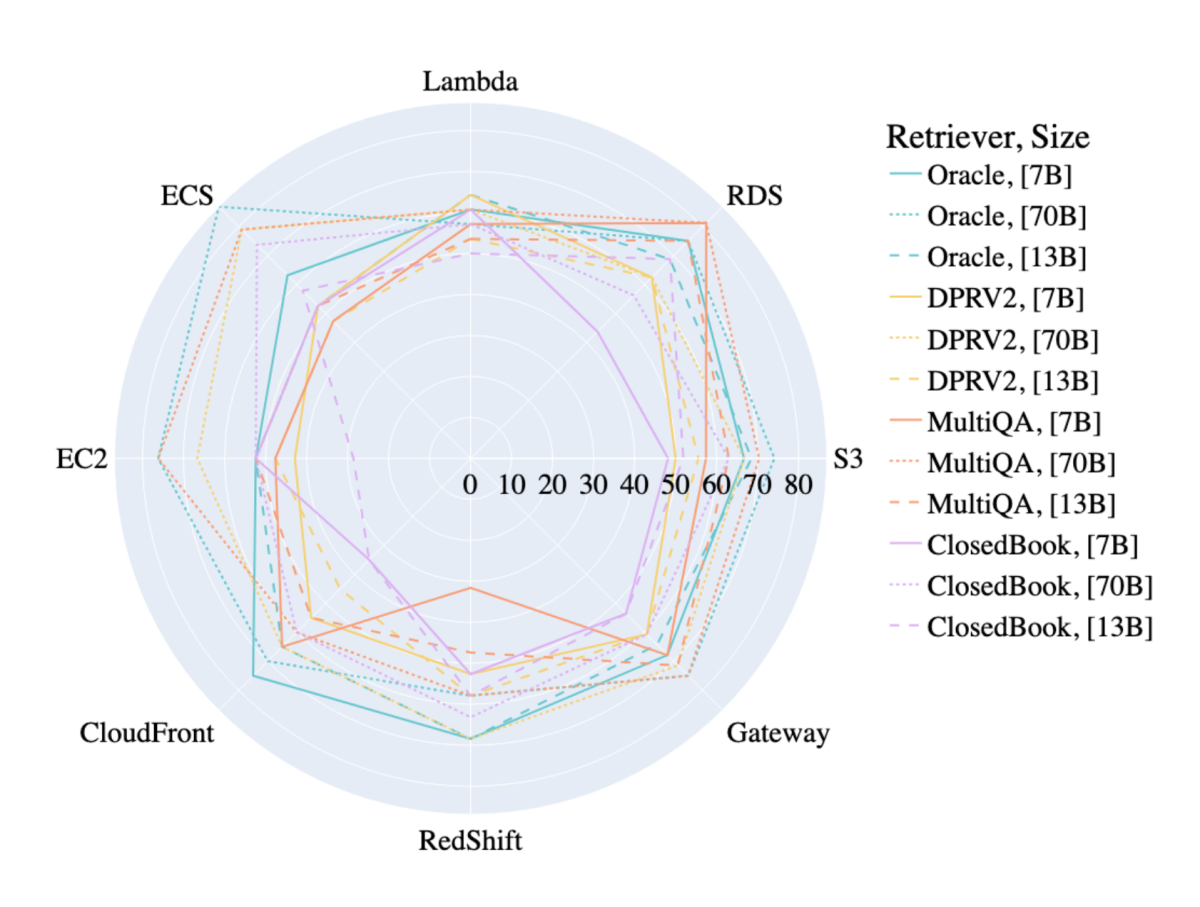
Our experiments yielded four key findings. First, there’s no one-size-fits-all solution; the optimal choice of retrieval method, and to a lesser extent LLM, is typically task dependent. For example, in tasks such as SEC filings and arXiv abstracts, BM25 outperforms MultiQA and Siamese network embeddings, indicating that sparse retrieval is generally more effective than dense retrieval. This could be because such tasks often contain easily identifiable terms (e.g., AWS service names in AWS DevOps) that can be retrieved with keyword search, while other tasks, such as StackExchange, mostly contain common words.
Second, the right choice of retrieval method can lead to greater performance improvements than simply using larger LLMs. For instance, in SEC filings, we observed a greater performance gain from switching from Siamese network embeddings to DPRV2 than from switching to larger LLMs.
Third, for tasks involving closed-source knowledge, the accuracy bottleneck is typically the LLM rather than the retrieval method. Finally, a poorly aligned retriever component can result in worse accuracy than having no retrieval at all.
Exam enhancements through item response theory
Integrating item response theory (IRT) into our process has significantly improved the quality of the exams. IRT models the likelihood of a correct response based on characteristics of a question and the capabilities of a model. It uses three factors — difficulty, discrimination, and guessing chance — to create exams that more accurately reflect and predict model performance.
IRT posits that a model’s probability of correctly answering a question is correlated with a latent variable known as ability, and it provides a method for estimating the value of that variable. As such, it offers a way to quantify a model’s ability level.
Our process begins with an initial exam assessment, identifying and removing questions that contribute minimally to discriminative insights. The exam is then refined iteratively, based on updated IRT parameters, which helps it accurately gauge nuanced model behaviors.
By continuously analyzing and adjusting exams based on IRT parameters, we have seen substantial improvements in the exams’ ability to discriminate among models. For instance, we use Fisher information to quantify the informativeness of exam questions. Fisher information measures the amount of information that an observable random variable provides about an unknown parameter, offering a way to gauge the precision of statistical estimators in parameter estimation theory.
During iterative improvements for the arXiv task, the Fisher information function consistently showed progress, marking a considerable enhancement of the exams' capacity to differentiate model capabilities. This iterative process ensures that each new version of the exam is more informative than the last and effectively evaluates the RAG model’s abilities.
Evaluating the generated exams
To further enhance the assessment of RAG models, we categorize exam questions using both semantic analysis and Bloom’s revised taxonomy, devised by the University of Chicago psychologist Benjamin Bloom. Bloom’s taxonomy helps classify questions by cognitive complexity — from basic recall to analytical tasks — enabling structured evaluation of model capabilities.
Different levels in Bloom's taxonomy differentiate between the knowledge dimension (factual, conceptual, procedural, and meta-cognitive) and the cognitive-process dimension (remember, understand, apply, analyze, evaluate, and create). Additionally, we classify questions semantically by identifying keywords like “what” and “which.” These additional classifications allow us to assess how well models perform at different ability levels.
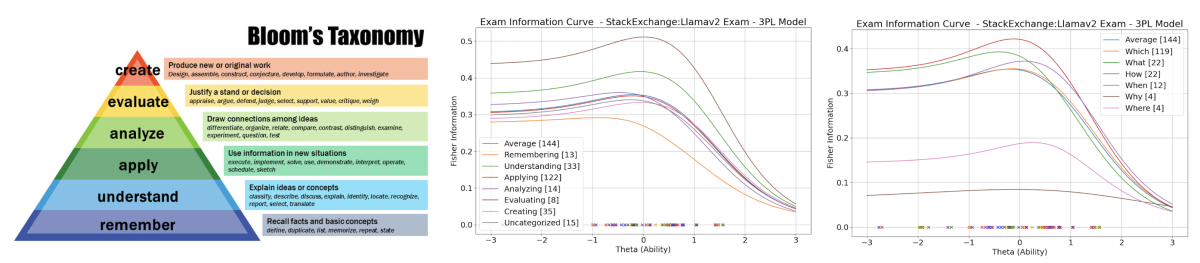
The above two figures present the average Fisher information value for each Bloom category (left) and semantic category (right) for the StackExchange task. For this specific task, we observe that “evaluating” and “understanding” are the most discriminate dimensions in Bloom’s taxonomy across different ability levels, while “remembering” is the least discriminatory.
On the semantic categories, we observe that “what” and “which” were the most discriminatory terms for lower ability levels, and “when” discriminated more at higher ability levels. One interpretation is that “what” and “how” questions tend to be more factual and syntax-based in the StackExchange domain, so at lower ability levels, RAG struggles more with these genres of questions.
The following figure illustrates the maximization process for the arXiv task as the exam and IRT estimation evolve. We show the results for three incremental steps. We observe a 0.05 increase in Fisher information even with a single iteration. This progress reaches a 0.1 increase in the subsequent steps.
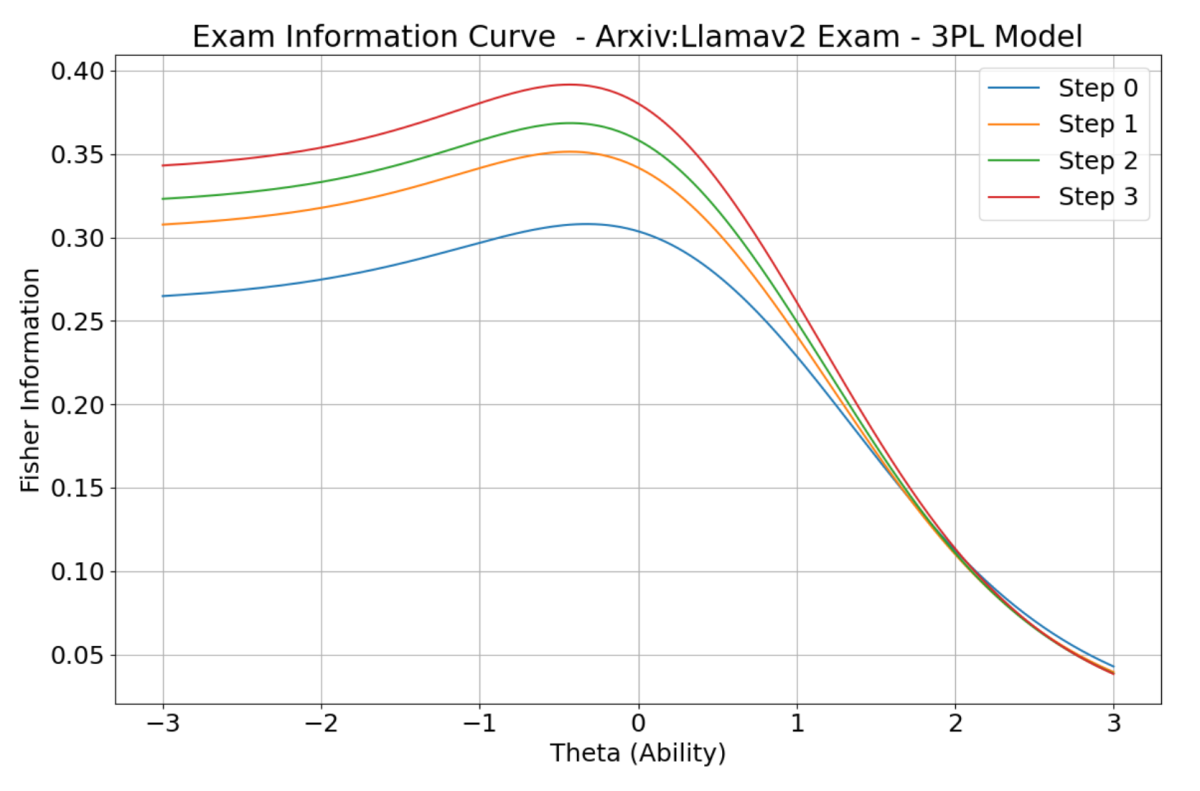
To expand our approach beyond Q&A applications, our future research will focus on domains such as summarization, translation, and sentiment analysis. We are also addressing the complex task of meta-evaluation, comparing and refining our evaluation methods to account for the multidimensional nature of LLM performance. Additionally, we will continuously update our methodologies to accommodate the rapid evolution of LLM technology, ensuring robust and comprehensive assessment of emerging models.
Acknowledgments: Laurent Callot