The Transformer is a neural-network architecture that has proven extremely useful for natural-language-processing tasks because it can recognize long-range dependencies. It could, for instance, recognize that in a sentence that includes the word “rented”, the word “flat” is more likely to mean “apartment” than it would be otherwise, even if “rented” is the second word in the sentence and “flat” the 10th.
In its most basic form, the Transformer is indifferent to word order. It can recognize the relationship between “rented” and “flat”, but it doesn’t care which comes first.
Word order, however, can make a big difference to meaning. Consider, for instance, the sentences “We rented a small but clean, well-equipped two-bed flat” and “We rented a small but clean, well-equipped flat-bed truck”.
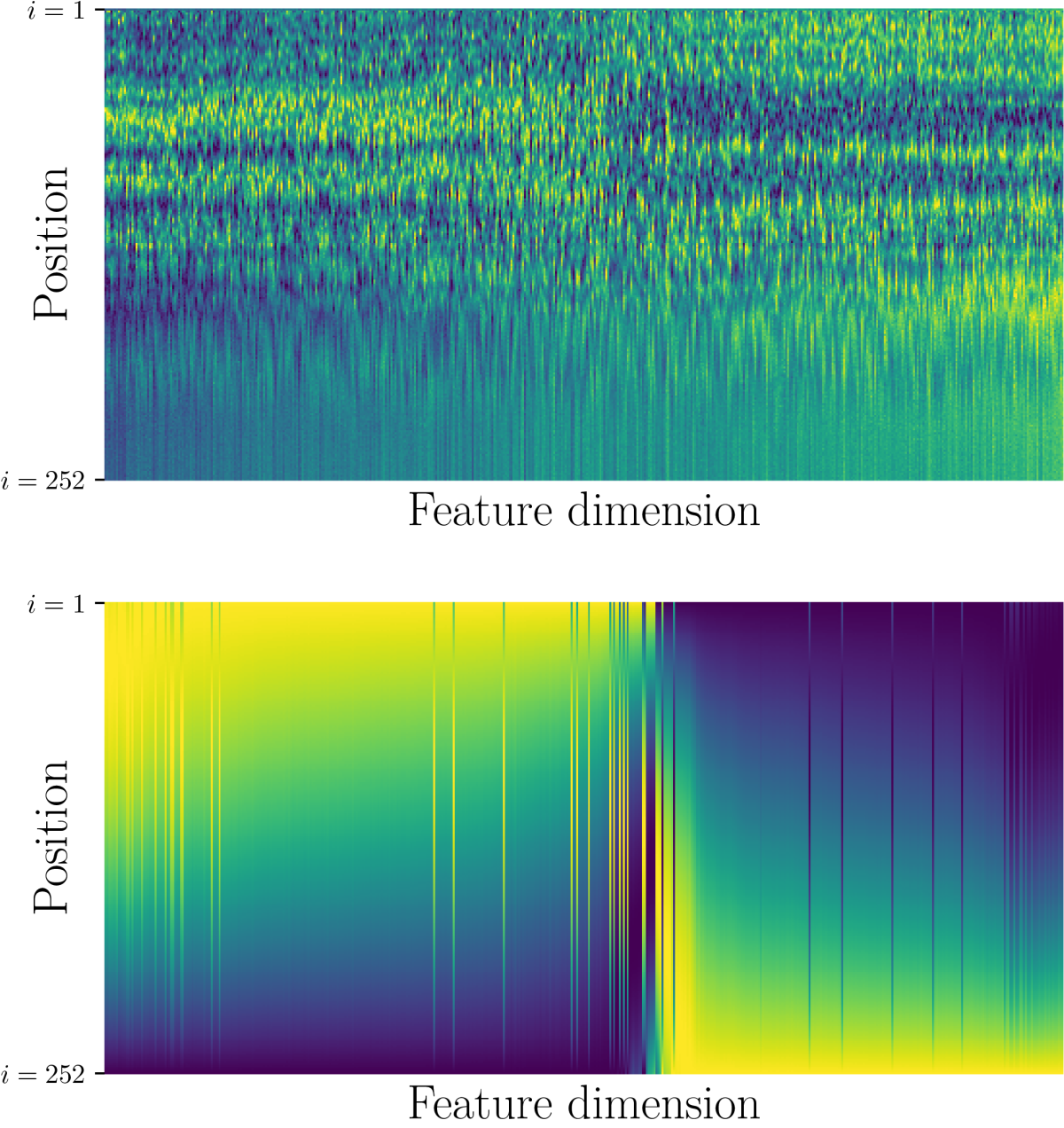
Starting with the paper that introduced the Transformer, researchers have proposed a series of position encoders that inject word-order information into the Transformer model. But last week, at the International Conference on Machine Learning, we presented a new position encoder that enables better performance than its predecessors on a range of natural-language-processing (NLP) tasks.
We designed our position encoder so that it can be integrated into existing Transformer models, conferring its benefits to NLP systems that already have been trained extensively on large data sets.
Before the Transformer was introduced in 2017, the most popular architecture for NLP was the long short-term memory, or LSTM. LSTMs process sequenced inputs in order, and each output reflects both the inputs and the outputs that preceded it.
LSTMs are very good at inferring local relationships — a word’s relationships, both syntactic and semantic, with the two or three words that immediately precede it — but they’re not as good at modeling long-range dependencies. That’s where the Transformer excels.
Position encodings are an attempt to achieve the best of both worlds: an awareness of long-range dependencies and a sensitivity to local word order. The ideal position encoding should have three properties:
- It should be able to handle sequences of arbitrary length; that is, it shouldn’t be locked in to some maximum sequence length.
- It should be learnable from training data; different encodings may work better for different tasks.
- It should be efficient; adding position encoding shouldn’t unreasonably inflate the size of the neural model.
Past position encoding schemes have met at best two of these criteria. For instance, the original Transformer paper proposed an encoding based on a family of sinusoidal functions; that encoding remains popular, but it is not learnable.
Our scheme, which we call FLOATER, is the first to meet all three criteria.
The naïve way to encode position would be simply to assign successive numbers to successive words in an input sequence. But this has drawbacks in a machine learning context. If at runtime the model sees a sequence of a length it did not encounter during training, it will be flummoxed about how to proceed.
So most position encoding schemes instead use position vectors, which carry information that can be used to deduce the relative positions of two inputs. If those schemes are fully learnable, however, they tend to inflate the model size; or, to keep model inflation under control, they limit the distances across which relative position can be compared.
Functional approach
Instead of learning to directly compute a position vector from each word in an input sequence, FLOATER learns a function that computes each word’s position vector from that of the word that preceded it.
Learning a general function rather than direct mappings makes FLOATER much more space efficient than other learnable encoding schemes. But a general function can also be applied to any word in a sequence, regardless of its position, so FLOATER is indifferent to sequence length.
Any given manually engineered position function — such as the sinusoidal functions proposed in the original Transformer paper — can be thought of as a special case of the general FLOATER function. So in a pretrained network, we can simply substitute FLOATER for any such function and then fine-tune it on a small set of training data.
Past work on position encoding has shown that re-encoding position information at every layer of a Transformer network improves performance on NLP tasks. If we allowed FLOATER to learn a different function for every layer, the model size would again begin to inflate.
So instead, we learn a single function that is applied at every layer. This results in different position encodings at each layer, however, because the inputs are different. Our experiments indicate that this approach strikes a good balance between model size and performance improvements.
In one set of experiments, we compared our position encoder to its two leading predecessors on four different machine translation tasks and found that it delivered the best results across the board.
In another set of experiments, we added our position encoder to Transformer models that had previously been trained on three different language-understanding and question-answering tasks.
Of 23 distinct tasks, the addition of our position encoder improved performance on 21. The two on which its performance fell slightly short were low-data versions of tasks on which, with larger sets of training data, it improved performance.