This morning, at the annual meeting of the Association for the Advancement of Artificial Intelligence (AAAI), Michael Wooldridge, a professor of computer science at the University of Oxford and a program director at the Alan Turing Institute, gave a talk entitled “Talking to the Public about AI”. It’s a subject that Wooldridge knows a lot about, having appeared frequently on TV and radio, testified before the House of Lords, and written three popular-science books (in addition to coauthoring seven technical books). His most recent book, A Brief History of Artificial Intelligence: What It Is, Where We Are, and Where We Are Going, was published on January 19.
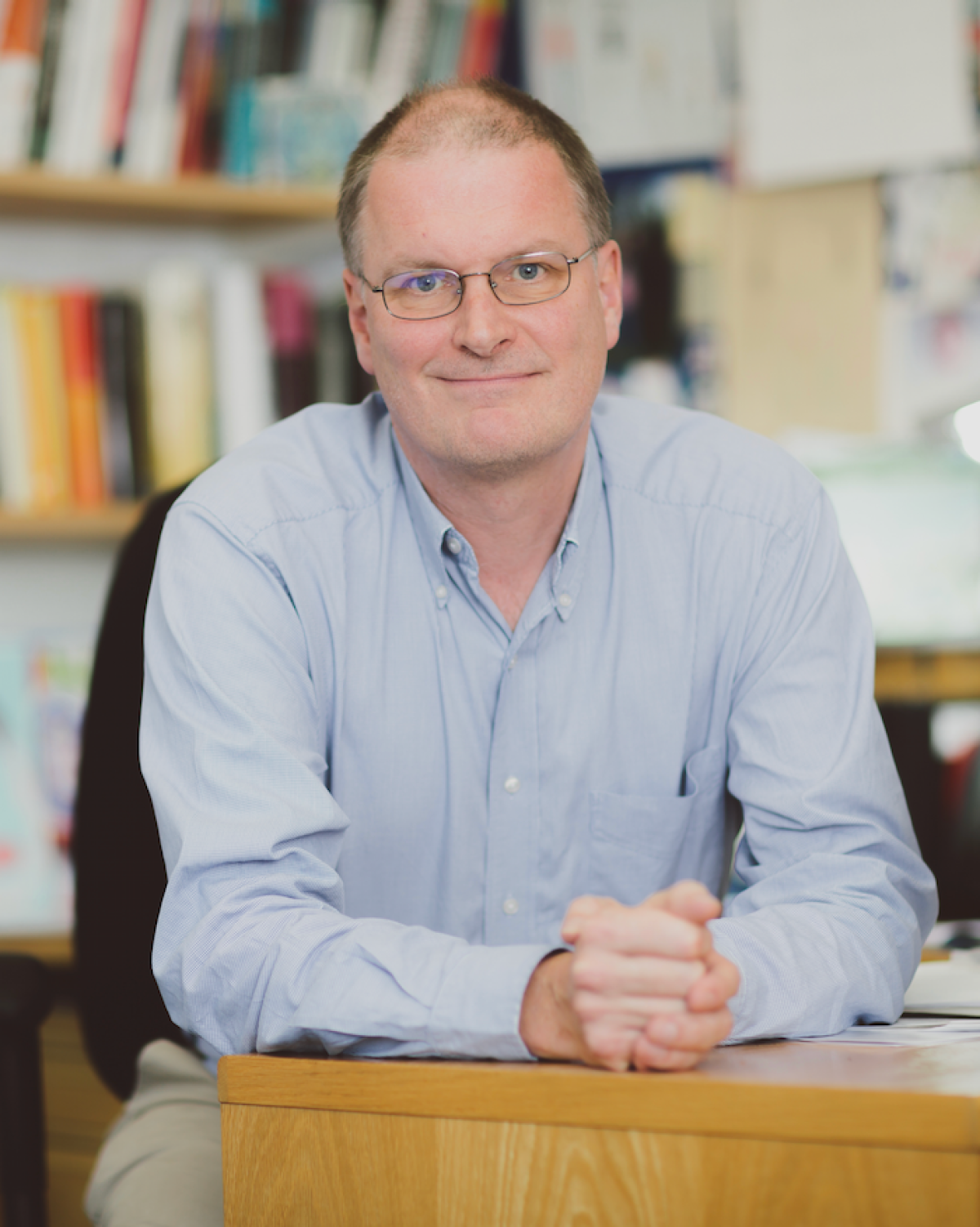
Talking to the public about AI is also a passion of Zachary Lipton, an Amazon Web Services scientist and the BP Junior Chair Assistant Professor of Operations Research and Machine Learning at Carnegie Mellon University. In 2016, Lipton created the Approximately Correct blog as a forum for examining both social and technical questions surrounding AI, and in 2018, he delivered a talk at MIT Technology Review’s Emerging Technologies conference titled “Machine Learning: The Opportunities and the Opportunists”.
As AAAI 2021 approached, Lipton and Wooldridge joined Amazon Science to share their perspectives on the topic of public communication around AI.
Amazon Science: What are the difficulties in talking about AI?
Mike Wooldridge: The reality of AI is a long, long, long way away from how it’s often portrayed. The portrayal often divides neatly into either dystopia or utopia. I think there's tons to be excited about; this is clearly the most exciting time that I've seen, and I've been in the game since the 1980s. There are also things that we should be worried about. One of the reasons that I decided to write A Brief History of Artificial Intelligence was to try to reframe the narrative a little bit.
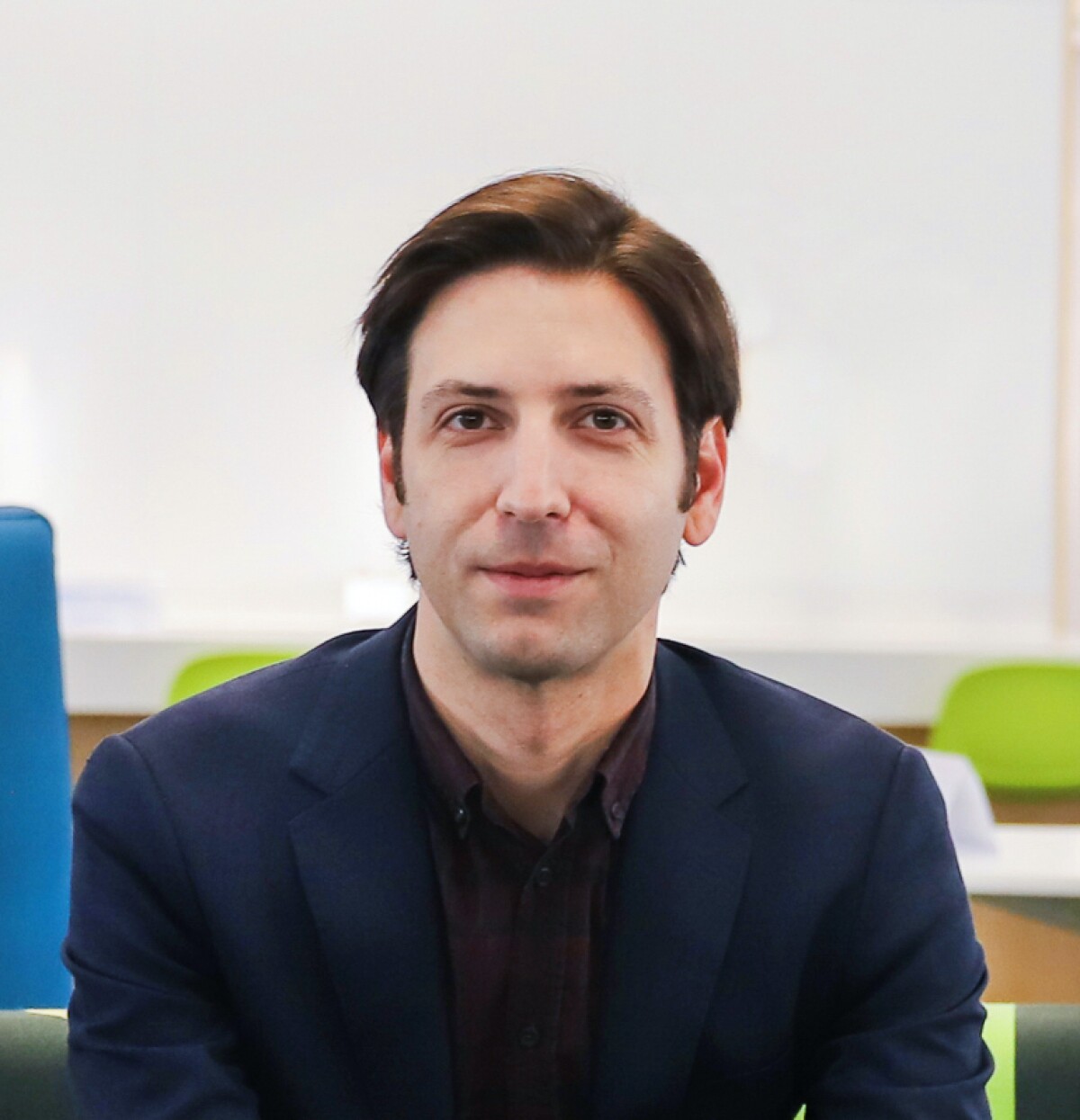
Zack Lipton: There's a distinction between the nature of the technology and the capabilities of technology, and I think there's a lot of confusion about both of them.
Neural-network algorithms, together with very large data sets and parallel computation, are really good at something called function fitting — learning to make predictions or to infer statistical relationships. And it turns out that there are a lot of tasks, even ones that might not be so intuitive, that you can frame as prediction problems. For example, machine translation: given some representation of a sentence in English, you could try to predict what's most likely to be the first word of the corresponding translation in French; then, given the input sentence and the first word, try to predict the second word; and so forth.
But if you have what we thought was a human capacity for doing translation, people start making leaps to all sorts of other things that humans do that are not necessarily as easily cast as prediction problems. A very natural one is making decisions. So people start saying, Well, machine learning is going to completely change medicine. But it turns out that decision making involves all kinds of considerations that aren't just straight-up prediction problems. You need to learn something about causal effects. And this is an area where we're trailing far behind where we are in prediction.
AS: Mike, what is the reframing you're attempting in the book?
MW: Where the book came from is, in 2014, I was sat at my desk in my office in Oxford, and the phone rang, and it was a news station looking for an expert on AI, because Stephen Hawking says AI might be the end of humanity. And I declined the interview, because I just assumed there was somebody smarter and more eloquent out there who was going to answer the call. But I started to see these stories appearing, and they were in a vacuum. There was no response.
So I finally decided I wanted to be one of the people who responded. Reframing the narrative is trying to say, Look, AI isn't necessarily what you think it is. The stuff that is so exciting from the movies and books is kind of on the fringes. The stuff that you lose sleep about is not necessarily what you should be losing sleep about.
But that doesn't mean there isn't anything you should be worried about. The famous one, which is going to be with us for a while, is bias. I think the AI community and machine learning community are genuinely working very hard to try to understand how bias arises and how to mitigate the risks of those biases.
ZL: I think, arguably, as much as the interest has ballooned, it topped out around 2018 and has been somewhat level since then. At the same time, I think there's also a lot more supply. It's not like there's only 300 people out there really working in deep learning. I think if you were active in the space in 2013, 2014, you probably started getting a lot of attention, where someone with a comparable level of experience and accomplishment in 2020 might have considerably less focus on them.
There is something interesting that the things we're excited about in 2020 and 2021 are not really qualitatively different than in 2015, 2016, 2017, right? I think it is a telling sign that the things that we're excited about are more or less the stuff we already got to work in 2016, just trained on bigger datasets.
I started to see these stories appearing, and they were in a vacuum. There was no response. So I finally decided I wanted to be one of the people who responded.
MW: Neural-net research really hit tough times by the mid-’90s. But the story there is, it just hit the limits of what computers could do. So there is a theory that the progress that we've seen will plateau for exactly that reason: it will just hit the limits. Not of the science, but just of the technology. And without fundamental new ideas to drive it forward — and it would require some quite big ideas in terms of training — we might just well hit the plateau in the next few years.
AS: If we are on a plateau, what do you see on the horizon?
ZL: I’ll give an analogy: Arguably, before AI was a big commercial interest, databases were, and after databases, an early kind of data mining. But databases never stopped being important. Maybe they weren't generating as revolutionary developments as in the past, but that doesn't mean we stop using them. They just stopped being where the action was.
I don't think neural networks are going away. I don't think we have a rival technology that is vying to replace neural networks as the best way for estimating things like functions that assign categories to images or make complicated predictions based on language data.
What I do see happening is it just not being where the action is. And I think this is already starting to happen. So I could say where I think I think the action is.
Almost all machine learning, including deep learning, proceeds under this idea that you have some fixed, static world that's throwing off data, and you're collecting the data and trying to find a function that does something useful. And that's just not the world that we live in. In the world that we live in, data is constantly coming in. It's coming from a variety of sources. It's becoming obsolete. The world is changing in various ways, and how to function in the world — even just making predictions — is actually a whole different kind of concern that requires that we think about this outer loop and what's going on in the environment.
Almost all machine learning ... proceeds under this idea that you have some fixed, static world that's throwing off data .... And that's just not the world that we live in.
In my work at CMU with my lab, this is where we have been driving a lot of our attention: to consider whether it's just a passively changing world or actually a world that's responding. For example, if you have a policy for making decisions, people will be strategic, and they'll start behaving differently. How you build technology that is suitable for a changing world and accounts for the fact that you are part of a dynamic environment, to me, that's where the action is already moving.
MW: I think we're overlapping in our answers in at least one respect, which is, what I'm disappointed about is not having enough AI in the physical world, the world that we all inhabit. And there's a number of reasons for that. For example, reinforcement learning is one of the technologies that underpins the breakthrough Atari-playing programs. The thing is just playing endless games against itself. When you're playing against space invaders, it doesn't matter if you make a mistake.
In the real world, it matters. So you can't do driverless-car technology, for example, with reinforcement learning. The natural answer to that is, well, you need high-fidelity simulators. That's what everybody's doing, naturally enough, but it will only take you so far. I want to see programs that could really learn how to do things in the physical world. That for me would be exciting.
The other thing — and again, it’s overlapping with what you said — is we know from experience with adversarial examples how brittle this technology is. We can only trust the technology so far until we understand where that brittleness lies and what the limits of it are. Understanding that is going to be quite crucial. If we don't get to that, then we're always going to be nervous about this technology whenever it's used outside scenarios like game playing. So those are the two things that I'm really excited about. At least this afternoon.