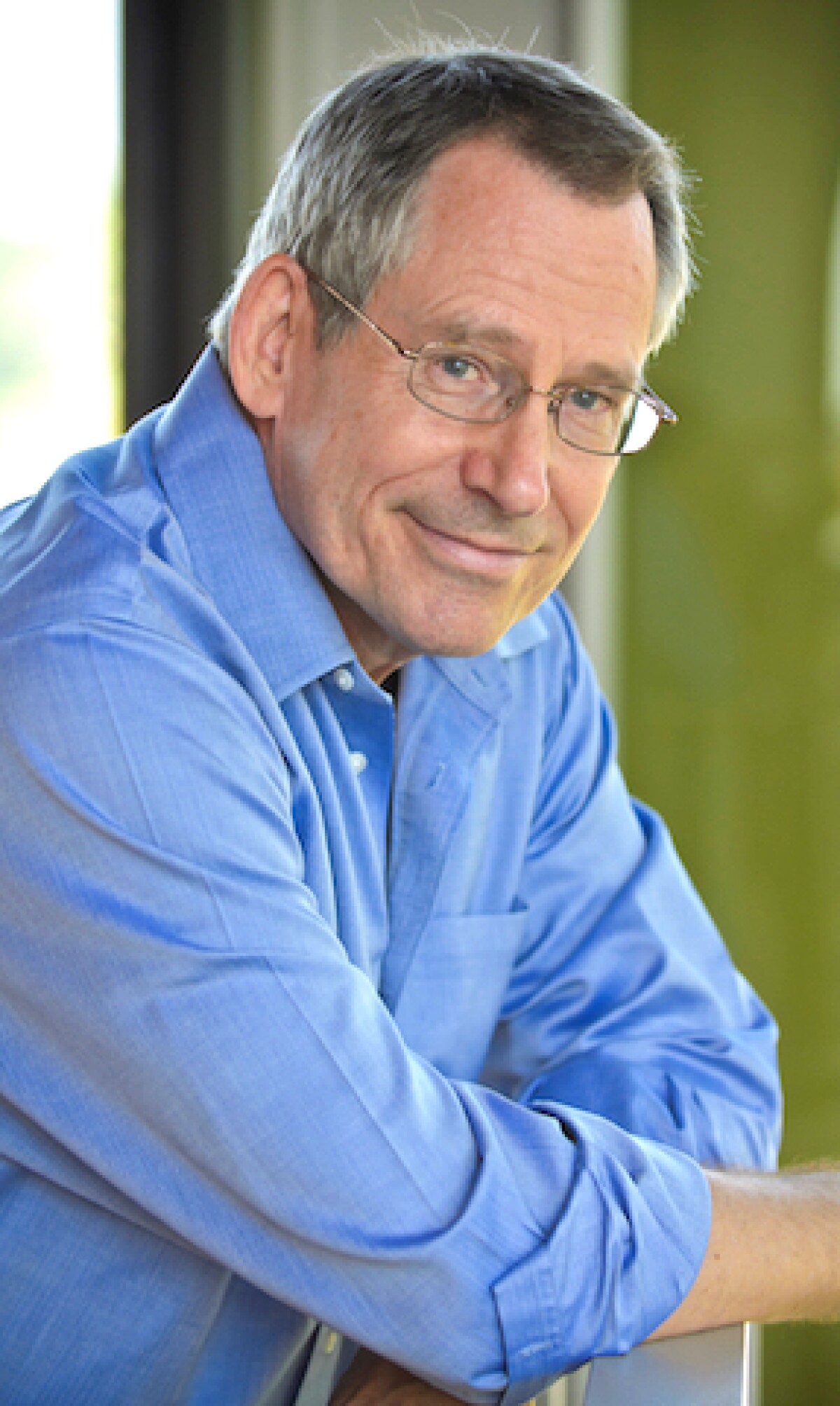
In August 2024, at the 10th International Conference on Quantum Information and Quantum Control, John Preskill, the Richard P. Feynman Professor of Theoretical Physics at the California Institute of Technology and an Amazon Scholar, will receive the John Stewart Bell Prize for Research on Fundamental Issues in Quantum Mechanics and Their Applications. The prize is named for the European Organization for Nuclear Research (CERN) physicist John Bell, who showed how to prove the existence of quantum entanglement, or strong correlations between the physical states of quantum systems, even when they’re separated by great distances.
The prize announcement cites Preskill’s work “at the interface of efficient learning and processing of quantum information in quantum computation”, work that explores both classical and quantum techniques for using machine learning to deepen our understanding quantum systems. Preskill recently took some time to explain his prize-winning work to Amazon Science.
- Q.
Can you describe the work that won the prize?
A.You could put it in two categories, which we could call learning about the quantum world using classical machines and using quantum machines. People have quantum computers now with hundreds of quantum bits, or qubits, and completely characterizing the state of a quantum computer with hundreds of qubits is beyond our ability, because that complete description grows exponentially with the number of qubits.
If we're going to make progress, we have to have some way of translating that quantum information to classical information that we can understand. So part of our work — and this was with two brilliant collaborators, Robert Huang, a student, and Richard Kueng, a postdoc — was a way of translating this very complex quantum system to a succinct classical description.
What we showed is that there's a way of doing a relatively modest number of experiments that gives you a description of the quantum system from which you can predict very many properties — a lot more properties than the number of measurements that you had to make. We call this description a “classical shadow”.
Computing "classical shadows" is analogous to projecting a 3-D object into two dimensions along multiple axes. Let's say there's a three-dimensional object, and we're trying to understand its geometry. We can take snapshots of it from different directions, which project it on two dimensions. This is kind of like that only on steroids, because the quantum system lives in some unimaginably large dimension, and we're projecting it down to a little bit of information. What we showed is that you don't need so many of these snapshots to be able to predict a lot of things that a physicist would typically be interested in.
We'd like to use the data that we get from quantum experiments and generalize to predict what we'll see when we look at related quantum systems or when we look at the same quantum system in a different way. And you know, AI is everywhere these days, and a lot of people are thinking about applying machine learning to understanding quantum systems. But it's mostly very heuristic: people try different things, and they hope that gives them the ability to generalize and make good predictions.
The computational pipeline for learning about quantum systems with classical computers. What we wanted to do is to give rigorous performance guarantees that you don't need that many of these snapshots in order to generalize with a small error. And we were able to prove that in some settings.
When it comes to learning with quantum machines, now let's do something different. Let's grab some quantum data — maybe we produce it on a quantum computer, or we have a sensing network that collected some photons from somewhere — and store that in a quantum memory. We don’t just measure it and put it in a classical memory; we store it in a quantum memory, and then we do a quantum computation on that data. And finally, at the end of the computation, we get a classical answer, because at the end of a quantum computation, you always do.
What we were able to show is that, for some properties of the quantum system that you might want to know, it's vastly more efficient to process with a quantum computer than a classical computer.
- Q.
In the case of the “classical shadows”, do you have to reset the system after each measurement?
A.I'm imagining a scenario in which I have access to many identically prepared copies. Now, I might have prepared them with a quantum computer, and I went through the same steps of the computation each time. Or maybe there was some experiment I did in the lab, which I can repeat over and over again. The main point of our work was, you don't need as many copies as you thought you might. Technically, the number of predictions we can make with some fixed accuracy, based on measuring the same state many times, is exponential in the number of copies that we measure.
- Q.
Do you have to know what questions you want to ask before you start making measurements?
A.We have a slogan, which is “Measure first, ask questions later”, because it turns out that no, you don't need to know what properties you're going to want to learn at the time that you make the measurements. And as a result, the measurements that we require for creating a classical shadow really are experimentally feasible today, because all you have to do is measure the individual qubits.
The trick is you measure them in a random basis. There are different ways of looking at a qubit. You can, so to speak, look at it straight up and down or horizontally or back and forth. So there are three types of measurements we consider, and for all the qubits, we randomly choose to measure in one of those three ways.
There's some power that comes from the randomness there. Later, you can say, Okay, I want to use that data and predict something like a correlation function for a clump of qubits here and a clump there, or maybe the expectation value of the energy of some quantum system, and just by processing that randomized data, I can make that prediction.
- Q.
What’s the setup in the quantum learning context?
A.The quantum setting is you can take two copies at once, store them in a quantum memory, and then do a computation across the two copies. We call that an entangled or entangling measurement of the two copies. And that's where the power comes from. When you do an entangling measurement on two copies at a time, that enables us to, in some cases, vastly reduce the number of experiments we need to do to predict the properties.
Of course, in a real computer, there's noise, which is always a factor. But if everything's noiseless, then for the particular case that we studied, the number of measurements that suffice when you do these entangling measurements across the copies is a constant. It doesn't depend on how large the system is. But if you measure one copy at a time, the theorem says that to get that same measurement accuracy, you'd have to measure a number of copies which is exponential in the number of qubits.
- Q.
What applications could this have?
A.What we imagine doing eventually, which I think will be very empowering, is a new kind of quantum sensing. If we are observing light from some source, what do we do now? We count photons, typically: with a camera, you've got pixels that flash when they get hit by photons.
If there's a state of many photons that has come from some source — maybe you’ve got telescopes, and you're looking at something coming in from space — there's a lot of information, at least in principle, in the quantum correlations among those photons. And we miss that if we're just counting photons. You're throwing away a tremendous amount of information in that many-photon state.
What we can imagine, when we have the technology to do it, is our telescopes won't just count photons. They'll collect this many-photon state and store it in a quantum memory, including multiple images, and then we can come along and do this collective measurement on the multiple copies. And we'll be able to see things in that signal that we would just miss if we do things the conventional way, measuring one copy at a time.
- Q.
One last question: the prize is named for John Bell, who proposed an experiment to prove that measurements on entangled particles really do depend on each other, even if the particles are separated by enormous distances. Does your work relate to Bell’s in some way?
A.The charge to the committee that selects the awardee is to identify research that advances the foundations of quantum theory. And of course, Bell did that by formulating Bell inequalities showing that quantum entanglement enables us to do things that we couldn't do without quantum entanglement. That's the point of experimental demonstrations of violations of Bell's inequality.
Part of Bell's legacy is that quantum entanglement is a resource that, if we know how to make use of it, enables us to do things we couldn't otherwise do — more-powerful computations, new kinds of measurements, new kinds of communication. So I think, at least in that sense, the work we've been talking about is very much following in Bell's footsteps — as is the whole field of quantum computing, in a way. Because I think the power of quantum computers really comes from the feature that in the middle of a quantum computation, you're dealing with a very highly entangled state of many qubits that we don't know how to represent classically.