Aleix M. Martinez, a senior principal scientist with Amazon’s retail division, first attended the Conference on Computer Vision and Pattern Recognition (CVPR) — the premier conference in the field of computer vision — in the late 1990s, when he was a graduate student. “Golly, I've been in almost all of them since then,” he says.
In that span, he’s served multiple times as an area chair, and in 2014, he was one of the conference organizers, when the conference came to Columbus, Ohio, home of the Ohio State University, where Martinez maintains a faculty appointment.
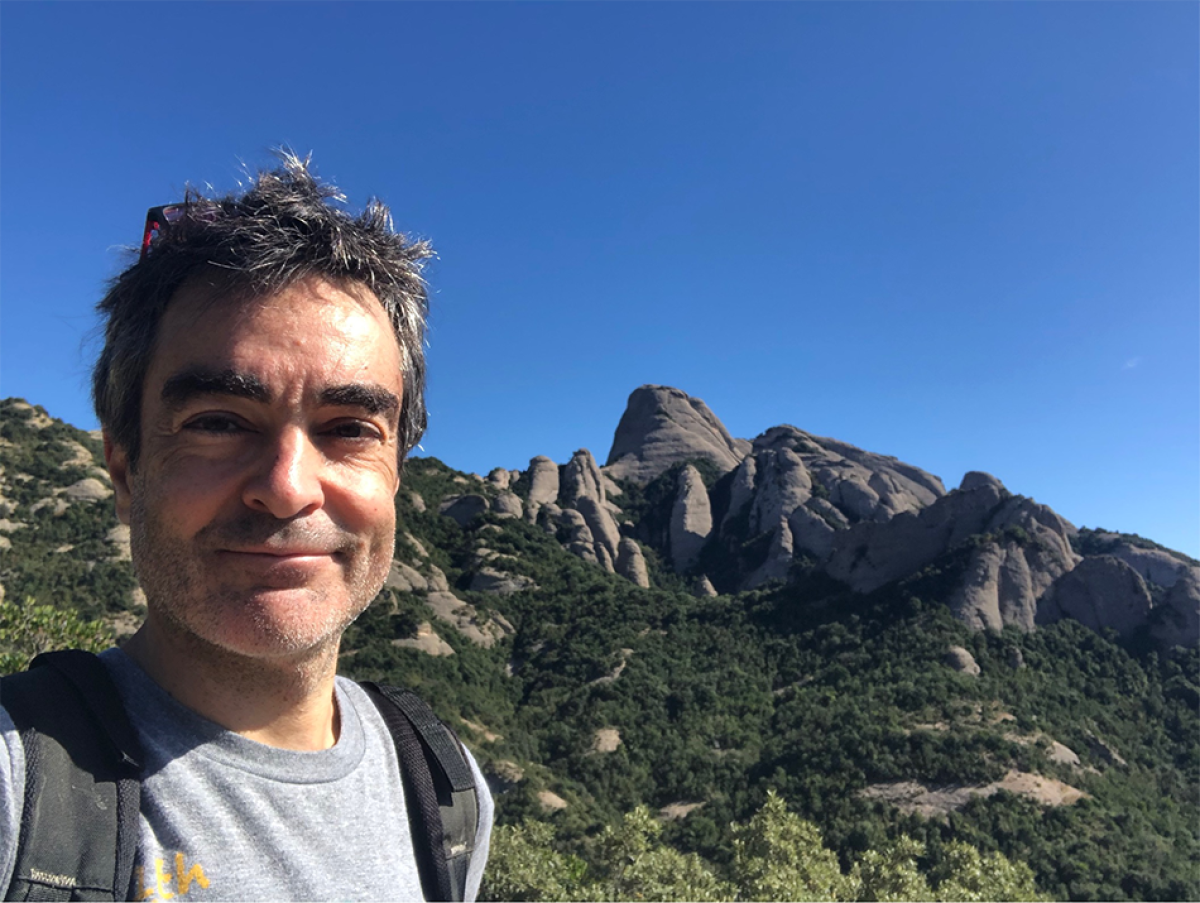
He’s also seen deep learning revolutionize computer vision, to the point that many of the problems that defined the field when he first attended the conference have virtually been solved. But, Martinez says, they’ve been succeeded by problems that are even richer and more complex.
“As a professor, I did a lot of work in computer vision and machine learning and also in cognitive science,” Martinez says. “And the reason is, I personally do not think that we can solve all these complex problems if we don't understand the brain.
“For example, one of the things that I worked on for many years is how to interpret nonverbal signals, including face and body motion. There was this belief that people would communicate emotion categories through their facial expressions. And we demonstrated over many, many years in our research group that that's not the case.
“I had a paper where I had an example where you could see just the face of a guy who was completely red, screaming like crazy. You show it to people, and they would say that this person is really angry at something — a very negative emotion. But when you show the actual picture, it was a soccer player with arms outstretched, running, screaming like mad, and you could see in the background the goalkeeper on the ground with the ball in sight. When you see it in that context, you understand that's not an angry person; that's a very happy person who is celebrating a goal.
“This is the complexity of human cognition that with the computer vision and machine learning methods that we have now cannot be achieved. You are not including all this knowledge, all these concepts. You need to understand what soccer is and how it’s played. You need to understand that there are two teams, and that if you're running away from the other team’s goalkeeper, and the goalkeeper is disappointed, you’re celebrating. We take these things for granted, but they are very complex.
“One of the other variables that we showed is important is blood flow to the face. When you experience an emotion internally, your body releases what's called peptides, including hormones like testosterone and cortisol. And that actually changes the blood flow and blood composition of your body. And because the face is suffused with a huge number of blood vessels, when you experience an emotion, your face pulsates in color. And we actually showed that humans use that signal to interpret what you're experiencing.
“Until we published this in the Proceedings of the National Academy of Sciences, no one even knew that signal existed. We use it all the time, yet we don't know that we use it. How many of those unknowns are out there about what we do to interpret the world? We don't even know how many unknowns.
“People are talking about, ‘When is machine learning going to achieve human intelligence?’ Well, it's an irrelevant question. For now, we cannot attain human-level intelligence, because we do not know what human intelligence is. Cognitive scientists, neuroscientists have written 500-, 700-page books trying to explain what human intelligence is. That's not the definition. That's a 700-page book.
“I'd like to see more help from the CVPR community to understand what human intelligence is and more work toward trying to imitate those things — including reasoning.”
Visual shopping
At Amazon, Martinez leads a team that uses computer vision to make shopping more convenient and enjoyable for customers of the Amazon store. One of the team’s projects, for instance, is “shoppable images”, images of rooms in which clicking on an object will pull up information about related products. Computer vision algorithms identify products that resemble those in the images.
“The idea is that, similar to when you go to a physical store, you walk through a set of showrooms that are decorated with a number of products, and when you find something that you like, you can click on the specific products here and find things that are similar,” Martinez explains.
Shoppable images launched in 2020, and this year, Martinez’s team extended the same functionality to images on product detail pages, enabling customers to, say, click on a lamp that’s just décor for a product shot of an armchair.
Currently, Martinez says, the team is working on algorithms that combine computer vision and specifications in the product catalogue to automatically overlay images with directional arrows indicating product dimensions. They’re also exploring the use of generative adversarial networks (GANs) to synthesize virtual showrooms, to expand the amount of shoppable content available to customers.
“Generative models are really good for generating single-object images, like a human face or a cat, a dog, a car,” Martinez says. “What I'm interested in is, ‘Can we generate realistic scenes, with multiple objects, multiple activities?’ Can you draw people interacting with one another meaningfully, and it looks realistic? Can you describe not only a noun in a dictionary — a product in our case — but can you describe actions, meaning the verbs of the dictionary? Can you edit those images to create videos that showcase viewpoint variation or illumination changes? Those are things that the scientific community has not fully addressed yet. And I think we are mature enough to start thinking about them and potentially addressing some of them.”