As climate change, in tandem with accelerating population growth, becomes an increasingly critical issue, the marriage of environmentalism and data-driven intelligence has never been more necessary. This is the intersection where 20tree.ai exists, with a declared mission to deliver actionable planet intelligence.
Their work in short: creating snapshots of the Earth in real-time. In essence, a digital twin of the Earth to better understand its natural resources, specifically its forests and how humans can be more responsible to them. They are accomplishing this by ingesting large volumes of satellite imagery and data, analyzing it through AI techniques like computer vision, and then breaking down this information into operative insights for their customers in a digestible and actionable manner.
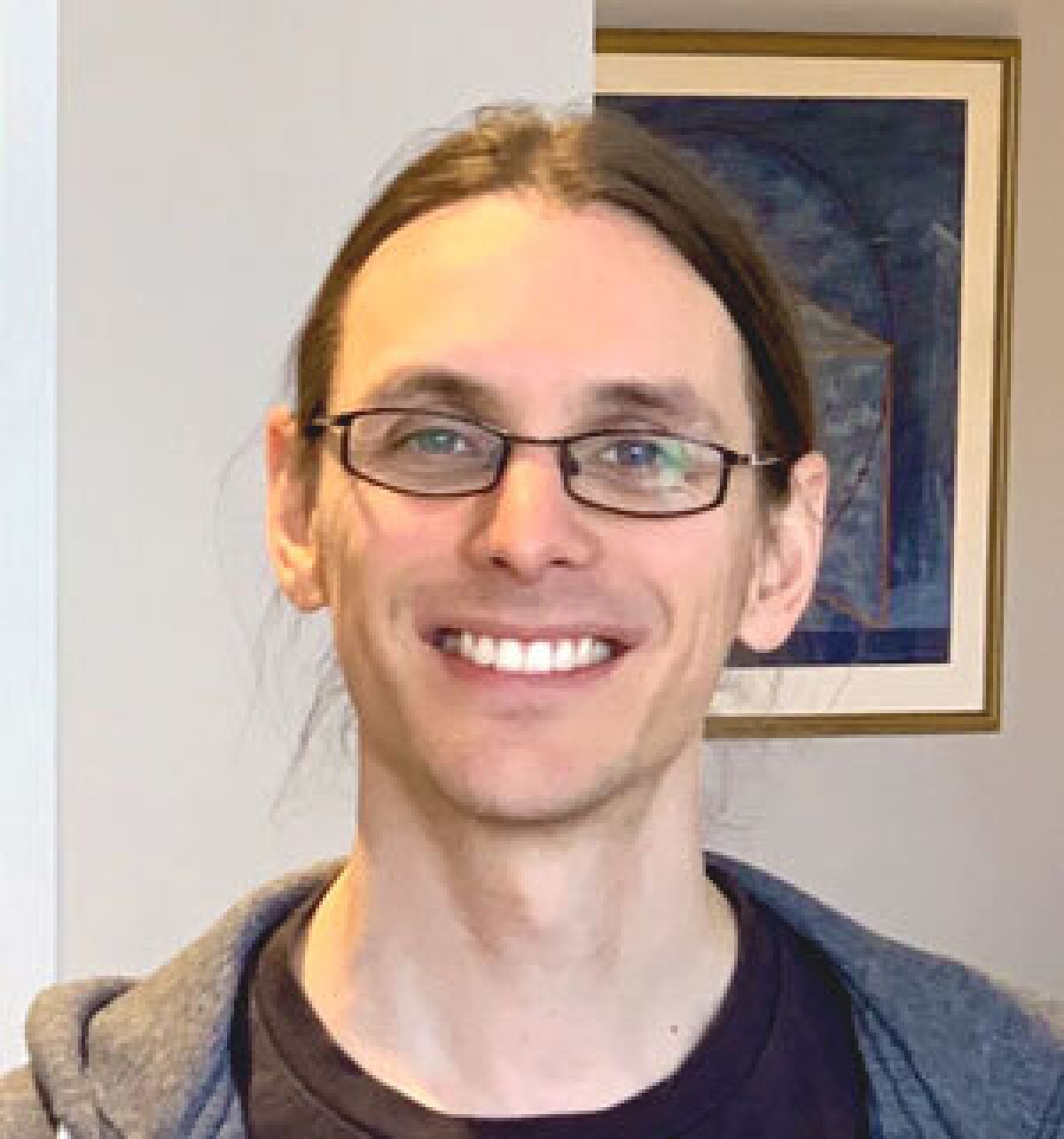
“Trees are still the best technology we have to sequester carbon,” Roelof Pieters, 20tree.ai co-founder and chief technology officer explains. “They’re 1,000-times more cost effective right now than any kind of technical solution.”
20tree.ai’s data challenges
Like many startups, 20tree.ai faced a number of data questions from the get-go.
How do they deal with massive amounts of data pouring in? Where do they store it? How do they make it accessible to themselves and their clients? How do they accomplish all of this in a cost-effective way? And these questions were further complicated by the type of data they work with — high-resolution satellite imagery and radar data.
While first operating off their own hardware, 20tree.ai eventually moved into Amazon’s startup program to help build up their infrastructure. Amazon S3, an object storage service, allows them to serve encrypted insights to their clients, providing them with a simple solution to replicate data across different regions.
Trees are still the best technology we have to sequester carbon. They’re 1,000-times more cost effective right now than any kind of technical solution.
And when it comes to heavy lifting for AI, Amazon EFS (Elastic File Storage), a cloud storage service, has proven to be a life saver for them, providing what Pieters described as like having “a hard disk of unlimited size.”
Applying computer vision
Looking a layer deeper, because forests and atmospheric conditions are always changing, the kind of data that is pulled is, from a computer vision perspective, very noisy. In order to accommodate these changes, 20tree.ai needs flexibility in its infrastructure and data pipeline.
While satellite imagery allows them to pull images rapidly on a massive scale, the maximum resolution can only provide so much information accurately. It’s one thing to be able to pull insights from data, but it’s even more important to be able to understand that you are pulling the right insights from your data, and computer vision makes this possible without having boots on the ground.
For image classification, 20tree.ai relies on deep learning algorithms. By applying a convolutional neural network to satellite images, they can figure out an image’s shape and exact location, and determine things like if an image is cloudy or not. 20tree.ai finds image segmentation networks are helpful for land cover classification, because they can partition shapes and objects, like trees, roads and buildings.
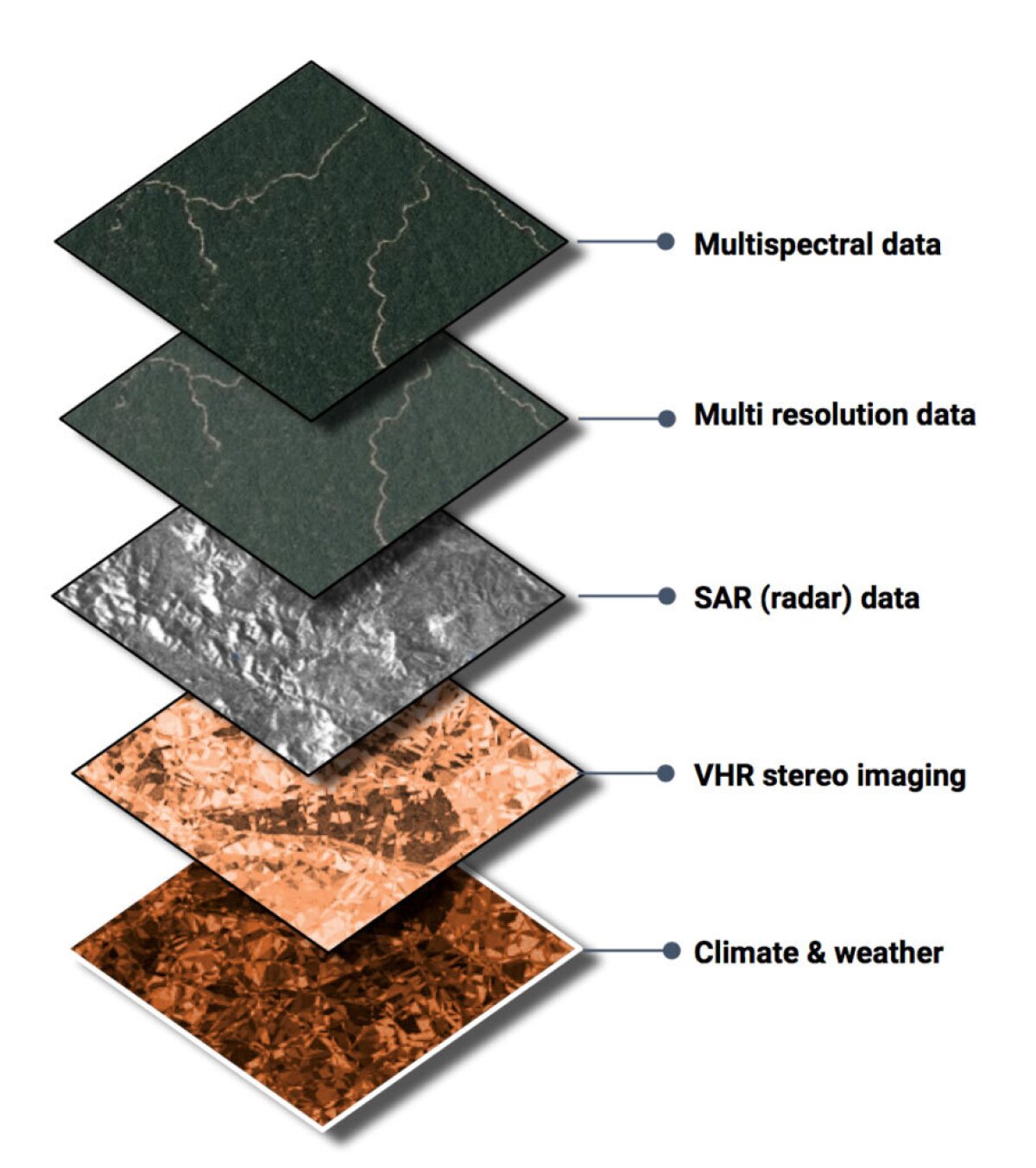
In order to enable the custom builds of their AI algorithms for satellite imagery, 20tree.ai employs Amazon SageMaker, a fully-managed machine learning service, to help put their various custom trained models into production and easily deploy them in a scalable way. As Pieters notes, using SageMaker means they don't have to worry about the specifics of where and how their models are exposed as scalable rest points internally for their products, as well as externally.
Because of this, 20tree.ai can train its algorithms with satellite images from different locations and points in time, to predict high risk areas and detect deforestation. With most companies in their field still relying solely on traditional AI techniques, 20tree.ai’s application of the latest advancements in computer vision provides them with a significant competitive advantage.
Actionable intelligence for the planet
Partnering primarily with large enterprises, 20tree.ai’s work touches on a variety of initiatives — helping denote deforestation patterns, improving productivity on farms, monitoring urban green spaces, and catching risks to forests like insect plagues or drought.
One major focus of their work has centered around managing the interaction between vegetation and power lines, with several large electric utilities companies across the U.S. and Europe as clients. In areas like California that have seen consistent issues with forest fires, tree interaction with power lines are one of the leading causes of these instances.
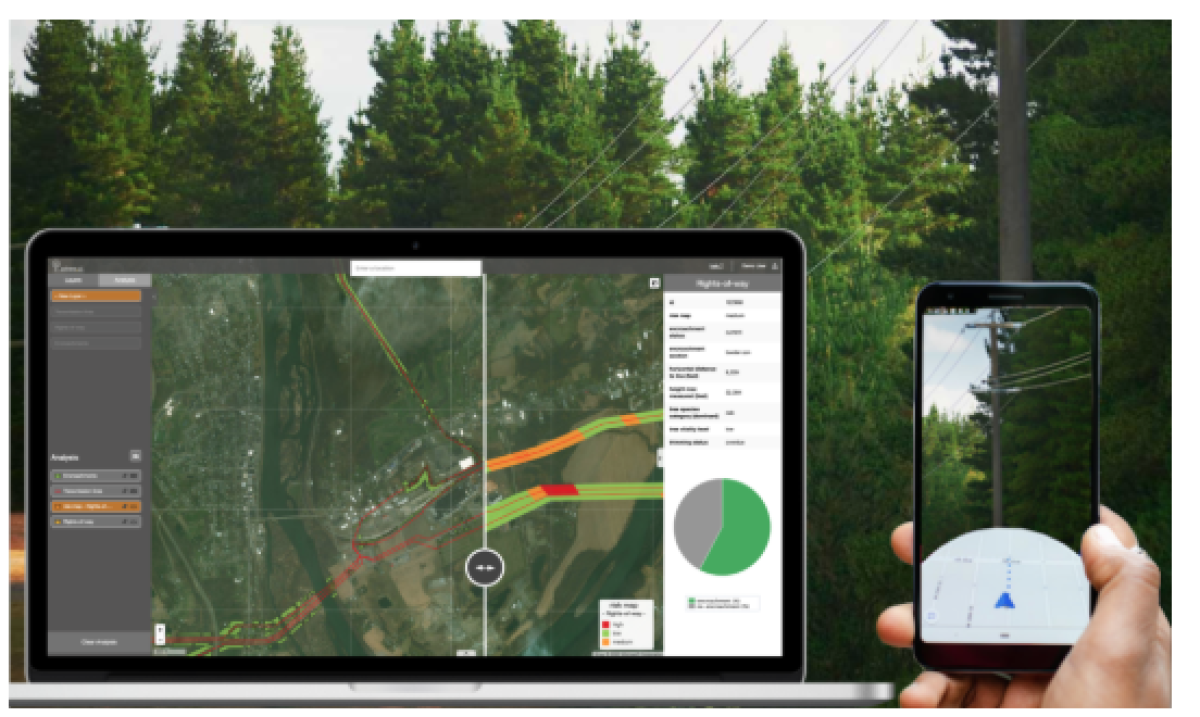
Utilities companies have been spending lots of money to try to prevent this from continuing, but the tools they’ve had to this point — drones, helicopters, people on the ground — have been inefficient relative to the size of lands they are attempting to manage. It can take them years to get a snapshot of their powerline infrastructure, and by the time they do that information is often outdated.
20Tree.ai’s use of satellite imagery makes it possible to conduct this work every single day, and while it can’t provide the same level of image resolution as drones or helicopters, computer vision makes it possible to provide a complementary level of insights needed to make critical decisions. They can see exactly what types of trees are standing where, revealing the current heights of trees near powerlines, whether certain trees are more or less of a hazard because of the pace at which they grow, where there are protected species of trees, any storm damage that might have occurred, and more.
With these insights in hand, utilities companies are able to identify the hotspots that need their attention, reducing surveillance costs by up to 50%, according to Pieters. Down the line, proactive planning like risk-based tree trimming cycles and verified vegetation maintenance (being able to check up and prove that this work is being done) becomes a lot easier, ultimately making risk reduction more quantifiable.