This week, at the Association for Computing Machinery’s annual conference on Knowledge Discovery and Data Mining (KDD), Thorsten Joachims, a Cornell University professor of computer science and an Amazon Scholar, received the conference’s Innovation Award. SIGKDD, the ACM special-interest group that organizes the conference, describes the award as “the highest honor for technical excellence in the field of knowledge discovery and data mining”.
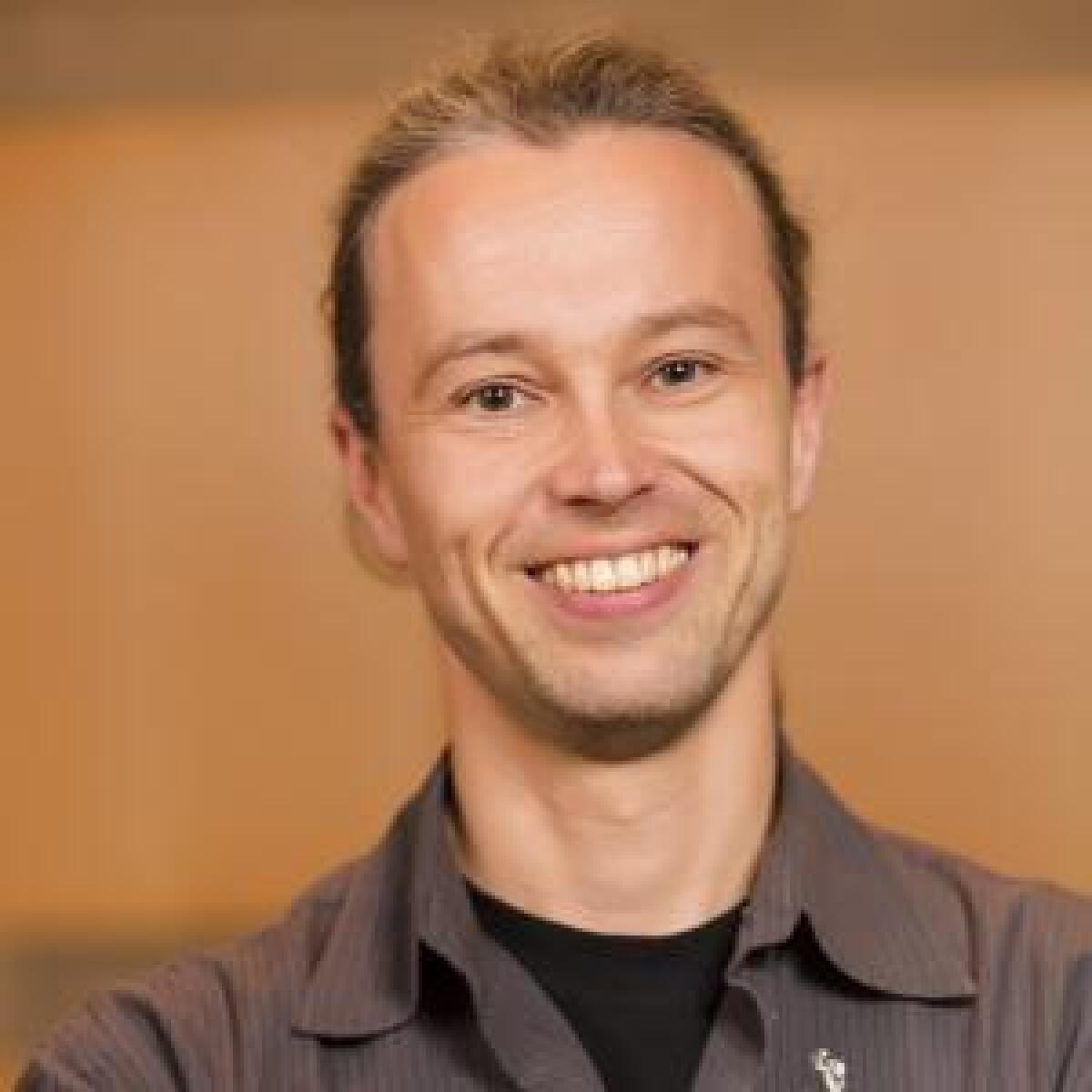
The award citation acknowledges Joachims’s “influential work studying human biases in information retrieval, SVM [support vector machines], and structured output prediction” and particularly his “methods for eliciting reliable preferences from implicit feedback, methods for unbiased learning-to-rank, and ranking methods that provide fairness guarantees.”
Amazon Science asked Joachims three questions about the work that earned the award — particularly as it pertains to his research at Amazon.
Q: What is the problem of “human biases in information retrieval”?
Much of my work has been about trying to learn from human behavior, particularly in systems that provide rankings or that provide recommendations. A great source of feedback that these systems provide is whether someone clicks on a result or reformulates a query and eventually consumes something. That provides a lot of data, and unlike more traditional ways of training these system, it’s not what some expert thinks is relevant to this query. It actually reflects what the users think — what’s the right answer to that query or what’s helpful to them.
The problem with learning from this implicit feedback is that the system biases how people behave. Things that are ranked highly will get a lot more exposure than things that are way down below, and that also affects what people can click or what people will purchase. So by the actions that the system takes, it is also contaminating the data. Something in position one gets the most clicks, so it stays in position one. It becomes this self-reinforcing cycle, which means that something that’s pretty bad can stay in position one while something that’s pretty good never gets discovered.
The question is, how do you deal with the biases that the systems introduce? One general insight is to view these systems as agents that interact with people: they’re not just collecting data; they’re taking an action, like making a recommendation. And what we observe is how people react to that intervention.
This means that system is a lot like a controlled randomized trial in medicine. You give a treatment to a patient, and you see how the patient reacts to that treatment, but you don’t get to see what would have happened if you had given that patient another treatment.
The same with a recommender system or ranker system: you see what happens if you make a particular intervention — recommend this movie, and the person watches it or not — but you don’t get to see what would have happened if you had recommended a different item. What we’ve brought to recommender systems is this idea that you want to treat them in the same way, from a statistical perspective, as a controlled randomized trial.
Now, in some ways this problem is easier than in medicine. We get a lot more data, and there’s a lot less risk. But in some sense it’s also harder. In medicine, you might have three different treatments, whereas in recommender systems, every item in your database is a potential treatment. We have millions of them, so dealing with complexity and the scale of the problem is challenging.
Something in position one gets the most clicks, so it stays in position one. It becomes this self-reinforcing cycle.
There are two ways you can approach this problem: the online way and the offline way. In the online way, you constantly try out new interventions, see how people react, and then tweak your policy step by step, always interactively running these experiments. That’s called online learning and, in particular, contextual-bandit online learning
In a sense, online learning is wasteful, and it potentially has a negative impact on the customer, in that you’re potentially trying out things multiple times that are not actually that good.
But we already have terabytes of existing data where we know we’ve taken that action in this context for that customer, and the customer was happy. Can we recycle all of this old data and use that for machine learning, instead of trying things out over and over again?
One of the things we’ve developed are these batch learning methods, which you can think about as learning from a controlled randomized trial in hindsight. Once you have the data, ask the question, “What would have been the best policy if I were able to rewind time and go back to when the data was collected?” I think these offline algorithms are particularly promising.
Excited to announce 2020 ACM SIGKDD Innovation Award is given to Thorsten Joachims (Cornell University) for his research contributions in machine learning, including influential work studying human biases in information retrieval, SVM, and structured output prediction. pic.twitter.com/6wy1RjFcCT
— SIGKDD 2020 (@kdd_news) August 13, 2020
Q: Do you use the same approach in the “learning-to-rank” setting mentioned in the award citation?
Learning to rank is one particular type of feedback. Contextual bandit is more like, you ask Alexa to play music, and Alexa has to play something for you. It picks exactly one action: it plays one track, and the user liked it or didn’t like it. The ranking setting is a little more forgiving. You present a ranking of items, so even if you don’t nail the top one, you can still get feedback if the user is patient and goes down the ranking.
But if the user doesn’t click on something, that could have two causes. One cause is the user didn’t like it. The other cause is that the user just didn’t see it; the user didn’t go far enough down to discover that item.
So the additional complication, compared to the contextual-bandit setting, is that you have to tease apart this ambiguity. We’ve come up with techniques where you can at least in expectation tease these two causes apart. Even though you can’t do it for any individual impression, you can say, “In expectation, I know that lack of seeing an item is responsible for that many missing clicks, and lack of relevance is responsible for the rest.” So similar techniques that come from controlled randomized trials can also be brought to bear on this problem.
Q: What is “structured output prediction”, which the citation also mentions?
Many machine learning problems are formulated as binary classifications — predicting yes or no — or as regression problems, where you just predict a number — 5.7 or something.
But for many other problems, you’re predicting a structured object. Rankings are an example of a structured object, where the thing that you’re predicting is a combinatorial object. It’s a permutation.
You want to model dependencies in this ranking. For example, if you have the query “Michael Jordan”, that’s ambiguous. It could mean the basketball player; it could mean the statistician; it could mean the actor.
Maybe the basketball player is the most likely interpretation, but filling your top 10 results only with links about the basketball player is probably not the right thing to do, because not everybody was looking for that.
You want to model dependencies: if I put the first links about the basketball player, what’s the best thing to put next? Maybe the next most popular intent is the actor. You want to make this prediction of what you put into the ranking as a prediction of all these items that are dependent on each other.
That gives you these machine learning problems where the thing that you’re predicting is one element of this huge combinatorial space of all possible permutations over documents, which are more than there are atoms in the universe. And you still want to learn these models, and you want to efficiently compute what’s the best ranking to present.
That’s a problem that’s relevant to Amazon. It’s also relevant to a lot of other problems, like predicting the structure of a protein. You have the sequence, and you want to predict how it folds. You really have to model all the dependencies, how things interact in the protein.
Or it’s relevant to natural-language processing — predicting the constituents of the semantic parse of a sentence, for example. You need to take into account how all of the constituents of the sentence relate to each other. So it’s really relevant to a lot of prediction problems.