More than 60% of sales in Amazon’s store come from independent sellers. One of the big drivers of this growth has been Fulfillment by Amazon, or FBA, which is an optional program to let sellers outsource order fulfillment to Amazon. FBA provides customers access to a vast selection of products at fast delivery speeds, and it lets sellers leverage Amazon’s global logistics network and advanced technology to pick, pack, and ship customer orders and handle customer service and returns. FBA also uses state-of-the-art optimization and machine learning models to provide sellers with inventory management recommendations, such as how much of which products to stock, how to promote products through sponsored ads, and whether and when to sell excess inventory at a discount.
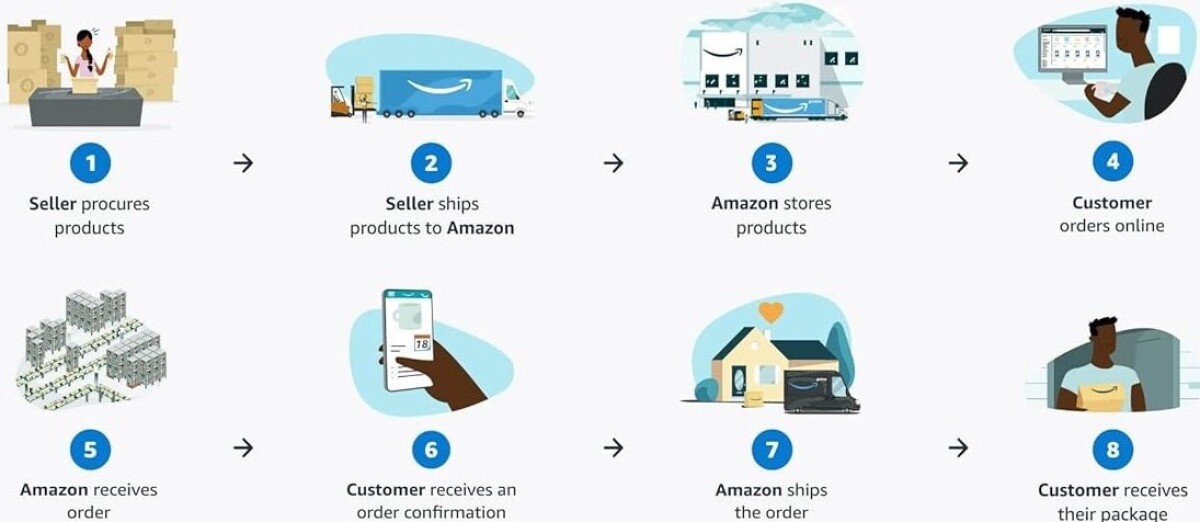
The goal of these recommendations is to improve seller performance — for example, to maximize seller-relevant outcome metrics such as revenue, units shipped, and customer clicks on product listings. To determine whether the recommendations are working, we would like to compare the results sellers get from aligning with Amazon FBA recommendations with the results they would get from not aligning with them.
But performing that comparison is not as simple as comparing outcomes for two seller populations, those who follow the recommendations and those who don’t. That’s because of the so-called selection bias: the very traits that cause some sellers to follow a recommendation could mean that if they hadn’t followed it, their outcomes would differ from those of sellers who in fact did not follow the same recommendation.
At this year’s meeting of the Institute for Operations Research and the Management Sciences (INFORMS), we’re presenting a tutorial that shows how to use cutting-edge causal machine learning methods to filter out selection bias when estimating the effects of FBA recommendations.
To build the causal model, we use double machine learning. Specifically, we train two machine learning models: one predicts whether each seller will follow the recommendation, based on inputs such as inventory management history and product characteristics; the other predicts seller outcomes, using the same inputs as the first model plus the sellers' acceptance decisions. We use the predictions of these models to account for any selection bias that can’t already be determined from the observed data, as we explain below.
Using this methodology, we have shown why and how much FBA recommendations improve seller outcomes. We surface the efficacy estimates to sellers through the Seller Central page to increase awareness and adoption.
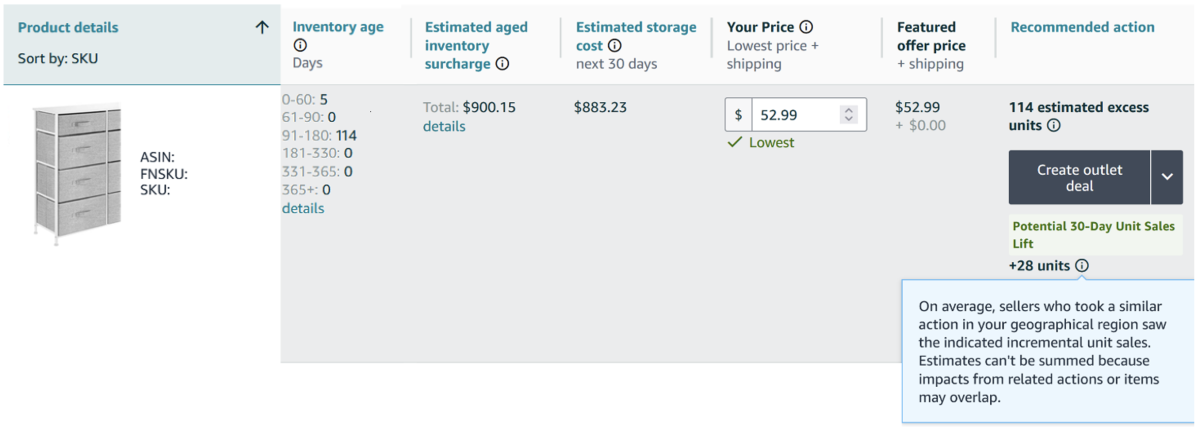
Selection bias
To measure and monitor the efficacy of such recommendations, we would, ideally, run experiments regularly. But we don’t run such experiments, because we want to preserve a positive seller experience and maintain fairness, and we do not want to negatively influence seller decisions. Let us explain.
An experiment involves two groups: the treatment group, which receives an intervention (such as a recommendation), and the control group, which doesn’t receive this intervention. A well-designed experiment would randomly assign some participants to the treatment group and others to the control group to ensure unbiased comparisons.
To avoid subjecting sellers to such differential treatment, we instead rely on data that we collect by observing sellers’ decisions and the resulting outcomes. Our methodology is, therefore, well-suited for environments in which experimentation is potentially infeasible (e.g., healthcare, where experimentation would disrupt patient treatment and outcomes).
Selection bias occurs when the assignment to treatment and control groups is not random, and the factors determining group membership also influence outcomes. In our case, the treatment group comprises sellers who decide to align their actions with the recommendations, and the control group consists of sellers who opt not to follow the recommendations. In other words, sellers are not assigned randomly but rather self-select to be in either of these groups.
Therefore, it is possible that sellers who are proactive and knowledgeable in managing their inventory may decide to be in the treatment group, whereas sellers who are less concerned with inventory management may decide to be in the control group. In this case, attributing the treatment group’s higher revenue solely to FBA recommendations would be incorrect, because a portion of that attribution is likely due to sellers’ prior knowledge about inventory management and not about whether they follow FBA recommendations.
It is also possible that the members of the control group already have such a thorough understanding of inventory management that they feel they don’t need FBA’s recommendations, and as a consequence, their outcomes are better than the treatment group’s would be in the absence of the intervention. Therefore, simply comparing the two groups’ outcomes is insufficient: a different method is needed to rigorously quantify the efficacy of following FBA recommendations.
Double machine learning
Our solution is to use double machine learning (DML), which combines two models to estimate causal effects: one model estimates the expected seller outcome, given the decision to align or not align with the recommendation; the other estimates the propensity to align with the recommendation. Variation in those propensities is the source of the selection bias.
Each model receives hundreds of inputs, including inventory management and product data. For each seller, we compute the residual of the seller outcome model (the difference between the model’s prediction and the actual outcome) and the residual of the seller decision model (the difference between the model’s prediction and the seller’s actual decision to follow recommendation). These residuals represent the unexplained variation in the seller outcome and the seller decision — the variation not explained by observable data.
Therefore, we “remove” any influence our inputs (e.g., the experience level of the seller) may have on the treatment effect estimate. When we regress the residuals of the outcome model on the residuals of the decision model, we estimate the impact of the unexplained variation in treatment status on the unexplained variation in the outcome. The resultant estimand is thus the causal impact of the seller’s decision to follow recommendations on the outcome.
In our tutorial, we show how to use this method to compute the average treatment effect (ATE), the average treatment effect on the treated (ATT), and the conditional average treatment effect (CATE). ATE is the overall effect of the treatment (following the FBA recommendation) on the entire population of FBA sellers. It answers the question “On average, how much does following the recommendation change the seller outcome compared to not following the recommendation?”
ATT focuses on sellers who actually followed the recommendation. It answers the question “For those who followed the recommendation, what was the average effect compared to not following the recommendation?”
CATE breaks it down even further, looking at specific subgroups based on characteristics such as product category or current inventory level. It answers the question “For a specific group of sellers and products, how does following the recommendation affect them compared to not following the recommendation?”
Our approach is agnostic as to the type of machine learning model used. But we observe that, given the scale and tabular nature of our data, gradient-boosted decision trees offer a good compromise between the high efficiency but lower accuracy of linear-regression models and the high accuracy but lower efficiency of deep-learning models. Readers who are interested in the details can attend the INFORMS tutorial — or read our paper in the forthcoming issue of the TutORials in Operations Research journal.
In closing, before we make recommendations to sellers to help improve their outcomes, we carry out rigorous scientific work to build the recommendation algorithms, monitor their outcomes, and revise and rebuild them to ensure that seller outcomes really do improve.
Acknowledgments:
Xiaoxi Zhao, Ethan Dee, and Vivian Yu for contributing to the tutorial; FBA scientists for contributing to the Seller Assistance Efficacy workstream; Michael Miksis for managing the related product and program; FBA product managers and engineers for pushing the outcome of this workstream into their respective products; Alexandre Belloni and Xinyang Shen for their constructive suggestions; and WW FBA Leadership for their support.