Amazon Research Awards (ARA) provides unrestricted funds and AWS Promotional Credits to academic researchers investigating various research topics in multiple disciplines. This cycle, ARA received many excellent research proposals and today is publicly announcing 10 award recipients who represent 10 universities.
This announcement includes awards funded under three calls for proposals during the winter 2024 and spring 2024 cycles: AI for Information Security, Foundation Model Development and Sustainability. Proposals were reviewed for the quality of their scientific content and their potential to impact both the research community and society.
Additionally, Amazon encourages the publication of research results, presentations of research at Amazon offices worldwide, and the release of related code under open-source licenses.
Recipients have access to more than 300 Amazon public datasets and can utilize AWS AI/ML services and tools through their AWS Promotional Credits. Recipients also are assigned an Amazon research contact who offers consultation and advice, along with opportunities to participate in Amazon events and training sessions.
“Security is crucial for Amazon and AI has become instrumental in making progress in this domain. The ARA program allows us to engage with a broader academic community to tackle important problems in this intersection of AI and cybersecurity,” said Baris Coskun, senior principal scientist with GuardDuty. “The response to our AI for Cybersecurity call for proposals has been amazing and we have received a large number of high-quality proposals. We look forward to supporting the new recipients in their development of impactful new technologies that provide meaningful security value”
“The response to Amazon's first Foundation Model CFP was excellent. We awarded the largest Amazon Research Awards grant to date with $250,000 in cloud credits for work on Trainium improving foundation models. The momentum in AI is just getting stronger; with the Build on Trainium program, AWS will invest $110MM to support AI research at universities around the world,” said Emily Webber, principal solutions architect with Annapurna. “We look forward to working with exceptional PIs to develop kernels and algorithms that improve the future of AI for everyone. The scaling of model growth, in size and applications, provides a strong justification for future work at the lowest levels of the stack. There's never been a better time to dive into compute optimization for AI – join us!”
ARA funds proposals throughout the year in a variety of research areas. Applicants are encouraged to visit the ARA call for proposals page for more information or send an email to be notified of future open calls.
The tables below list, in alphabetical order by last name, winter 2024 and spring 2024 cycle call-for-proposal recipients, sorted by research area.
Spring 2024
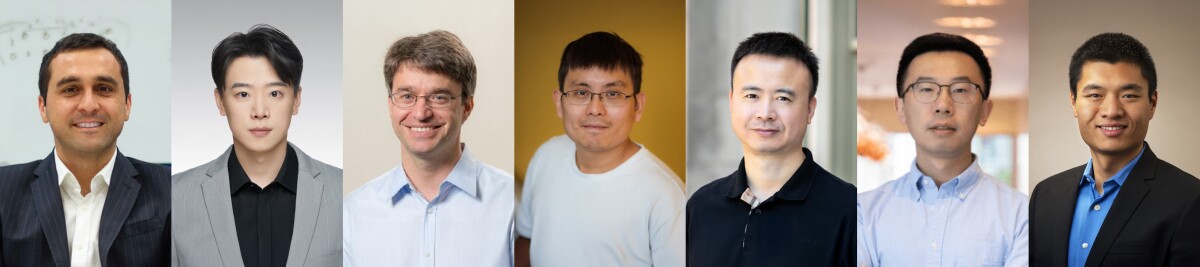
AI for Information Security
Recipient | University | Research title |
Z. Berkay Celik | Purdue University | Time-Preserving Audit Log Reduction: A Scalable Approach for Precise Attack Investigation and Anomaly Detection |
Kaize Ding | Northwestern University | Label-Efficient Graph Anomaly Detection for Information Security: Detection, Automation, and Explanation |
Christopher Kruegel | University Of California, Santa Barbara | Combating False Positives in ML-Based Security Applications With Context-Adaptive Classification |
Sijia Liu | Michigan State University | Fostering Trustworthy Generative AI: The Role of Machine Unlearning |
Chongjie Zhang | Washington University In St. Louis | Towards Practical Preference-Based Offline Reinforcement Learning for Information Security |
Yue Zhao | University Of Southern California | Label-Efficient Graph Anomaly Detection for Information Security: Detection, Automation, and Explanation |
Sustainability
Recipient | University | Research title |
Fengqi You | Cornell University | Large Language Model Co-Pilot for Transparent and Trusted LCA |
Winter 2024
Foundation Model Development
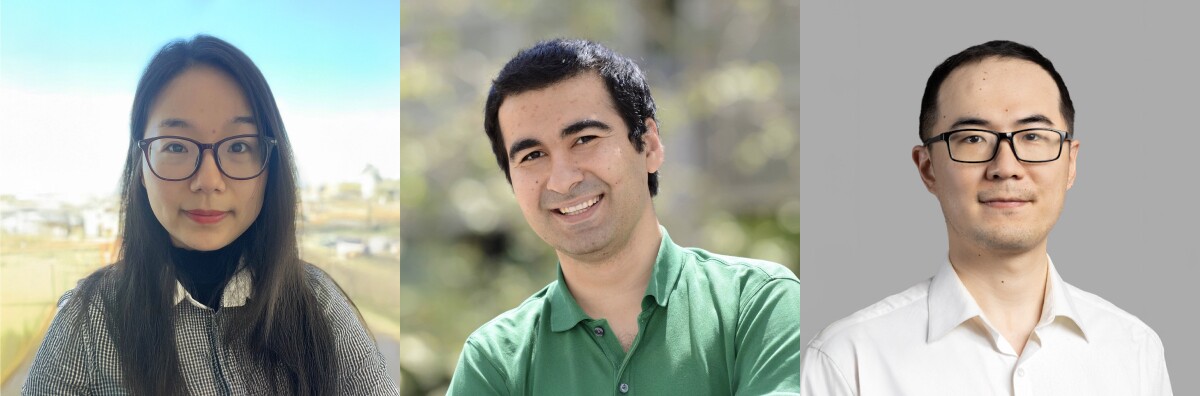
Recipient | University | Research title |
Lu Cheng | University of Illinois at Chicago | Reliable Large Language Model Alignment via Uncertainty Quantification |
Samet Oymak | University of Michigan, Ann Arbor | Beyond Transformer: Optimal Architectures for Language Model Training and Fine-tuning |
Hua Wei | Arizona State University | Reliable Large Language Model Alignment via Uncertainty Quantification |