Matthias Seeger, a principal applied scientist in Amazon’s Berlin office, and three coauthors have received the ICML 2020 test of time award for a paper from ten years ago that has had a significant impact within the machine learning field, including both research and practice. The award was announced on July 1; the recipients will give a plenary talk at the virtual conference on July 13.
The paper, Gaussian Process Optimization in the Bandit Setting: No Regret and Experimental Design, forged durable links between previously separate domains, Bayesian optimization (BO), linear contextual multi-armed bandits (MAB), and Bayesian experimental design (BED), says Seeger.
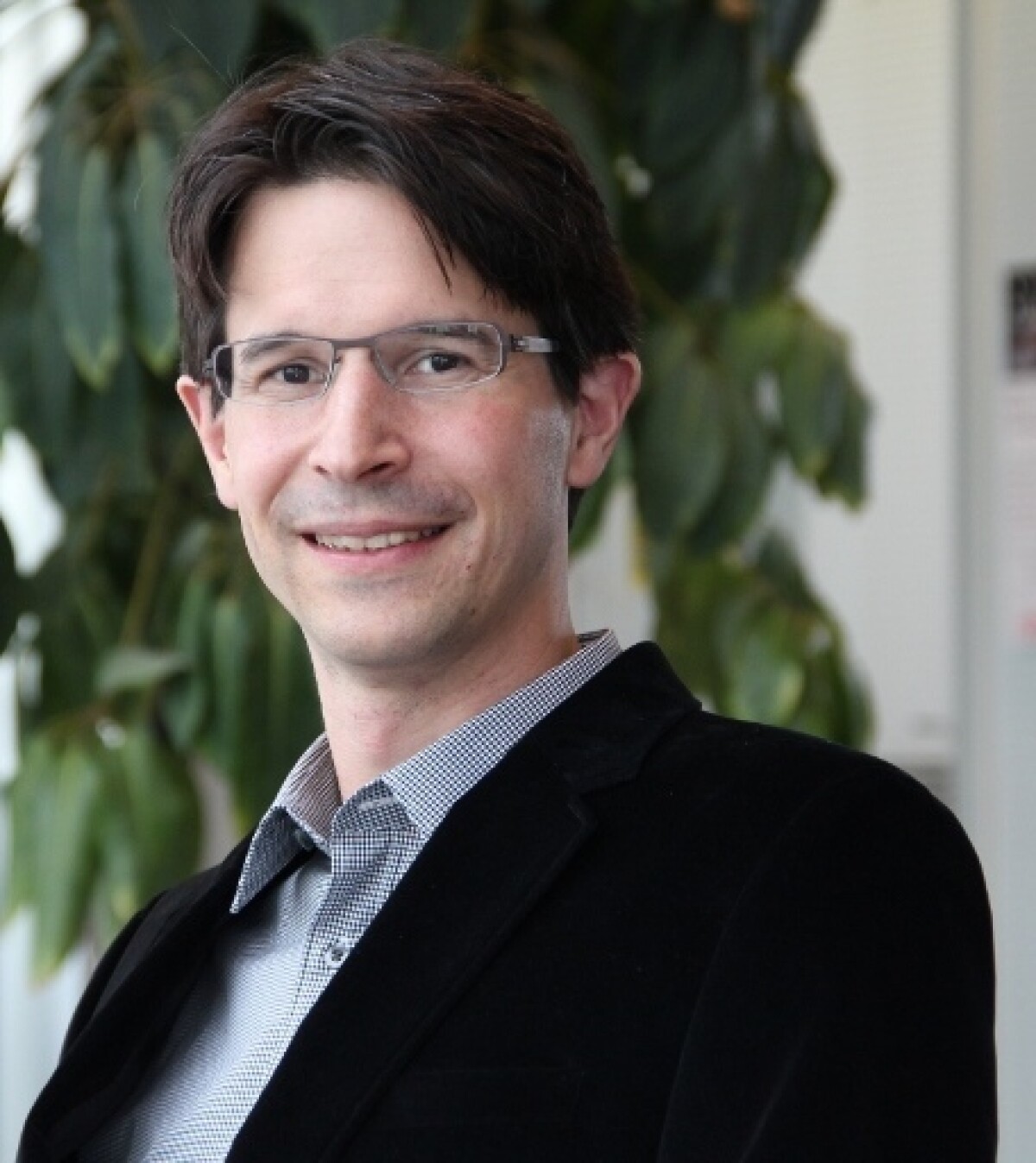
“Our work has enabled a sound theoretical basis for Bayesian optimization, and has since cross-fertilized the separate research domains of MABs, BO, and BED,” he said.
Seeger’s coauthors were Niranjan Srinivas, now a computational biologist at 10xGenomics; Andreas Krause, now a professor of computer science at ETH Zurich; and Sham Kakade, now a professor in the departments of computer science and statistics at the University of Washington.
The award-winning paper was selected by ICML General Chair David Blei and Program Co-Chairs Aarti Singh and Hal Daumé III, in consultation with an award committee.
In announcing the award, they said: “This paper brought together the fields of Bayesian optimization, bandits and experimental design by analyzing Gaussian process bandit optimization, giving a novel approach to derive finite-sample regret bounds in terms of a mutual information gain quantity. This paper has had profound impact over the past ten years, including the method itself, the proof techniques used, and the practical results. These have all enriched our community by sparking creativity in myriad subsequent works, ranging from theory to practice.”
We are very pleased to announce that the #icml2020 Test of Time award goes to
— Hal Daumé III (@haldaume3) July 1, 2020
Gaussian Process Optimization in the Bandit Setting: No Regret and Experimental Design
by Niranjan Srinivas, Andreas Krause, Sham Kakade and Matthias Seegerhttps://t.co/kDuE8kwBx6
> pic.twitter.com/UWG3NiBJ5i
Seeger has been a scientist at Amazon for more than six years; he is one of the principal scientists behind Amazon SageMaker, an AWS service that provides every developer and data scientist with the ability to build, train, and deploy machine learning models quickly. He’s also contributed to science and technology behind Automatic Model Tuning and Amazon SageMaker Autopilot.
Seeger serves as ICML 2020 Newcomers co-chair and in the Best Paper Award committee. He continues to publish, as well.
At this year’s ICML AutoML Workshop, he and coauthors Eric Hans Lee, Valerio Perrone, and Cedric Archambeau will present the paper Cost-aware Bayesian Optimization. In the paper’s abstract the authors say: “While the standard cost-aware heuristic in the black-box setting is to normalize the acquisition function by the cost, we show that this often underperforms, and adopt a different approach by scheduling cheaper evaluations before expensive ones. We do so through two improved heuristics: a cost-effective initial design and a cost-cooled optimization phase which depreciates a learned cost model as iterations proceed.”
ICML 2020 takes place July 12-18. Initially scheduled for Vienna, Austria, the conference will be a fully virtual event. More information about Amazon’s presence at ICML 2020 will be available soon on our conference page.