As general chair of this year’s ACM Conference on Knowledge Discovery and Data Mining (KDD), Huzefa Rangwala, a senior manager at the Amazon Machine Learning Solutions Lab, has a broad view of the topics under discussion there. Two of the most prominent, he says, are graph neural networks and fairness in AI.
Graphs are data representations that can encode relationships between different data items, and graph neural networks are machine learning models that are useful for knowledge discovery because they can be used to infer graph structures.
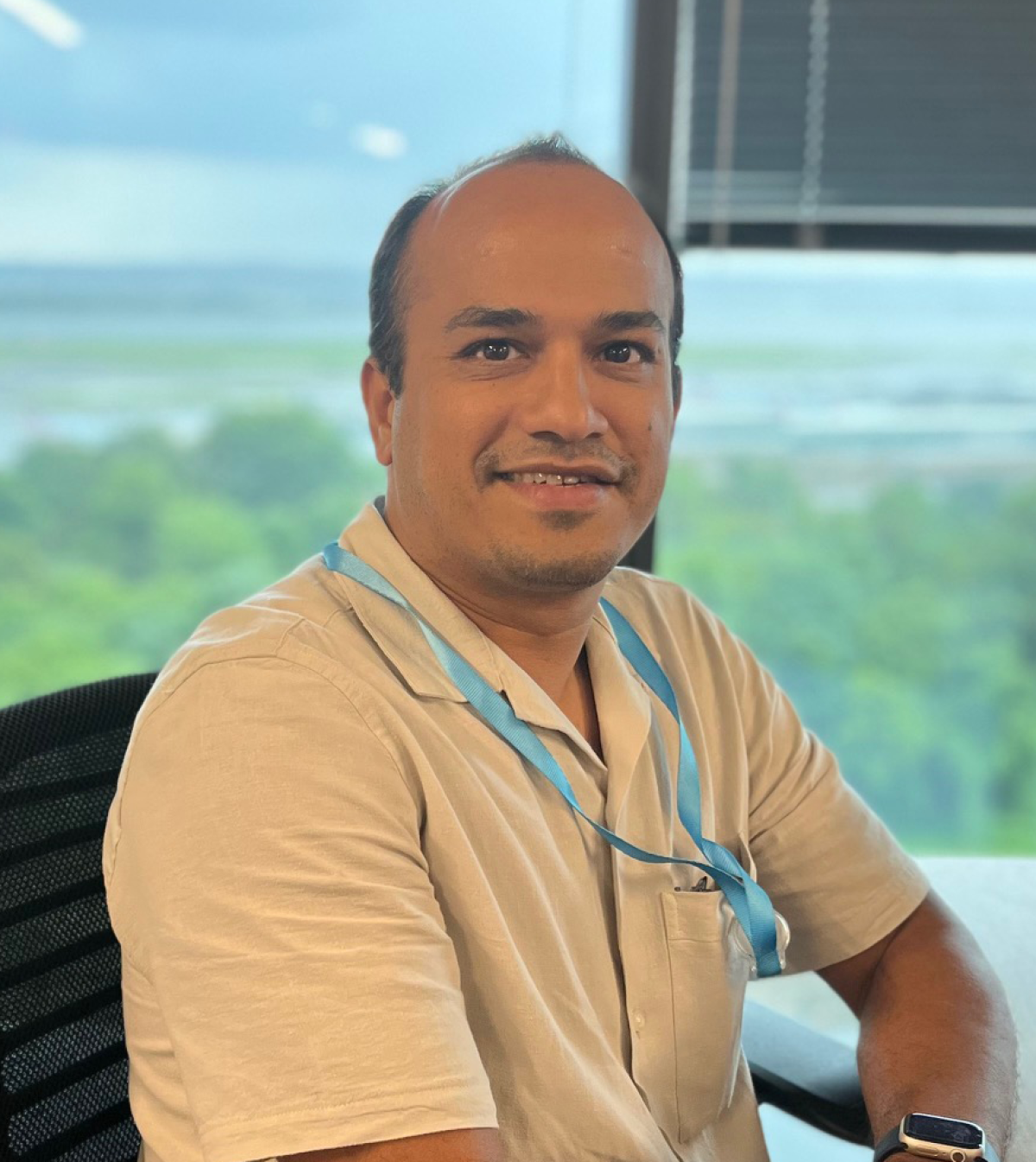
“Our world is connected in lots of ways, so you'll see graph neural networks find applications in lots of different domains, all the way from social networks and transportation networks to knowledge graphs and drug discovery,” Rangwala says.
The Amazon Machine Learning Solutions Lab brings the expertise of Amazon scientists and the resources of Amazon Web Services to bear on customers’ machine learning problems. Before joining Amazon, Rangwala was a professor of computer science at George Mason University, where he focused on interdisciplinary applications of machine learning — particularly, in biomedicine, learning sciences, and social sciences. Similarly, his team at the ML Solutions Lab works with customers across industries, including health care and life sciences, sports, and manufacturing.
“We’re using graph neural networks to represent macromolecules like proteins and their interacting partners,” Rangwala says. “So we’re using graph neural networks to essentially accelerate drug discovery or to find new biotherapeutics. And we’ve already deployed this approach with one of our customers, Janssen Pharmaceuticals.
“One of the outstanding questions is how you take the input from, let’s say, proteins and transform them into a representation for these graph structures. That’s step one: how do you engineer this in a robust manner, so you get good results.
“Some of the other open challenges are similar to the challenges that you see in deep-learning approaches: how do you ensure that the end results that you're getting are explainable and robust? At the end of the day, the end user might not be happy with just the prediction score. They might want to know why the predictions make sense.
“At KDD, there are several ideas being presented on how to scale, how to be efficient at running these and training these, as the dataset gets larger, the number of interactions get larger, and hence the representation gets larger. There are approaches to parallelize this, and there are also approaches to use efficient data structures. And then there are approaches to developing new formulations and computer architectures that can that can work nicely on these structures.
“But the really exciting thing for me is that we are seeing so many uses — proteins, molecules, information extraction, recommendations, anomaly detection — that all lead to improved scientific and business outcomes. These are some of the challenges and excitement around these techniques.”
Theory into practice
Indeed, Rangwala says, the breadth of the applications on display at KDD is, for him, one of the conference’s chief points of appeal.
I really like applied science. I'm not tied to one particular method or one particular domain. I'm most interested in how we can use these computing and machine learning techniques to solve challenging problems.
“I'm mostly excited about KDD as a conference because it not only has innovations on the core data science methodologies, but many researchers are focused on how to use them,” Rangwala says. “How do you go from theory to practice? How do you translate machine learning research into the hands of end users?
“There's a lot of interdisciplinary work, first of all, even on the research track. And for many years KDD has had an applied-data-science track, so you not only get to see cutting-edge research, but you also got to see translational research, where you get to see how these things are applied.
“This suits my background, because I really like applied science. I'm not tied to one particular method or one particular domain. I'm most interested in how we can use these computing and machine learning techniques to solve challenging problems in different domains, be it physics, biology, chemistry, all the way to social sciences.”
Trustworthy computing
In knowledge discovery, as in many other fields related to machine learning, fairness has become a prominent research topic in recent years, Rangwala explains.
“Trustworthiness is crucial for the adoption of AI technologies and realizing their potential gains to the society. Being trustworthy means they need to be fair, they need to be explainable, and they also need to be reproducible.
“Now, there's a whole argument around this: is it that the data is biased, or is it the society that's biased? I think the field overall is cognizant of these issues, and they are asking the right questions — for example, building auditing approaches or mitigation approaches. Most importantly, they’re empowering the different stakeholders — developers, decision makers, and end users — to complement the developed solutions and ensure that algorithms are trustworthy.
“At KDD, there is a special day that is devoted to this topic, called Trustworthy AI Day. Also, if you look at the research track, there are lots of sessions on these topics.
“I also want to highlight the Women in KDD event. It's really exciting because it’s the first time it’s in-person. Judith Spitz, who founded an organization called Break Through Tech, is co-leading the event with Johannes Gehrke at Microsoft Research, and there is a plan to have power lunches and also to hear panelists talk about career journeys, especially for women and non-binary individuals in KDD. It's something that I'm very passionate about — how to have a more inclusive community.”