Last year, Amazon announced the beta release of Alexa Guard, a new service that lets customers who are leaving the house instruct their Echo devices to listen for glass breaking or smoke and carbon dioxide alarms going off.
At this year’s International Conference on Acoustics, Speech, and Signal Processing, our team is presenting several papers on sound detection. I wrote about one of them a few weeks ago, a new method for doing machine learning with unbalanced data sets.
Today I’ll briefly discuss two others, both of which, like the first, describe machine learning systems. One paper addresses the problem of media detection, or recognizing when the speech captured by a digital-assistant device comes from a TV or radio rather than a human speaker. In particular, we develop a way to better characterize media audio by examining longer-duration audio streams versus merely classifying short audio snippets. Media detection helps filter a particularly deceptive type of background noise out of speech signals.
For our other paper, we used semi-supervised learning to train a system developed from an external dataset to do acoustic-event detection. Semi-supervised learning uses small sets of annotated training data to leverage larger sets of unannotated data. In particular, we use tri-training, in which three different models are trained to perform the same task, but on slightly different data sets. Pooling their outputs corrects a common problem in semi-supervised training, in which a model’s errors end up being amplified.
Our media detection system is based on the observation that the audio characteristics we would most like to identify are those common to all instances of media sound, regardless of content. Our network design is an attempt to abstract away from the properties of particular training examples.
Like many machine learning models in the field of spoken-language understanding, ours uses recurrent neural networks (RNNs). An RNN processes sequenced inputs in order, and each output factors in the inputs and outputs that preceded it.
We use a convolutional neural network (CNN) as feature extractor, and stack RNN layers on top of it. But each RNN layer has only a fraction as many nodes as the one beneath it. That is, only every third or fourth output from the first RNN provides an input to the second, and only every third or fourth output of the second RNN provides an input to the third.

Because the networks are recurrent, each output we pass contains information about the outputs we skip. But this “pyramidal” stacking encourages the model to ignore short-term variations in the input signal.
For every five-second snippet of audio processed by our system, the pyramidal RNNs produce a single output vector, representing the probabilities that the snippet belongs to any of several different sound categories.
But our system includes still another RNN, which tracks relationships between five-second snippets. We experimented with two different ways of integrating that higher-level RNN with the pyramidal RNNs. In the first, the output vector from the pyramidal RNN simply passes to the higher-level RNN, which makes the final determination about whether media sound is present.
In the other, however, the higher-level RNN lies between the middle and top layers of the pyramidal RNN. It receives its input from the middle layer, and its output, along with that of the middle layer, passes to the top layer of the pyramidal RNN.
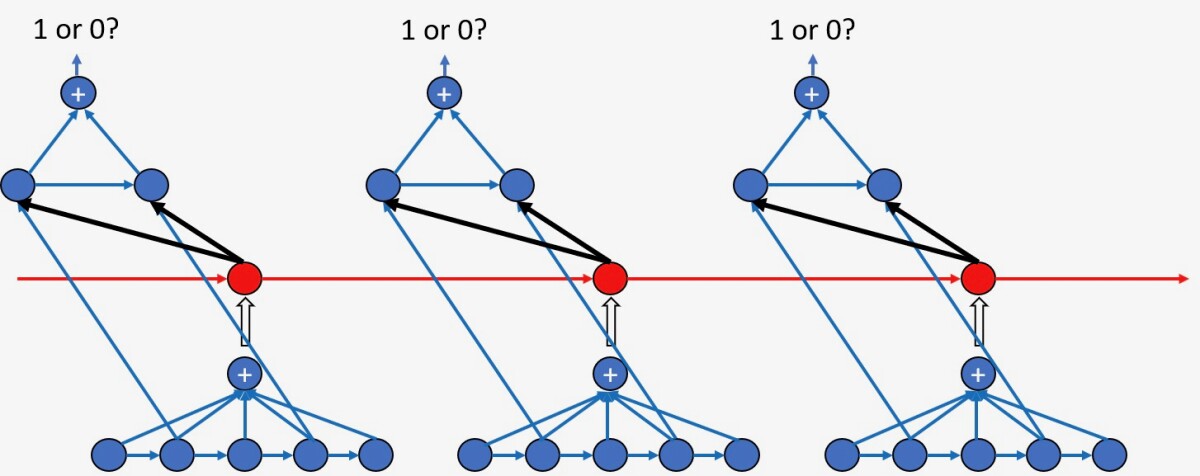
This was our best-performing model. When compared to a model that used the pyramidal RNNs but no higher-level RNN, it offered a 24% reduction in equal error rate, which is the error rate that results when the system parameters are set so that the false-positive rate equals the false-negative rate.
Our other ICASSP paper presents our semi-supervised approach to acoustic-event detection (AED). One popular and simple semi-supervised learning technique is self-training, in which a machine learning model is trained on a small amount of labeled data and then itself labels a much larger set of unlabeled data. The machine-labeled data is then sorted according to confidence score — the system’s confidence that its labels are correct — and data falling in the right confidence window is used to fine-tune the model.
The model, that is, is retrained on data that it has labeled itself. Remarkably, this approach tends to improve the model’s performance.
But it also poses a risk. If the model makes a systematic error, and if it makes it with high confidence, then that error will feed back into the model during self-training, growing in magnitude.
Tri-training is intended to mitigate this kind of self-reinforcement. In our experiments, we created three different training sets, each the size of the original — 39,000 examples — by randomly sampling data from the original. There was substantial overlap between the sets, but in each, some data items were oversampled, and some were undersampled.
We trained neural networks on all three data sets and saved copies of them, which we might call initial models. Then we used each of those networks to label another 5.4 million examples. For each of the initial models, we used machine-labeled data to re-train it only if both of the other models agreed on the labels with high confidence. In all, we retained only 5,000 examples out of the more than five million in the unlabeled data set.
Finally, we used six different models to classify the examples in our test set: the three initial models and the three retrained models. On samples of three sounds — dog sounds, baby cries, and gunshots — pooling the results of all six models led to reductions in equal-error rate (EER) of 16%, 26%, and 19%, respectively, over a standard self-trained model.
Of course, using six different models to process the same input is impractical, so we also trained a seventh neural network to mimic the aggregate results of the first six. On the test set, that network was not quite as accurate as the six-network ensemble, but it was still a marked improvement over the standard self-trained model, reducing EER on the same three sample sets by 11%, 18%, and 6%, respectively.
Acknowledgments: Qingming Tang, Chieh-Chi Kao, Viktor Rozgic, Bowen Shi, Spyros Matsoukas, Chao Wang