A central task of natural-language-understanding systems, like the ones that power Alexa, is domain classification, or determining the general subject of a user’s utterances. Voice services must make finer-grained determinations, too, such as the particular actions that a customer wants executed. But domain classification makes those determinations much more efficient, by narrowing the range of possible interpretations.
Sometimes, though, an Alexa customer might say something that doesn’t fit into any domain. It may be an honest request for a service that doesn’t exist yet, or it might be a case of the customer’s thinking out loud: “Oh wait, that’s not what I wanted.”
If a natural-language-understanding (NLU) system tries to assign a domain to an out-of-domain utterance, the result is likely to be a nonsensical response. Worse, if the NLU system is tracking the conversation, so that it can use contextual information to improve performance, the interpolation of an irrelevant domain can disrupt its sequence of inferences. Getting back on track can be both time consuming and, for the user, annoying.
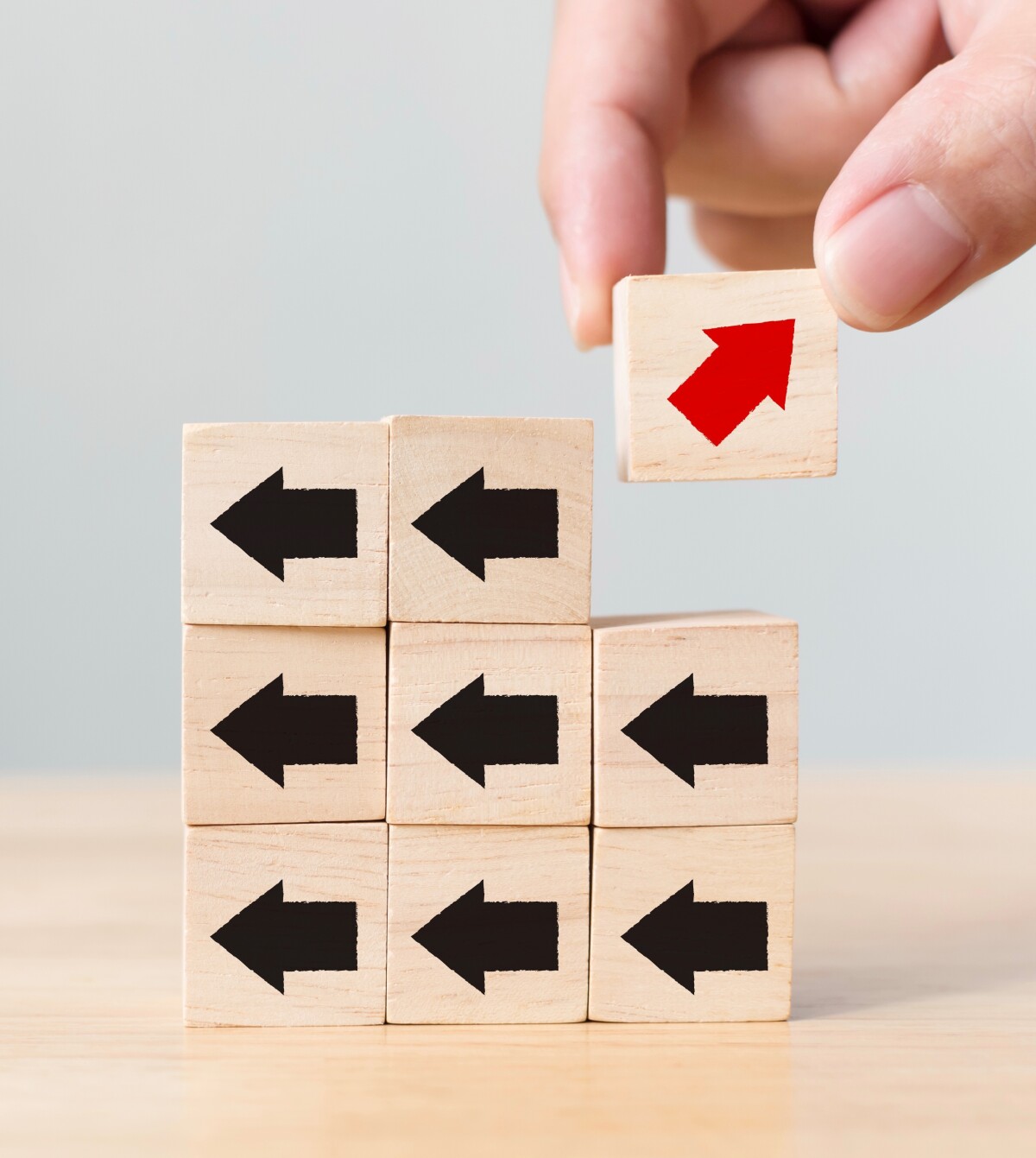
One possible solution is to train a second classifier that sits on top of the domain classifier and just tries to recognize out-of-domain utterances. But this looks like an intrinsically inefficient arrangement. Data features that help a domain classifier recognize utterances that fall within a particular domain are also likely to help an out-of-domain classifier recognize utterances that fall outside it.
In a paper we’re presenting at this year’s Interspeech, my colleague Joo-Kyung Kim and I describe a neural network that we trained simultaneously to recognize in-domain and out-of-domain utterances. By using a training mechanism that iteratively attempts to optimize the trade-off between those two goals, we significantly improve on the performance of a system that features a separately trained domain classifier and out-of-domain classifier.
For purposes of comparison, we set a series of performance targets for out-of-domain (OOD) classification, which both our system and the baseline system had to meet. For each OOD target, we then measured the accuracy of domain classification. On average, our system improved domain classification accuracy by about 6% for a given OOD target.
As inputs to our system, we use both word-level and character-level information. At the word level, we use a standard set of “embeddings,” which represent words as points in a 100-dimensional space, such that words with similar meanings are grouped together. We also feed the words’ constituent characters to a network that, during training, learns its own character-level embeddings, which identify substrings of characters useful for predictive purposes.
The character embeddings for each word in the input pass to a bidirectional long short-term memory (bi-LSTM) network. LSTM networks are common in natural-language processing because they factor in the order in which data are received, which is useful in analyzing both strings of characters and strings of words. Bi-LSTM models consider data sequences both forward and backward.
For each word of the input, the bi-LSTM layers produce a single vector summarizing the useful features identified by the individual character embeddings. Together with the corresponding word embeddings, those summary vectors then pass to another bi-LSTM network, which learns a similar summary of the entire input utterance. That summary, in turn, is the input to both a domain classifier and an OOD classifier.
All of these components are trained in concert, so the network learns character embeddings and summarization procedures that are tailored to the joint tasks of domain classification and OOD classification.
During training, our network, like all machine learning systems, attempted to minimize the error rate of its classifications. But of course, it was performing two classification tasks at once, so a central design question was how much weight to give each in the cumulative error metric.
We resolved this question by giving the network a target rate for false acceptance of out-of-domain utterances. In our experiments, that rate ranged from 1% to 6%. But for each target rate, we trained and retrained the network, adjusting the relative weight of the two error metrics each time, until it met the target rate precisely.
This often meant reducing the weight of the OOD error metric if the false acceptance rate got too low. That is, from one iteration to the next, we would often let the performance of the OOD detector suffer, if it meant a possible increase in the accuracy of the domain classifier. For each target false-acceptance rate, we retrained the network 50 times.
We used two different sets of data to train the system. One consisted of utterances that had been assigned to one of 21 domains; the other consisted of utterances that had been assigned to one of 1,500 frequently used Alexa skills.
When we compared our system’s performance to that of a system that used a separate domain classifier and OOD detector, we fixed the target false-acceptance rate and assessed performance according to domain classification accuracy.
The most dramatic results came when we trained the systems on the 21-domain data set, with a false-acceptance rate of 5%. There, the existing system had a domain classification accuracy of 83.7%, while ours had an accuracy of 90.4%. But in all cases, our system showed improvement over the existing one.