- Building machine learning models with encrypted data
At the Workshop on Encrypted Computing and Applied Homomorphic Cryptography, Amazon researchers presented a paper exploring the application of homomorphic encryption to logistic regression, a statistical model used for myriad machine learning applications, from genomics to tax compliance. Learn how this new approach to homomorphic encryption speeds up the training of encrypted machine learning models sixfold.
- Improving explainable AI’s explanations
Mohammad Taha Bahadori and David Heckerman presented a paper at the International Conference on Learning Representations, where they "adapt a technique for removing confounders from causal models, called instrumental-variable analysis, to the problem of concept-based explanation." Learn more about how causal analysis improves both the classification accuracy and the relevance of the concepts identified by popular concept-based explanatory models.
- Alexa enters the “age of self”
"Some of the technologies we’ve begun to introduce, together with others we’re now investigating, are harbingers of a step change in Alexa’s development — and in the field of AI itself," wrote Prem Natarajan, Alexa AI vice president of natural understanding. Read his post explaining why more-autonomous machine learning systems will make Alexa more self-aware, self-learning, and self-service.
- New take on hierarchical time series forecasting improves accuracy
The researchers' method enforces coherence, or agreement among different levels of a hierarchical time series, through projection. The plane (S) is the subspace of coherent samples; yt+h is a sample from the standard distribution (which is always coherent); ŷt+h is the transformation of the sample into a sample from a learned distribution; and ỹt+h is the projection of ŷt+h back into the coherent subspace. In a paper presented at the International Conference on Machine Learning, Amazon scientists "describe a new approach to hierarchical time series forecasting that uses a single machine learning model, trained end to end, to simultaneously predict outputs at every level of the hierarchy and to reconcile them." Read more about how this method enforces “coherence” of hierarchical time series, in which the values at each level of the hierarchy are sums of the values at the level below.
- Determining causality in correlated time series
In a paper presented at the International Conference on Machine Learning, coauthored by Bernhard Schölkopf, Amazon researchers "described a new technique for detecting all the direct causal features of a target time series — and only the direct or indirect causal features — given some graph constraints." Learn how the proposed method goes beyond Granger causality and "yielded false-positive rates of detected causes close to zero".
- How to train large graph neural networks efficiently
In a paper presented at KDD, Amazon scientists "describe a new sampling strategy for training graph neural network models with a combination of CPUs and GPUs." Learn how their method enables two- to 14-fold speedups over its best-performing predecessors.
- How to make on-device speech recognition practical
At this year’s Interspeech, Amazon scientists presented two papers describing some of the innovations that will make it practical to run Alexa at the edge. Learn how branching encoder networks make operation more efficient, while “neural diffing” reduces bandwidth requirements for model updates.
- Using learning-to-rank to precisely locate where to deliver packages
In this figure, the dark-blue circles represent the GPS coordinates recorded for deliveries to the same address. The red circle is the actual location of the customer’s doorstep. Taking the average (centroid) value of the measurements yields a location (light-blue circle) in the middle of the street, leaving the driver uncertain and causing delays. In a paper presented at the European Conference on Machine Learning, a principal applied scientist in the Amazon Last Mile organization adapts "an idea from information retrieval — learning-to-rank — to the problem of predicting the coordinates of a delivery location from past GPS data." Learn more about how models adapted from information retrieval deal well with noisy GPS input and can leverage map information.
- 3Q: Making silicon-vacancy centers practical for quantum networking
Synthetic-diamond chips with so-called silicon-vacancy centers are a promising technology for quantum networking because they’re natural light emitters, and they’re small, solid state, and relatively easy to manufacture at scale. But they’ve had one severe drawback, which is that they tend to emit light at a range of different frequencies, which makes exchanging quantum information difficult.
Members of Amazon’s AWS Center for Quantum Computing, together with colleagues at Harvard University, the University of Hamburg, the Hamburg Centre for Ultrafast Imaging, and the Hebrew University of Jerusalem, demonstrated a technique that promises to overcome that drawback. The first author on the paper, David Levonian, a graduate student at Harvard and a quantum research scientist at Amazon, answered three questions about the research for Amazon Science.
- AWS team wins best-paper award for work on automated reasoning
An example of the ShardStore deletion procedure. Deleting the second data chunk in extent 18 (grey box) requires copying the other three chunks to different extents (extents 19 and 20) and resetting the write pointer for extent 18. The log-structured merge-tree itself is also stored on disk (in this case, in extent 17). See below for details. At the ACM Symposium on Operating Systems Principles, researchers at Amazon Web Services and won a best-paper award for their work using automated reasoning to validate that ShardStore — Amazon's new S3 storage node microservice — will do what it’s supposed to. Learn more about lightweight formal methods for validating the new S3 data storage service.
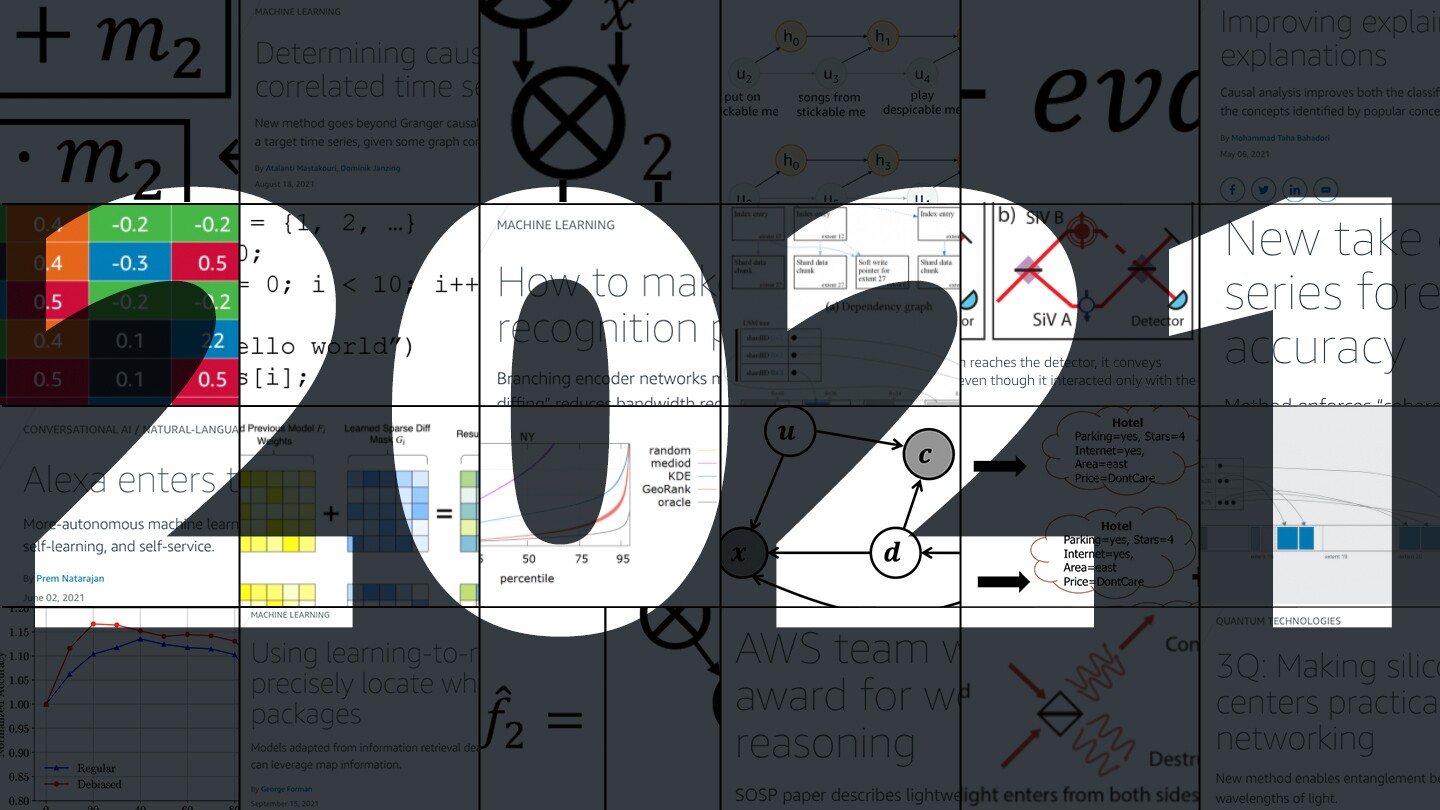