State-of-the-art language models have billions of parameters. Training these models within a manageable time requires distributing the workload across a large computing cluster. Ideally, training time would decrease linearly as the cluster size scales up. However, linear scaling is difficult to achieve because the communication required to coordinate the work of the cluster nodes eats into the gains from parallelization.
Recently, we put some effort into optimizing the communication efficiency of Microsoft’s DeepSpeed distributed-training library, dramatically improving performance for up to 64 GPUs. However, when we scale from tens of GPUs to hundreds, in the public cloud environment, communication overhead again begins to overwhelm efficiency gains.
In a paper that we'll present in 2023 at the International Conference on Very Large Data Bases (VLDB), we propose a method to make model training scale efficiently on hundreds of GPUs in the cloud. We call this method MiCS, because it minimizes communication scale to bring down communication overhead.
Specifically, where existing distributed-training frameworks such as DeepSpeed and FairScale divide a model state across all GPUs, MiCS makes multiple replicas of the model state and partitions each replica within a subset of GPUs. Depending on the model size, a replica may fit on a single computing node — a single machine with high-speed connections between its GPUs — or on multiple nodes.
Thus, in MiCS, frequent communication operations, like parameter gathering, are restricted to a subset of GPUs. In this way, when we scale a cluster up — by adding new replicas across new nodes — the communication latency of frequent communication operations remains fixed, rather than growing with the size of the cluster.
We also reduce the data volume transmitted between nodes in the event that a copy of the model state won’t fit in a single node. Lastly, MiCS includes a gradient synchronization schedule that amortizes expensive gradient synchronization among all workers.
Our experimental results show significant improvement in throughput and scaling efficiency on different-sized BERT models evaluated on clusters consisting of p3dn.24xlarge instances. MiCS is able to achieve near-linear scalability (denoted by the rectangular frames in the figure below) and provides up to 2.82-fold throughput compared to the second and third states of the three-stage zero-redundancy optimizer, or ZeRO, the communication management method built into DeepSpeed-v0.5.6 .
We have also compared MiCS with our earlier optimizations of ZeRO’s third stage (see figure below), demonstrating improvements even at the lower GPU counts that we investigated previously. We report all these findings in greater detail in a preprint paper on the arXiv.
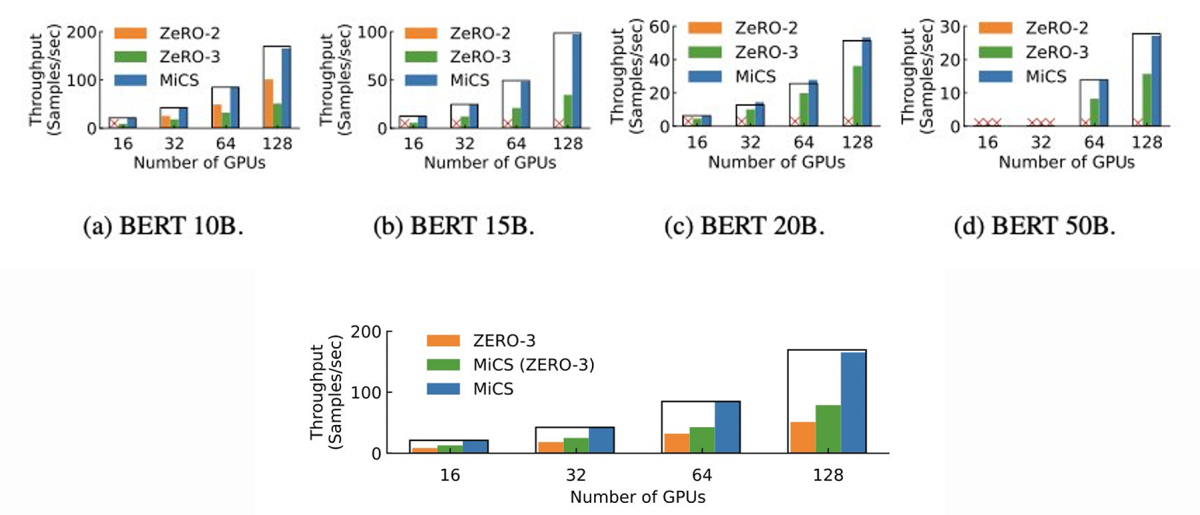
AWS P4d provides up to 400Gbps networking bandwidth for high-performance computing. Unfortunately, the distributed system may not be able to fully utilize 400Gbps efficiently because of communication overhead — especially latency, which increases when adding more GPUs to the cluster.
We have deployed MiCS to train proprietary models with up to 175 billion parameters on p4d.24xlarge (40GB A100) and p4de.24xlarge (80GB A100) instances. When training a 175-billion-parameter model with a sequence length of 2,048 on 16 p4de.24xlarge instances, we are able to achieve 169-teraflops (54.2% of the theoretical peak) performance on each GPU. When we train a 100-billion-parameter model on 64 p4d.24xlarge instances (512 A100 GPUs), MiCS maintains over 170 teraflops per GPU (54.5% of the theoretical peak).
When the size of the cluster is scaled from 128 GPUs to 512 GPUs, MiCS achieves 99.4% of the linear-scaling efficiency (as measured by the “weak scaling” metric). In contrast, DeepSpeed ZeRO’s third stage achieves only 72% weak-scaling efficiency and saturates at 62 teraflops per GPU (19.9% of the theoretical peak).
Scale-aware model partitioning
By default, DeepSpeed partitions model states across all devices, a strategy that lowers the memory consumption on each GPU in the cluster but incurs large communication overhead in training. More importantly, the overhead scales up with the size of the cluster, which causes the scalability to drop significantly at large scale.
Instead of partitioning model states to all GPUs, MiCS divides GPUs in the cluster into multiple groups and partitions model states within each group. We call these groups partition groups. Each group holds a complete replica of model states. The following figure gives an example of partition groups consisting of two consecutive GPUs. Those GPUs holding the same part of the model state form another kind of group, a replication group.
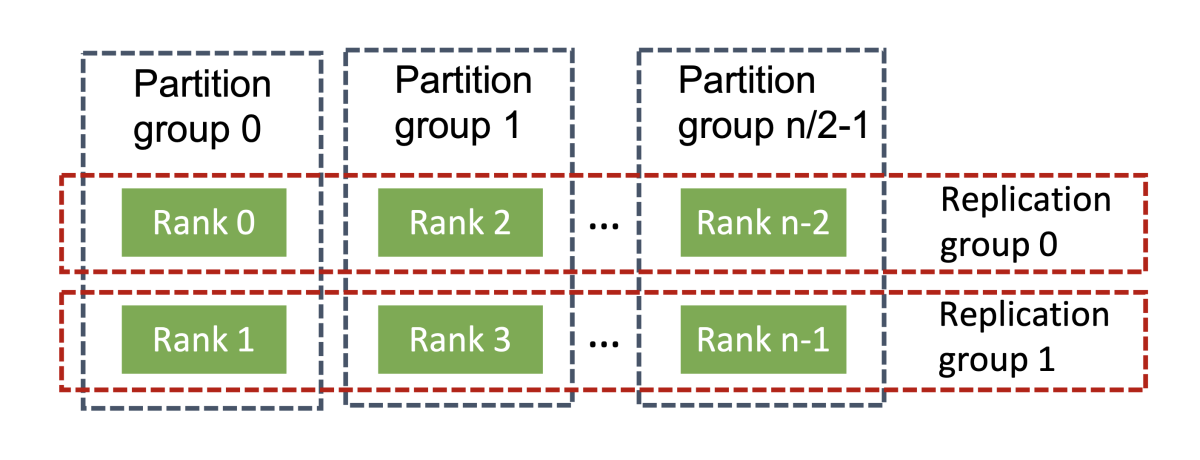
Partitioning model states within each partition group restricts the most frequent communications, parameter gathering and gradient synchronization, within a fixed number of GPUs. This strategy effectively controls the communication overhead and does not let it grow with the size of the cluster.
Hierarchical communication strategy
When the memory requirement for a single replica of the model state is larger than the total amount of GPU memory in a single node, we need to store the replica on GPUs spanning multiple nodes. In that case, we have to rely on less-efficient internode communication.
The volume of transmitted data and the latency in a collective communication are determined by the message size and the number of participants. Particularly, the communication volume is proportional to (p - 1)/p, where p denotes the number of participants, and if the participants use the standard ring-shaped communication pattern, the latency has a linear dependency on the number of participants.
The message size cannot be reduced without compromising data integrity, but we can reduce the number of participants in internode communications. This lowers the communication volume factor to (p - k)/p and latency by p/(p/k + k) times, where k is the number of GPUs on a single node.
Consider the simple example below, involving two nodes with two GPUs each. The standard ring-shaped communication pattern would aggregate data across nodes (left) by passing messages from each GPU to the next, so a single internode communication involves four GPUs.
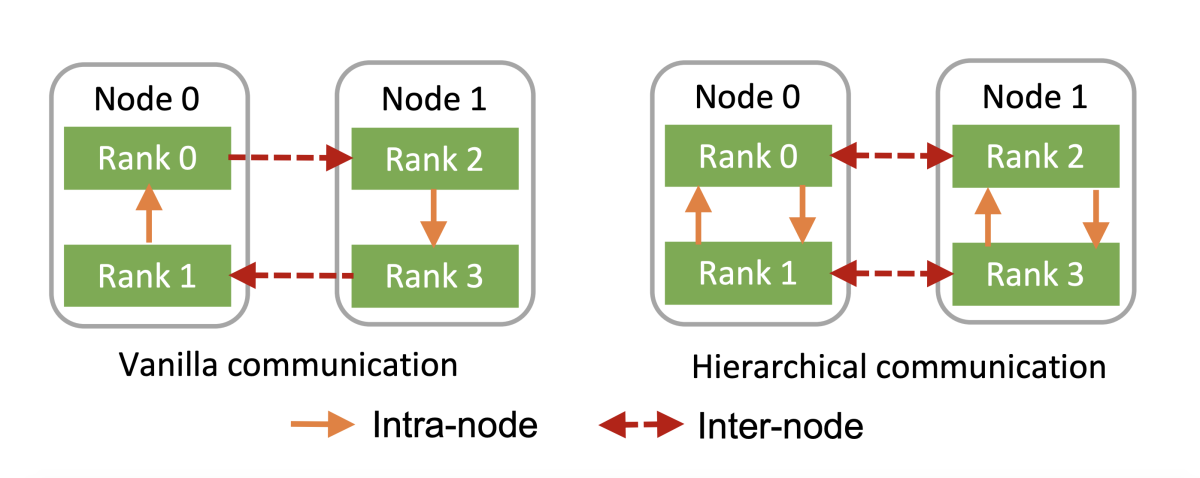
MiCS, by contrast, executes these internode operations in parallel, so each internode communication involves only two GPUs (right), which exchange only half the information that we want to communicate. Each node then aggregates the internode data locally to assemble the full message. In this case, the communication volume factor is reduced from ¾ ((4-1)/4) to ½ ((4-2/4).
Two-hop gradient synchronization
Synchronizing gradients among all workers is an expensive operation, required to keep workers working on the same model states. During the training of large neural nets, batch size is typically limited by GPU memory. Gradient accumulation is a technique that splits a batch of samples into several microbatches that will be run sequentially in multiple microsteps.
With MiCS, we can accumulate gradients inside each partition group in multiple microbatches until the last microbatch is processed. That is, for each microstep, we can accumulate the full set of gradients for each model replica inside a subset of GPUs (i.e., a partition group). Then, after the last microbatch is handled, each GPU synchronizes gradients with the other GPUs representing the same part of the model state.
This allows us to amortize the synchronization overhead across replication groups to multiple microsteps. The following figure gives an example of two-hop gradient synchronization for training with four microsteps.
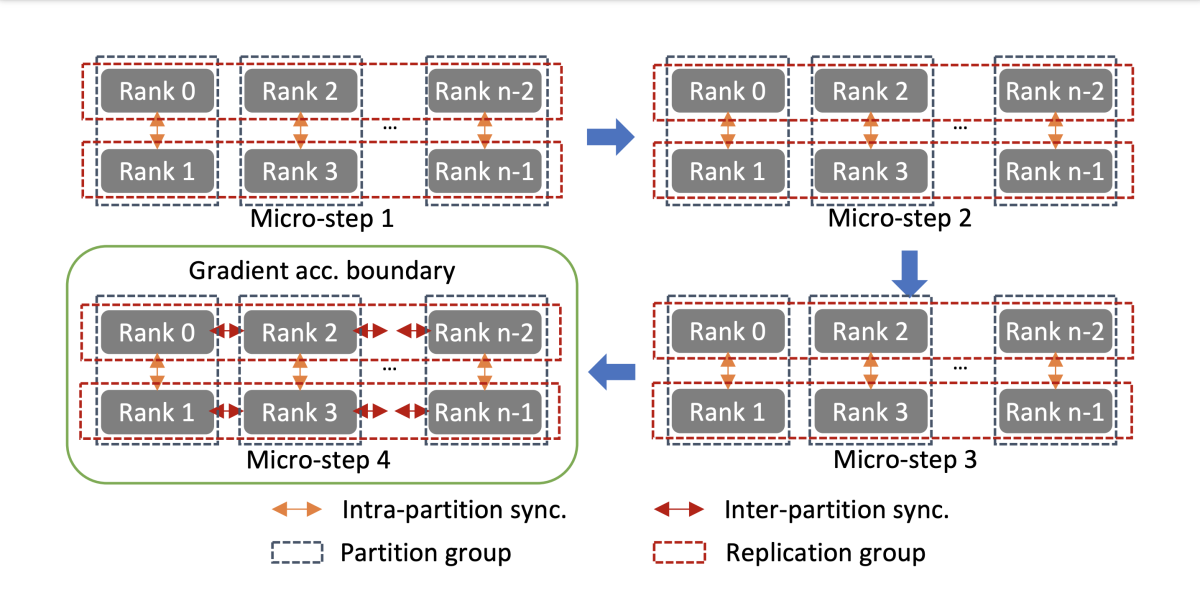
Because of these three techniques, MiCS shows great scalability on large clusters and delivers excellent training throughput performance, and it enables us to achieve a new state-of-the-art performance on AWS p4de.24xlarge machines.
We are working to open-source MiCS for public use, in the belief that it will greatly reduce the time and cost of large-model training on the Amazon EC2 platform. Please refer to our preprint for a more detailed explanation of our system and analysis of its performance.
Acknowledgements: Yida Wang, Justin Chiu, Roshan Makhijani, RJ, Stephen Rawls, Xin Jin