In March of 2022, Amazon and Carnegie Mellon University announced the second class of Amazon graduate research fellows, marking an expansion of the company’s efforts to help amplify the work being done by master’s-degree and PhD students. Now, the next round of fellows has been announced.
The program, which was launched in 2021, supports graduate students engaged in scientific research in automated reasoning, computer vision, robotics, language technology, machine learning, operations research, and data science.
This year’s class includes two new fellows and two who were also fellows in 2022. Fellows will also be invited to interview for science internships at Amazon.
The four fellows, and their areas of focus, are below:
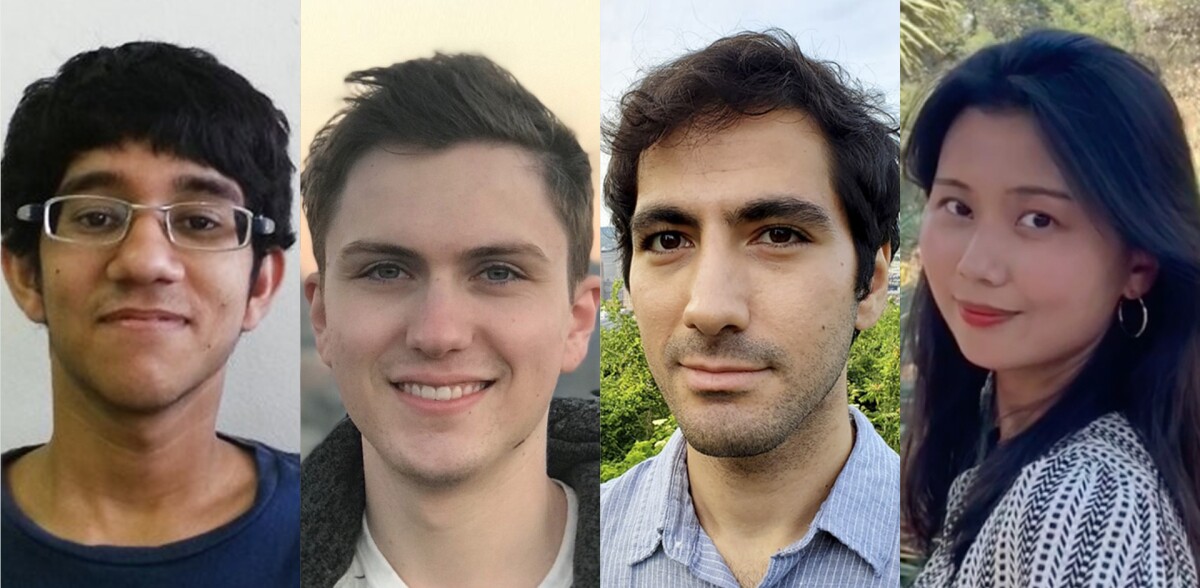
Shantanu Gupta, machine learning: Gupta is advised by Zachary Lipton, an assistant professor of operations research and machine learning who also works as a consultant for Amazon, and David Childers, assistant professor of economics.
He is researching the development of algorithms “for efficiently estimating a target parameter when we have access to multiple data sources (with an associated cost structure) returning a different subset of features. Under budget constraints, we must decide which data source to query at each time step. The methods will apply to parameters in semi-parametric models where high-dimensional nuisance parameters (e.g., propensity score) need to be estimated to estimate the target parameter (e.g., ATE).”
Ian Waudby-Smith, statistics: Waudby-Smith is advised by Aaditya Ramdas, an Amazon Visiting Academic and assistant professor in the departments of statistics and data science as well as machine learning.
His research concerns online changepoint detection algorithms “tasked with determining if and when an online stream of data has had a change in distribution. For example, it may be of interest to detect whether daily traffic on a website has substantially changed or whether users’ responses to an A/B test are nonstationary. This proposal is concerned with developing methodology and practical algorithms for tackling such problems under realistic nonparametric assumptions.”
Emre Yolcu, computer science: Yolcu’s faculty advisor is Marijn Heule, associate professor of computer science and an Amazon Scholar. Yolcu was also a fellow in 2022; this year his focus is automated reasoning and proof complexity.
“The main goal is to better understand the relative strengths of the proof systems that are designed to bring about the next step in the evolution of today’s SAT solvers by facilitating efficient proof search in proof systems stronger than resolution (the proof system underpinning the CDCL solvers). To achieve this goal, we are developing elegant and modular proof techniques that allow us to prove separations across those systems, which ultimately help point us in a principled way toward a proof system that has the ideal balance of expressivity in theory and ease of proof search in practice.”
Minji Yoon, computer science: Yoon, who was also a fellow in 2022, is advised by Christos Faloutsos, Fredkin Professor of Computer Science and an Amazon Scholar, and Ruslan Salakhutdinov, professor of computer science. She is focused on deep learning on graphs.
“The goal of this thesis is to make deep learning on graphs (DLG) more practical. To have a broader impact, we need to make DLG (1) require less hand-crafted work such as hyperparameter tuning and (2) satisfy real-world constraints, including scalability and privacy. To achieve these goals, I propose (a) an automated DLG algorithm search, (b) a generalizable neural module for graphs that transfers across different domains with minimum fine-tuning, and (c) a redefinition of conventional problem formulations that considers scalability and privacy constraints in the real world.”