As neural networks grow in size, deploying them on-device increasingly requires special-purpose hardware that parallelizes common operations. But for maximum efficiency, it’s not enough to optimize the hardware for the networks; the networks should be optimized for the hardware, too.
The standard way to optimize a neural network is through neural-architecture search (NAS), where the goal is to minimize both the size of the network and the number of floating-point operations (FLOPS) it performs. But this approach doesn’t work with neural chips, which can often execute easily parallelized but higher-FLOPS tasks more rapidly than they can harder-to-parallelize but lower-FLOPS tasks.
Minimizing latency is a more complicated optimization objective than minimizing FLOPS, so in the Amazon Devices Hardware group, we’ve developed a number of strategies for adapting NAS to the problem of optimizing network architectures for Amazon’s new Neural Engine family of accelerators. Those strategies involve curating the architecture search space to, for instance, reduce the chances of getting stuck in local minima. We’ve also found that combining a little human intuition with the results of NAS for particular tasks can help us generalize to new tasks more reliably and efficiently.
In experiments involving several different machine learning tasks, we’ve found that our NAS strategies can reduce latencies by as much as 55%.
Varieties of neural-architecture search
NAS needs three things: a definition of the search space, which specifies the building blocks available to construct a network; a cost model, which is a function of the network's accuracy, latency, and memory; and an optimization algorithm. We use a performance estimator to measure latency and memory footprint, but to measure accuracy, we must train the network. This is a major bottleneck, as training a single network can take days. Sampling thousands of architectures would take thousands of GPU days, which is clearly neither practical nor environmentally sustainable.
There are three categories of NAS algorithm, which require networks to be trained different numbers of times: multishot, single-shot, and zero-shot.
Multishot methods sample a cohort of architectures in each iteration. Each network is trained and evaluated for accuracy and performance, and the next set of architectures is sampled based on their cost. Evolutionary or reinforcement-learning-based algorithms are generally used for multishot methods.
Single-shot methods start with a large network called the supernet, which has multiple possible subgraphs. During training, the subgraphs start converging to a single, small network. Single-shot methods are designed to be trained only once, but their training takes much longer than that of a single network in multishot methods.
Zero-shot methods works like multishot methods, with the key difference that the network is never trained. As a proxy for accuracy, we use the network’s trainability score, which is computed using the network's topology, nonlinearity, and operations. Zero-shot methods are the fastest to converge, because calculating the score is computationally very cheap. The downside is that the trainability may not correlate well with model accuracy.
Search space curation
The NAS cost function can be visualized as a landscape, with each point representing a potential architecture. A cost function based on FLOPS changes monotonically with factors such as sizes or channels: that is, if you find a direction across the terrain in which the cost is going down, you can be sure that continuing in that direction will not cause the cost to go up.
However, the inclusion of accelerator-aware constraints disrupts the function by introducing more asymptotes, or points at which the cost switches from going down to going up. This results in a more complex and rocky landscape.
To address this issue, we reduced the number of options in the search space. We were exploring convolutional architectures, meaning that the inputs are decomposed into several different components, each of which has its own channel through the network. The data in each channel, in turn, is filtered in several different ways; each filter involves a different data convolution.
Previously, we would have explored the number of channels — known as the channel size — at increments of one; instead, we considered only a handful of channel sizes. We limited the options for channel sizes to certain values that were favorable for the parallelism factor of the Neural Engine. The parallelism factor is a count of operations, such as dot product, that can be performed in parallel. In some cases, we even added "depth multiplier" ratio that could be used to scale the number of channels across the entire model to the search space.
These improvements can be visualized as taking fewer, larger steps across a smoother terrain, rather than trying to navigate the rocky landscape that resulted from the inclusion of accelerator-aware performance in the cost function. During the optimization process, they resulted in a faster convergence rate because of the reduced number of options and in improved stability and reliability thanks to the monotonic nature of the curated search space.
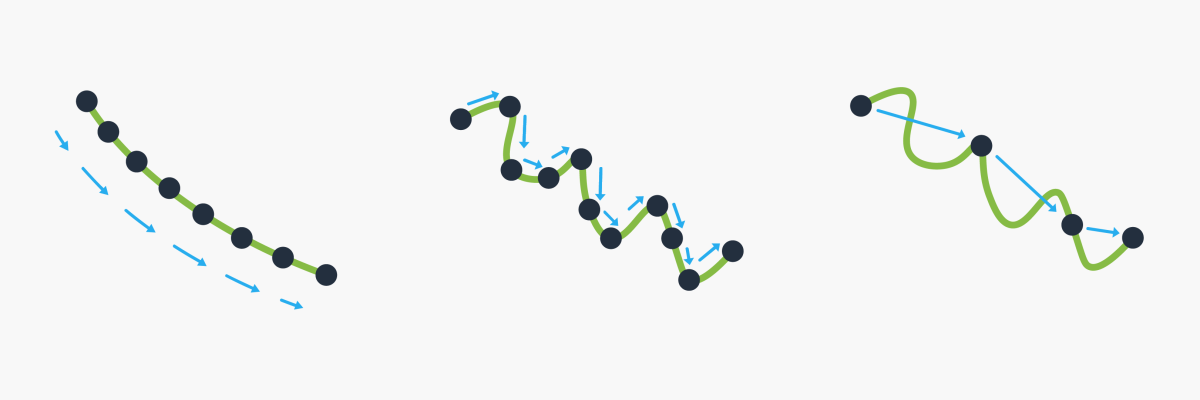
One key detail in our implementation is the performance estimator. Instead of deploying an architecture on real hardware or an emulator to obtain performance metrics, we estimated them using a machine learning regression model trained on measurements of different operators or subgraphs.
At inference time, the estimator would decompose the queried architecture into subgraphs and use the regression model to estimate the performance of each. Then it would accumulate these estimates to give the model-level performance. This regressor-based design simplified our NAS framework, as it no longer required compilation, inference, or hardware. This technique enables us to test accelerators in the design phase, before we’ve developed custom compilers and hardware emulators for them.
Productizing NAS with expert-in-the-loop
Curating the search space improves convergence rate, stability, and reliability, but transferability to new use cases is not straightforward. NAS results for a detector model, for instance, may not be easy to transfer to a classification model. On the other hand, running NAS from scratch for each new dataset may not be feasible, due to time constraints. In these situations, we found that combining NAS results and human expertise was the fastest approach.
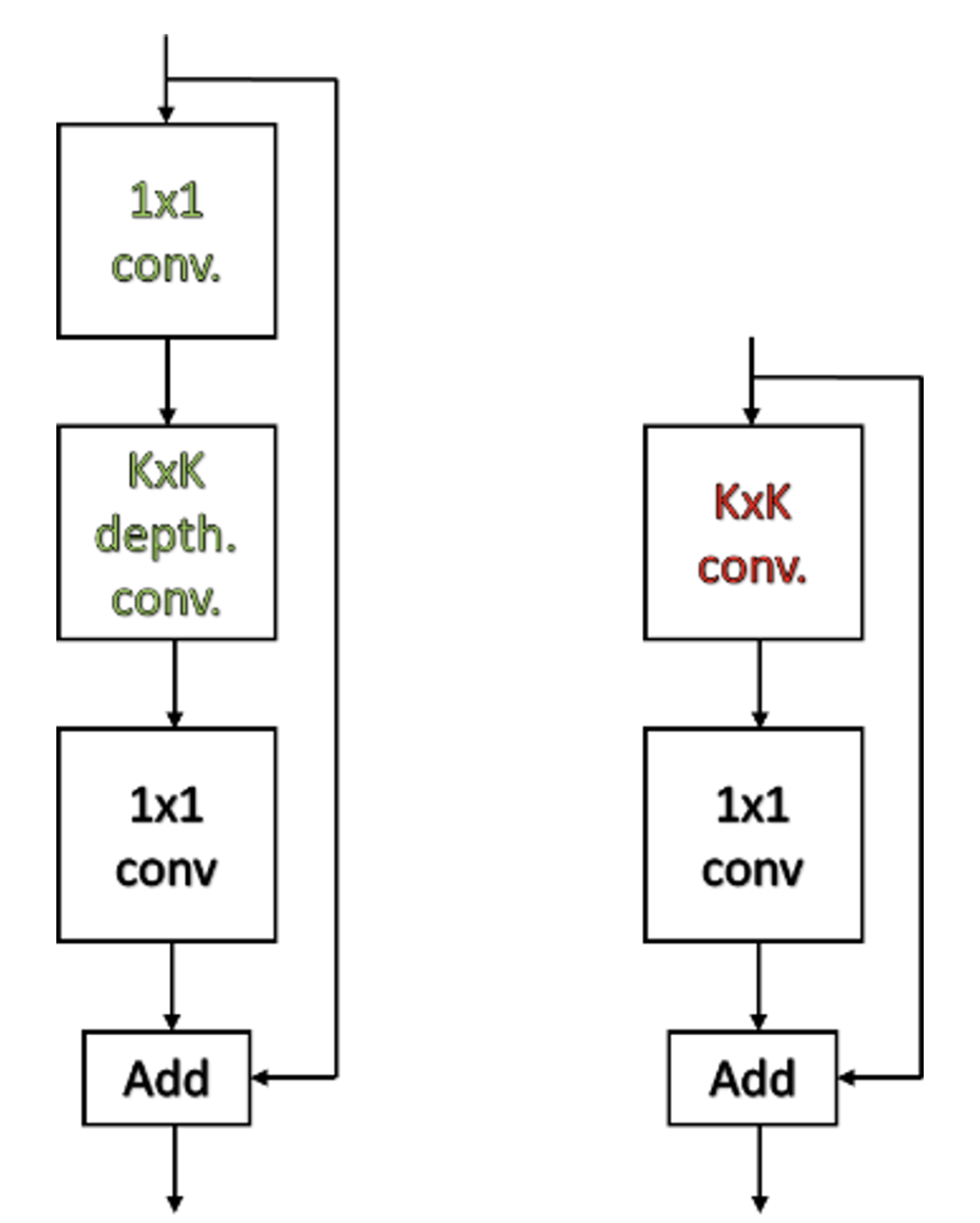
When we performed NAS on different datasets, we saw common patterns, such as the fusion of convolution layers with previous convolution layers, reducing the number of channels and, aligning them with the hardware parallelism factor.
In particular, fusing convolution layers in inverted bottleneck (IBN) blocks contributed most to boosting efficiency. With just these modifications, we observed latency reductions of up to 50%, whereas a fully converged NAS model would yield a slightly better 53% reduction.
In situations where running NAS from scratch is not feasible, a human expert can rely on mathematical intuition and observations of the results of NAS on similar datasets to build the required model architecture.
Results and product impact
We applied this technique to multiple products in the Amazon Devices portfolio, ranging from Echo Show and Blink home security products to the latest Astro, the in-home consumer robot.
1. Reduced detection latency by half on Echo Show
Echo Show runs a model to detect human presence and locate the detected person in a room. The original model used IBN blocks. We used accelerator-aware NAS to reduce the latency of this model by 53%.
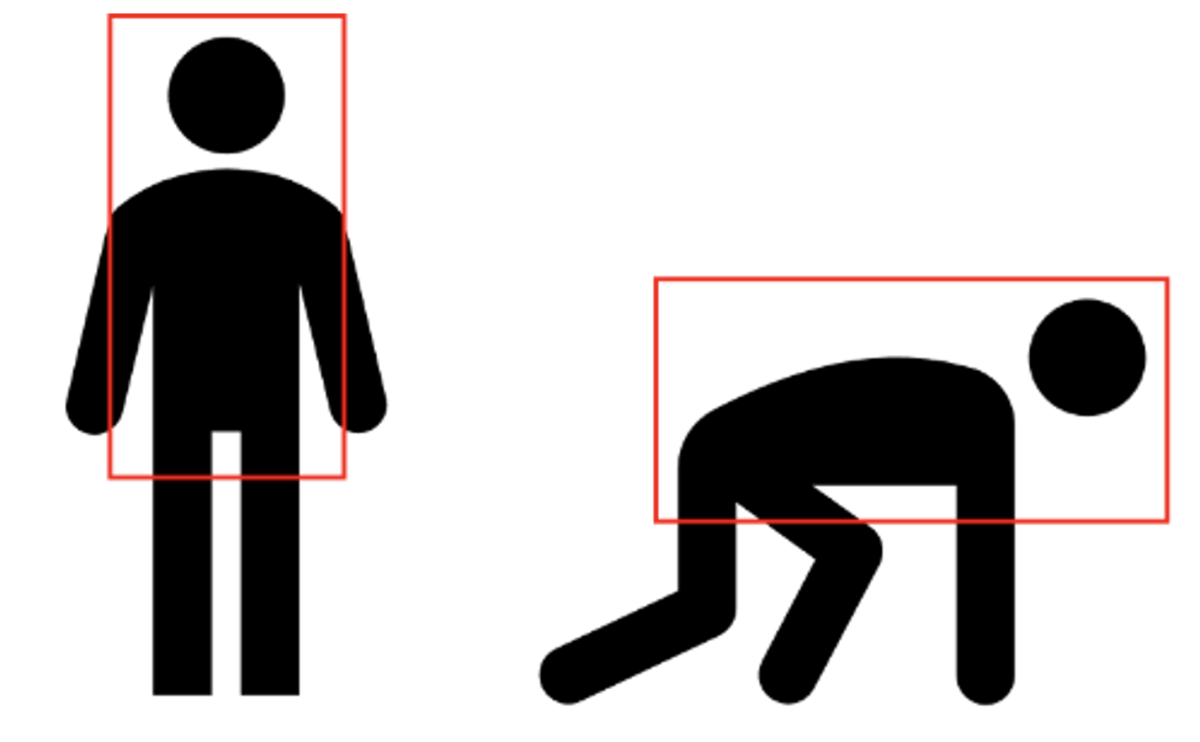
We performed a search for depth multipliers — that is, layers that multiply the number of channels — and for opportunities to replace IBN blocks with fused-IBN blocks. The requirement was to maintain the same mean average precision (mAP) of the original model while improving the latency. Our V3 model improved the latency by more than 53% (i.e. 2.2x faster) while keeping the mAP scores same as baseline.
Latency results for the original model and three models found through NAS. |
|||
Fused-IBN search |
Depth multiplier search |
Latency reduction (%) |
|
Baseline |
No |
No |
Baseline |
V1 |
No |
Yes |
14% |
V2 |
Yes |
No |
35% |
V3 |
Yes |
Yes |
53% |
After performing NAS, we found that not every IBN fusion improves latency and accuracy. The later layers are larger, and replacing them with fused layers hurt performance. For the layers where fusion was selected, the FLOPs, as expected, increased, but the latency did not.
2. Model fitting within the tight memory budget of the Blink Floodlight Camera
Blink cameras use a classification model for security assistance. Our goal was to fit the model parameters and peak activation memory within a tight memory budget. In this case, we combined NAS techniques with an expert-in-the-loop to provide fine-tuning. The NAS result on the classification dataset provided intuition on what operator/subgraph changes could extract benefits from the accelerator design.
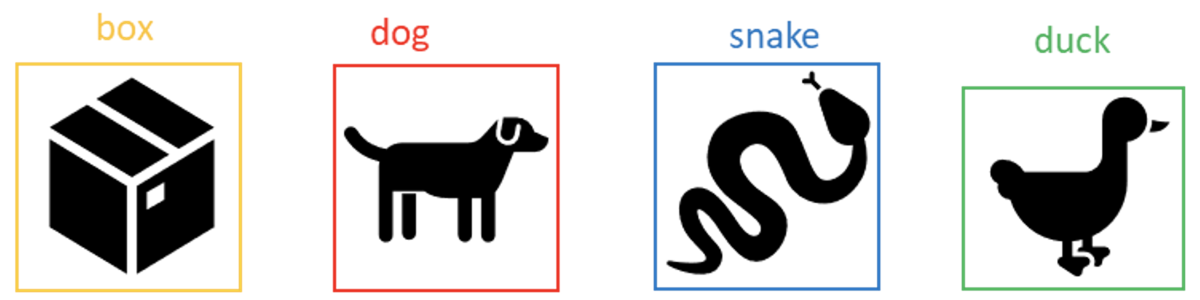
The expert recommendations were to replace the depth-wise convolutions with standard convolutions and reduce the channels by making them even across the model, preferably by a multiple of the parallelism factor. With these changes, model developers were able to reduce both the model size and the intermediate memory usage by 47% and fit the model within the required budget.
3. Fast semantic segmentation for robotics
In the context of robotics, semantic segmentation is used to understand the objects and scenes the robot is interacting with. For example, it can enable the robot to identify chairs, tables, or other objects in the environment, allowing it to navigate and interact with its surroundings more effectively. Our goal for this model was to reduce latency by half. Our starting point was a semantic-segmentation model that was optimized to run on a CPU.
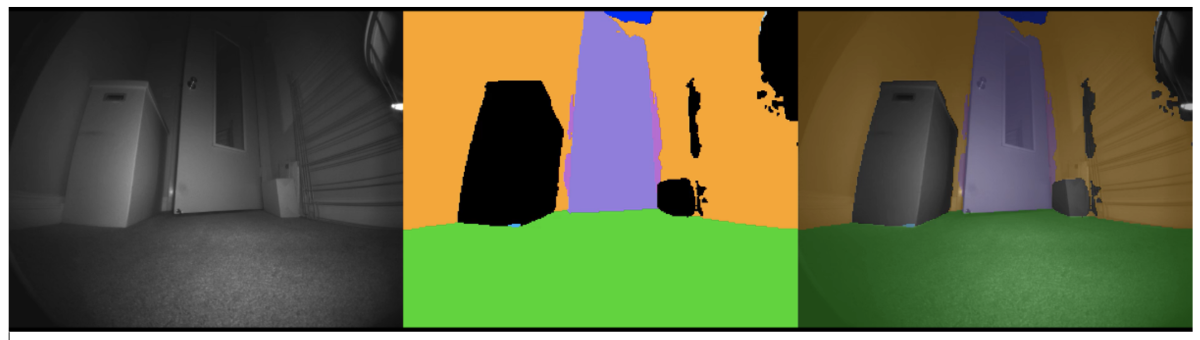
For this model, we searched for different channel sizes, fusion, and also output and input dimensions. We used the multishot method with the evolutionary search algorithm. NAS gave us multiple candidates with different performances. The best candidate was able to reduce the latency by half.
Latency improvement for different architectures found through NAS. |
|
Latency reduction (%) |
|
Original |
Baseline |
Model A |
27% |
Model B |
37% |
Model C |
38% |
Model D |
41% |
Model E |
51% |
4. User privacy with on-device inference
Amazon's Neural Engine supports large-model inference on-device, so we can process microphone and video feeds without sending data to the cloud. For example, the Amazon Neural Engine has enabled Alexa to perform automatic speech recognition on-device. On-device processing also provides a better user experience because the inference pipeline is not affected by intermittent connection issues. In our NAS work, we discovered that even larger, more accurate models can now fit on-device with no hit on latency.
Making edge AI sustainable
We mentioned earlier that multishot NAS with full training can take up to 2,000 GPU-days. However, with some of the techniques described in this blog, we were able to create efficient architectures in a substantially shorter amount of time, making NAS much more scalable and sustainable. But our sustainability efforts don't end there.
Because of its parallelism and mixed-precision features, the Neural Engine is more power efficient than a generic CPU. For a million average users, the difference is on order of millions of kilowatt-hours per year, equivalent to 200 gasoline-powered passenger vehicles per year or the energy consumption of a hundred average US households.
When we optimize models through NAS, we increase the device's capability to run more neural-network models simultaneously. This allows us to use smaller application processors and, in some cases, fewer of them. By reducing the hardware footprint in this way, we are further reducing the carbon footprint of our devices.
Future work
We have identified that curation requires an expert who understands the hardware design well. This may not scale to future generations of more complex hardware. We have also identified that in situations where time is tight, having an expert in the loop is still faster than running NAS from scratch. Because of this, we are continuing to investigate how NAS algorithms with accelerator awareness can handle large search spaces. We are also working on improving the search algorithm’s efficiency and effectiveness by exploring how the three categories of algorithms can be combined. We also plan to explore model optimization by introducing sparsity through pruning and clustering. Stay tuned!
Acknowledgements: Manasa Manohara, Lingchuan Meng, Rahul Bakshi, Varada Gopalakrishnan, Lindo St. Angel