At the annual meeting of the North American chapter of the Association for Computational Linguistics in June, researchers at Amazon and the University of Sheffield released a new dataset that can be used to train machine-learning systems to determine the veracity of factual assertions online. The dataset is called FEVER, for fact extraction and verification.
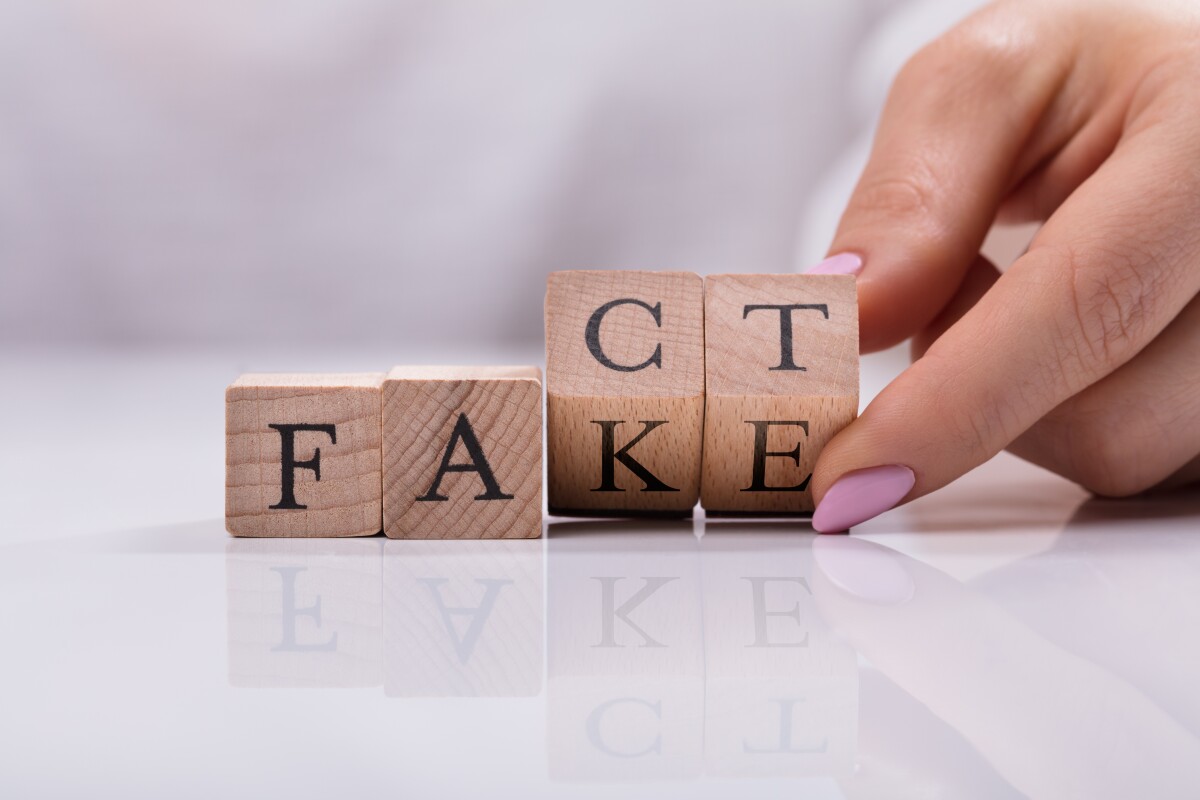
Just two months later, the dataset’s developers have announced preliminary results of an online competition to build fact-verification systems using the FEVER data. Submissions from 23 university, industry, and independent teams were judged according to their “FEVER scores,” which measure both the accuracy of their truth assessments and the quality of the evidence they present to support them.
The leading entry so far, from a team at the University of North Carolina, earned a preliminary FEVER score of 64% — a 134% improvement over the 27% score achieved by the baseline system that the FEVER developers reported in their paper.
“A great result that significantly beats the baseline — which was already an advanced system,” says Arpit Mittal, a senior machine learning scientist with the Alexa information domain group, who, together with Christos Christodoulopoulos, an applied scientist in the same group, led Amazon’s contribution to the project. “There is still a lot of room for improvement, though, demonstrating how challenging this problem is.”
Representatives of the top finishers in the competition will describe their approaches at a workshop at the 2018 Conference on Empirical Methods in Natural Language Processing. The workshop’s organizers have also issued a call for papers that will be presented at the workshop, either orally or in poster sessions. “Publications accepted to the workshop will show advances beyond state of the art in the fields of information verification, fact checking, argumentation, or related topics,” Mittal says.
The FEVER dataset consists of 185,000 assertions of fact, together with sentences from Wikipedia entries that either substantiate or refute them. The true assertions were extracted from Wikipedia, although they often combine assertions from distinct articles (for instance, “Colin Firth is a Gemini,” which combines assertions from the articles on Colin Firth and the Gemini zodiac sign); the false assertions were created by mutating true assertions. The evidentiary sentences were proposed by human annotators, and a subset of them underwent thorough validation, as a quality-control mechanism.
“We wanted to maximize use of the dataset,” Mittal says, “so we publicly released the data and the annotation tools used to create it. The sentences themselves are pulled from Wikipedia, so others can reproduce the data set — and extend it.”
Systems entered in the competition had two tasks: assess the truth of assertions and justify those assessments with sentences extracted from Wikipedia. The sentences selected by the machine-learning systems, however, may sometimes differ from those selected by the human annotators.
That’s why the current scores are preliminary. Mittal, Christodoulopoulos, and their colleagues are subjecting evidentiary sentences that differ from those in the dataset to the same validation procedures they used to produce the dataset in the first place. Sentences that hold up will be added to the dataset, and all the contestants’ entries will be reevaluated. That’s likely to result in improvements in several contestants’ scores.
“We are making sure that we are not penalizing the system just because it’s better than human,” Christodoulopoulos says.
At the moment, however, the leaders are teams from, in order, the University of North Carolina, University College London, and Technical University Darmstadt.