With billions of customer orders flowing through Amazon’s global network of fulfillment centers (FCs) every year, it is an unfortunate but inevitable fact that some of those items will suffer accidental damage during their journey through a warehouse.
Amazon associates are always on the lookout for damaged items in the FC, but an extra pair of “eyes” may one day support them in this task, powered by machine-learning approaches being developed by Amazon’s Robotics AI team in Berlin, Germany.
As well as avoiding delays in shipping and improving warehouse efficiency, this particular form of artificial intelligence has the benefit of aiming to reduce waste by shipping fewer damaged goods in the first place, ensuring customers have fewer damaged items to return.
For every thousand items that make their way through an FC prior to being dispatched to the customer, fewer than one becomes damaged. That is a tiny proportion, relatively speaking, but working at the scale of Amazon this nevertheless adds up to a challenging problem.
Damage detection is important because while damage is a costly problem in itself, it becomes even more costly the longer the damage goes undetected.
Amazon associates examine items at multiple occasions through the fulfillment process, of course, but if damage occurs late in the journey and a compromised item makes it as far as the final packaging station, an associate must sideline it so that a replacement can be requested, potentially delaying delivery. As associate must then further examine the sidelined item to determine its future.
Toward the end of 2020, Sebastian Hoefer, senior applied scientist with the Amazon Robotics AI team, supported by his Amazon colleagues, successfully pitched a novel project to address this problem. The idea: combine computer vision and machine learning (ML) approaches in an attempt to automate the detection of product damage in Amazon FCs.
“You want to avoid damage altogether, but in order to do so you need to first detect it,” notes Hoefer. “We are building that capability, so that robots in the future will be able to utilize it and assist in damage detection.”
Needles in a haystack
Damage detection is a challenging scientific problem, for two main reasons.
Damage caused in Amazon FCs is rare, and that’s clearly a good thing. But that also makes it challenging because we need to find these needles in the haystack, and identify the many forms damage can take.
The first reason is purely practical — there is precious little data on which to train ML models.
“Damage caused in Amazon FCs is rare, and that’s clearly a good thing,” says Ariel Gordon, a principal applied scientist supporting Hoefer’s team from Seattle. “But that also makes it challenging because we need to find these needles in the haystack, and identify the many forms damage can take.”
The second reason takes us into the theoretical long grass of artificial intelligence more generally.
For an adult human, everyday damage detection feels easy — we cannot help but notice damage, because our ability to do so has been honed as a fundamental life skill. Yet whether something is sufficiently damaged to render it unsellable is subjective, often ambiguous, and depends on the context, says Maksim Lapin, an Amazon senior applied scientist in Berlin. “Is it damage that is tolerable from the customer point of view, like minor damage to external packaging that will be thrown into the recycling anyway?” Lapin asks. “Or is it damage of a similar degree on the product itself, which would definitely need to be flagged?”
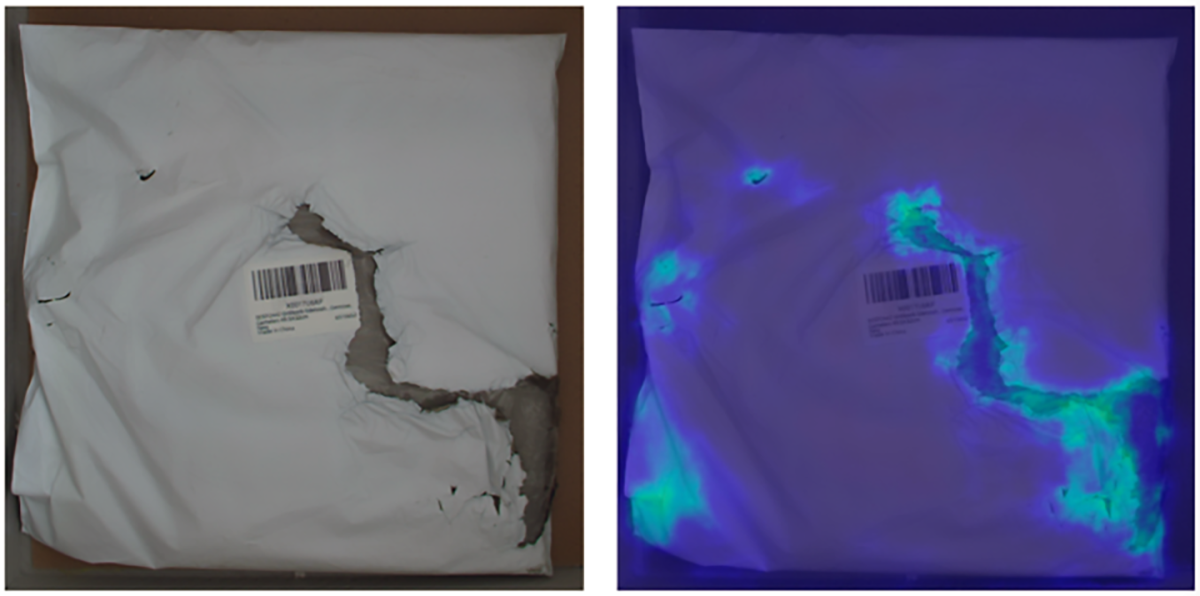
In addition, the nature of product damage makes it difficult to even define what damage is for ML models. Damage is both heterogenous — any item or product can be damaged — and can take many forms, from rips to holes to a single broken part of a larger set. Multiplied over Amazon’s massive catalogue of items, the challenge becomes enormous.
In short, do ML models stand a chance?
Off to “Damage Land”
To find out, Hoefer’s team first needed to obtain that data in a standardized format amenable to machine learning. They set about collecting it at an FC near Hamburg, Germany, called HAM2, in a section of the warehouse affectionately known as “Damage Land”. Damaged items end up there while decisions are made on whether such items can be sold at a discount, refurbished, donated or, as a last resort, disposed of.
The team set up a sensor-laden, illuminated booth in Damage Land.
“I’m very proud that HAM2 was picked up as pilot site for this initiative,” says Julia Dembeck, a senior operations manager at HAM2, who set up the Damage Taskforce to coordinate the project’s many stakeholders. “Our aim was to support the project wholeheartedly.”
After workshops with Amazon associates to explain the project and its goals, associates started placing damaged items on a tray in the booth, which snapped images using an array of RGB and depth cameras. They then manually annotated the damage in the images using a linked computer terminal.
“The results were amazing and got even better when associates shared their best practices on the optimal way to place items in the tray,” says Dembeck. Types of damage included things like crushes, tears, holes, deconstruction (e.g., contents breaking out from its container) and spillages.
The associates collected about 30,000 product images in this way, two-thirds of which were images of damaged items.
“We also collected images of non-damaged items because otherwise we cannot train our models to distinguish between the two,” says Hoefer. “Twenty thousand pictures of damage are not a lot in ‘big data’ terms, but it is a lot given the rarity of damage.”
With data in hand, the team first applied a supervised learning ML approach, a workhorse in computer vision. They used the data as a labelled training set that would allow the algorithm to build a generalizable model of what damage can look like. When put through its paces on images of products it had never seen before, the model’s early results were promising.
When analyzing a previously unseen image of a product, the model would ascribe a damage confidence score. The higher the score, the more confident it was that the item was damaged.
The researchers had to tune the sensitivity of the model by deciding upon the confidence threshold at which the model would declare a product unfit for sending to a customer. Set that threshold too high, and modest but significant damage could be missed. Set it too low, and the model would declare some undamaged items to be damaged, a false positive.
“We did a back-of-the-envelope calculation and found that if we're sidelining more than a tiny fraction of all items going through this process, then we're going to overwhelm with false positives,” says Hoefer.
Since those preliminary results in late 2021, the team has made significant improvements.
“We’re now optimizing the model to reduce its false positive rate, and our accuracy is increasing week to week,” says Hoefer.
Different types of damage
However, the supervised learning approach alone, while promising, suffers some drawbacks.
For example, what is the model to make of the packaging of a phone protector kit that shows a smashed screen? What is it to make of a cleaning product whose box is awash with apparent spills? What about a blister pack that is entirely undamaged and should hold three razor blades but for some reason contains just two — the “broken set” problem? What about a bag of ground coffee that appears uncompromised but is sitting next to a little puddle of brown powder?
Again, for humans, making sense of such situations is second nature. We not only know what damage looks like, but also quickly learn what undamaged products should look like. We learn to spot anomalies.
Hoefer’s team decided to incorporate this ability into their damage detection system, to create a more rounded and accurate model. Again, more data was needed, because if you want to know what an item should look like, you need standardized imagery of it. This is where recent work pioneered by Amazon’s Multimodal Identification (MMID) team, part of Berlin's Robotics AI group, came in.
The MMID team has developed a computer vision tool that enables the identification of a product purely from images of it. This is useful in cases where the all-important product barcode is smudged, missing, or wrong.
In fact, it was largely the MMID team that developed the sensor-laden photo booth hardware now being put to use by Hoefer’s team. The MMID team needed it to create a gallery of standardized reference images of pristine products.
“Damage detection could also exploit the same approach by identifying discrepancies between a product image and a gallery of reference images,” says Anton Milan, an Amazon senior applied scientist who is working across MMID and damage detection in Berlin. “In fact, our previous work on MMID allowed us to quickly take off exploring this direction in damage detection by evaluating and tweaking existing solutions.”
By incorporating the MMID team’s product image data and adapting that team’s techniques and models to sharpen their own, the damage-detection system now has a fighting chance of spotting broken sets. It is also much less likely to be fooled by damage-like images printed on the packaging of products, because it can check product imagery taken during the fulfillment process against the image of a pristine version of that product.
“Essentially, we are developing the model’s ability to say ‘something is amiss here’, and that’s a very useful signal,” says Gordon. “It's also problematic, though, because sometimes products change their design. So, the model has to be ‘alive’, continuously learning and updating in accordance with new packaging styles.”
The team is currently exploring how to combine the contributions of both discriminative and anomaly-based ML approaches to give the most accurate assessment of product damage. At the same time, they are developing hardware for trial deployment in an FC, and also collecting more data on damaged items.
The whole enterprise has come together fast, says Hoefer. “We pitched the idea just 18 months ago, and already we have an array of hardware and a team of 15 people making it a reality. As a scientist, this is super rewarding. And if it works as well as we hope, it could be sitting in across the network of Amazon fulfillment centers within a couple of years.”
Hoefer anticipates that the project will ultimately improve customer experience while also reducing waste.
“Once the technology matures, we expect to see a decrease in customer returns due to damage, because we will be able to identify and fix damaged products before dispatching them to customers. Not only that, by identifying damage early in the fulfillment chain, we will be able to work with vendors to build more robust products. This will again result in reducing damage overall — an important long-term goal of the project,” says Hoefer.
Also looking to the future, Lapin imagines this technology beyond warehousing.
“We are building these capabilities for the highly controlled environments of Amazon fulfillment centers, but I can see some future version of it being deployed in the wild, so to speak, in more chaotic bricks-and-mortar stores, where customers interact with products in unpredictable ways,” says Lapin.