At next week’s Interspeech, the largest conference on the science and technology of spoken-language processing, Alexa researchers have 16 papers, which span the five core areas of Alexa functionality: device activation, or recognizing speech intended for Alexa and other audio events that require processing; automatic speech recognition (ASR), or converting the speech signal into text; natural-language understanding, or determining the meaning of customer utterances; dialogue management, or handling multiturn conversational exchanges; and text-to-speech, or generating natural-sounding synthetic speech to convey Alexa’s responses. Two of the papers are also more-general explorations of topics in machine learning.
Device Activation
Model Compression on Acoustic Event Detection with Quantized Distillation
Bowen Shi, Ming Sun, Chieh-Chi Kao, Viktor Rozgic, Spyros Matsoukas, Chao Wang
The researchers combine two techniques to shrink neural networks trained to detect sounds by 88%, with no loss in accuracy. One technique, distillation, involves using a large, powerful model to train a leaner, more-efficient one. The other technique, quantization, involves using a fixed number of values to approximate a larger range of values.
Sub-band Convolutional Neural Networks for Small-footprint Spoken Term Classification
Chieh-Chi Kao, Ming Sun, Yixin Gao, Shiv Vitaladevuni, Chao Wang
Convolutional neural nets (CNNs) were originally designed to look for the same patterns in every block of pixels in a digital image. But they can also be applied to acoustic signals, which can be represented as two-dimensional mappings of time against frequency-based “features”. By restricting an audio-processing CNN’s search only to the feature ranges where a particular pattern is likely to occur, the researchers make it much more computationally efficient. This could make audio processing more practical for power-constrained devices.
A Study for Improving Device-Directed Speech Detection toward Frictionless Human-Machine Interaction
Che-Wei Huang, Roland Maas, Sri Harish Mallidi, Björn Hoffmeister
This paper is an update of prior work on detecting device-directed speech, or identifying utterances intended for Alexa. The researchers find that labeling dialogue turns (distinguishing initial utterances from subsequent utterances) and using signal representations based on Fourier transforms rather than mel-frequencies improve accuracy. They also find that, among the features extracted from speech recognizers that the system considers, confusion networks, which represent word probabilities at successive sentence positions, have the most predictive power.
Automatic Speech Recognition (ASR)
Acoustic Model Bootstrapping Using Semi-Supervised Learning
Langzhou Chen, Volker Leutnant
The researchers propose a method for selecting machine-labeled utterances for semi-supervised training of an acoustic model, the component of an ASR system that takes an acoustic signal as input. First, for each training sample, the system uses the existing acoustic model to identify the two most probable word-level interpretations of the signal at each position in the sentence. Then it finds examples in the training data that either support or contradict those probability estimates, which it uses to adjust the uncertainty of the ASR output. Samples that yield significant reductions in uncertainty are preferentially selected for training.
Improving ASR Confidence Scores for Alexa Using Acoustic and Hypothesis Embeddings
Prakhar Swarup, Roland Maas, Sri Garimella, Sri Harish Mallidi, Björn Hoffmeister
Speech recognizers assign probabilities to different interpretations of acoustic signals, and these probabilities can serve as inputs to a machine learning model that assesses the recognizer’s confidence in its classifications. The resulting confidence scores can be useful to other applications, such as systems that select machine-labeled training data for semi-supervised learning. The researchers append embeddings — fixed-length vector representations — of both the raw acoustic input and the speech recognizer’s best estimate of the word sequence to the inputs to a confidence-scoring network. The result: a 6.5% reduction in equal-error rate (the error rate that results when the false-negative and false-positive rates are set as equal).
Multi-Dialect Acoustic Modeling Using Phone Mapping and Online I-Vectors
Harish Arsikere, Ashtosh Sapru, Sri Garimella
Multi-dialect acoustic models, which help convert multi-dialect speech signals to words, are typically neural networks trained on pooled multi-dialect data, with separate output layers for each dialect. The researchers show that mapping the phones — the smallest phonetic units of speech — of each dialect to those of the others offers comparable results with shorter training times and better parameter sharing. They also show that recognition accuracy can be improved by adapting multi-dialect acoustic models, on the fly, to a target speaker.
Neural Machine Translation for Multilingual Grapheme-to-Phoneme Conversion
Alex Sokolov, Tracy Rohlin, Ariya Rastrow
Grapheme-to-phoneme models, which translate written words into their phonetic equivalents (“echo” to “E k oU”), enable speech recognizers to handle words they haven’t seen before. The researchers train a single neural model to handle grapheme-to-phoneme conversion in 18 languages. The results are comparable to those of state-of-the-art single-language models for languages with abundant training data and better for languages with sparse data. Multilingual models are more flexible and easier to maintain in production environments.
Scalable Multi Corpora Neural Language Models for ASR
Anirudh Raju, Denis Filimonov, Gautam Tiwari, Guitang Lan, Ariya Rastrow
Language models, which compute the probability of a given sequence of words, help distinguish between different interpretations of speech signals. Neural language models promise greater accuracy than existing models, but they’re difficult to incorporate into real-time speech recognition systems. The researchers describe several techniques to make neural language models practical, from a technique for weighting training samples from out-of-domain data sets to noise contrastive estimation, which turns the calculation of massive probability distributions into simple binary decisions.
Natural-Language Understanding
Neural Named Entity Recognition from Subword Units
Abdalghani Abujabal, Judith Gaspers
Named-entity recognition is crucial to voice-controlled systems — as when you tell Alexa “Play ‘Spirit’ by Beyoncé”. A neural network that recognizes named entities typically has dedicated input channels for every word in its vocabulary. This has two drawbacks: (1) the network grows extremely large, which makes it slower and more memory intensive, and (2) it has trouble handling unfamiliar words. The researchers trained a named-entity recognizer that instead takes subword units — characters, phonemes, and bytes — as inputs. It offers comparable performance with a vocabulary of only 332 subwords, versus 74,000-odd words.
Dialogue Management
HyST: A Hybrid Approach for Flexible and Accurate Dialogue State Tracking
Rahul Goel, Shachi Paul, Dilek Hakkani-Tür
Dialogue-based computer systems need to track “slots” — types of entities mentioned in conversation, such as movie names — and their values — such as Avengers: Endgame. Training a machine learning system to decide whether to pull candidate slot values from prior conversation or compute a distribution over all possible slot values improves slot-tracking accuracy by 24% over the best-performing previous system.
Towards Universal Dialogue Act Tagging for Task-Oriented Dialogues
Shachi Paul, Rahul Goel, Dilek Hakkani-Tür
Dialogue-based computer systems typically classify utterances by “dialogue act” — such as requesting, informing, and denying — as a way of gauging progress toward a conversational goal. As a first step in developing a system that will automatically label dialogue acts in human-human conversations (to, in turn, train a dialogue-act classifier), the researchers create a “universal tagging scheme” for dialogue acts. They use this scheme to reconcile the disparate tags used in different data sets.
Topical-Chat: Towards Knowledge-Grounded Open-Domain Conversations
Karthik Gopalakrishnan, Behnam Hedayatnia, Qinlang Chen, Anna Gottardi, Sanjeev Kwatra, Anu Venkatesh, Raefer Gabriel, Dilek Hakkani-Tür
The researchers report a new data set, which grew out of the Alexa Prize competition and is intended to advance research on AI agents that engage in social conversations. Pairs of workers recruited through Mechanical Turk were given information on topics that arose frequently during Alexa Prize interactions and asked to converse about them, documenting the sources of their factual assertions. The researchers used the resulting data set to train a knowledge-grounded response generation network, and they report automated and human evaluation results as state-of-the-art baselines.
Text-to-Speech
Towards Achieving Robust Universal Neural Vocoding
Jaime Lorenzo Trueba, Thomas Drugman, Javier Latorre, Thomas Merritt, Bartosz Putrycz, Roberto Barra-Chicote, Alexis Moinet, Vatsal Aggarwal
A vocoder is the component of a speech synthesizer that takes the frequency-spectrum snapshots generated by other components and fills in the information necessary to convert them to audio. The researchers trained a neural-network-based vocoder using data from 74 speakers of both genders in 17 languages. The resulting “universal vocoder” outperformed speaker-specific vocoders, even on speakers and languages it had never encountered before and unusual tasks such as synthesized singing.
Fine-Grained Robust Prosody Transfer for Single-Speaker Neural Text-to-Speech
Viacheslav Klimkov, Srikanth Ronanki, Jonas Rohnke, Thomas Drugman
The researchers present a new technique for transferring prosody (intonation, stress, and rhythm) from a recording to a synthesized voice, enabling the user to choose whose voice will read recorded content, with inflections preserved. Where earlier prosody transfer systems used spectrograms — frequency spectrum snapshots — as inputs, the researchers’ system uses easily normalized prosodic features extracted from the raw audio.
Machine Learning
Two Tiered Distributed Training Algorithm for Acoustic Modeling
Pranav Ladkat, Oleg Rybakov, Radhika Arava, Sree Hari Krishnan Parthasarathi,I-Fan Chen, Nikko Strom
When neural networks are trained on large data sets, the training needs to be distributed, or broken up across multiple processors. A novel combination of two state-of-the-art distributed-learning algorithms — GTC and BMUF — achieves both higher accuracy and more-efficient training then either, when learning is distributed to 128 parallel processors.
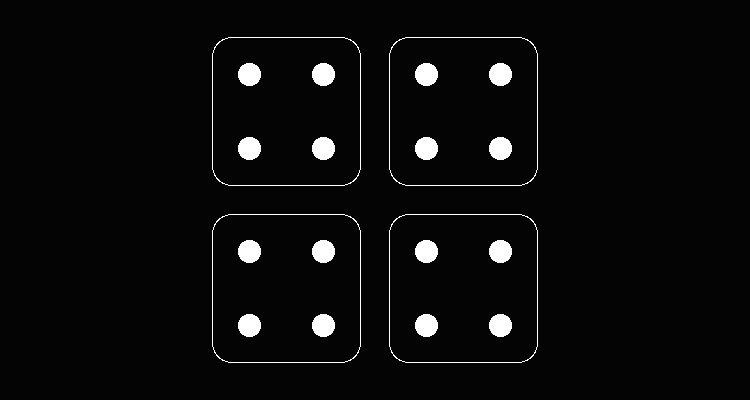
One-vs-All Models for Asynchronous Training: An Empirical Analysis
Rahul Gupta, Aman Alok, Shankar Ananthakrishnan
A neural network can be trained to perform multiple classifications at once: it might recognize multiple objects in an image, or assign multiple topic categories to a single news article. An alternative is to train a separate “one-versus-all” (OVA) classifier for each category, which classifies data as either in the category or out of it. The advantage of this approach is that each OVA classifier can be re-trained separately as new data becomes available. The researchers present a new metric that enables comparison of multiclass and OVA strategies, to help data scientists determine which is more useful for a given application.