What if artificial intelligence could help an aspiring author write a novel? Or coach people to improve the quality of their writing? Could machines learn how to make jokes? Inspired by these questions, computer scientist Jiao Sun has been exploring the potential of AI-generated text as a PhD candidate at the University of Southern California (USC).
After a four-month internship at Alexa AI last spring, she is now starting her journey as an Amazon Machine Learning Fellow for the 2022–23 academic year and hopes to continue developing text-generation models that enhance the interaction between humans and AI.
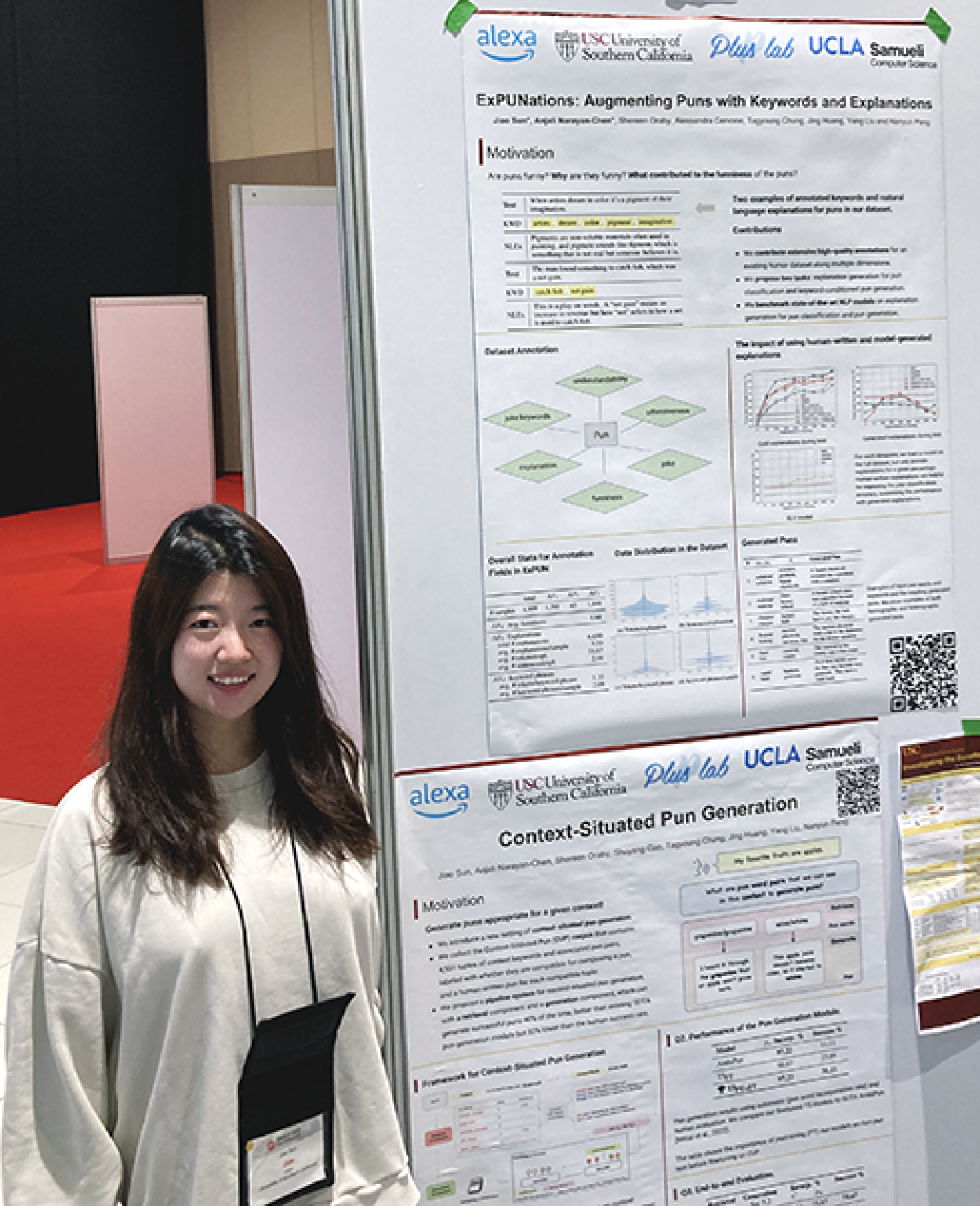
While Sun is passionate about the potential of natural language generation, she also believes it’s important to develop tools that improve human control over machine-created content. She is also cautiously optimistic about the surge in popularity surrounding text generation models.
“I am thrilled to see more and more great models in the space of text generation in recent years,” she says. “It can help spur more innovation for text generation field, but might also obsolete some research, and even some research directions. Personally, my research philosophy is to work on research that is agnostic to model choices and creative by itself.”
One of her research goals is to improve the quality, fairness, and reliability of that content to achieve what she calls trustworthy text generation.
For example, she and her colleagues recently investigated the presence of gender stereotypes in greeting card messages written by both humans and machines. The research — which received the Best Paper Honorable Mention in the 2022 CHI Conference on Human Factors in Computing Systems, an international conference on human-computer interaction — led to the development of a writing assistant tool to combat those biases.
“This is so important, because we can see that machines have the potential to generate cool things, but we don’t want them to freely create whatever they want,” Sun says. “We want to make sure that the content machines are generating is fair and grounded by knowledge, and we want humans to have control over that output.”
Protecting authors’ privacy
Sun is still in the early stages of her fellowship, but one area of research she would like to explore during the program is using AI to ensure author privacy, which she sees as another aspect of trustworthy text generation.
She notes that natural language processing techniques can be used to infer the authorship of articles and documents based on the author’s writing style, especially if the author has multiple articles published online.
But what if, for some reason, the author wants to remain anonymous?
“We're thinking about ways we can rewrite something in a way that maintains the semantics from your text while keeping the authorship protected,” Sun says. The idea is to develop AI models that rephrase contents to remove stylistic fingerprints that could give away who the author is.
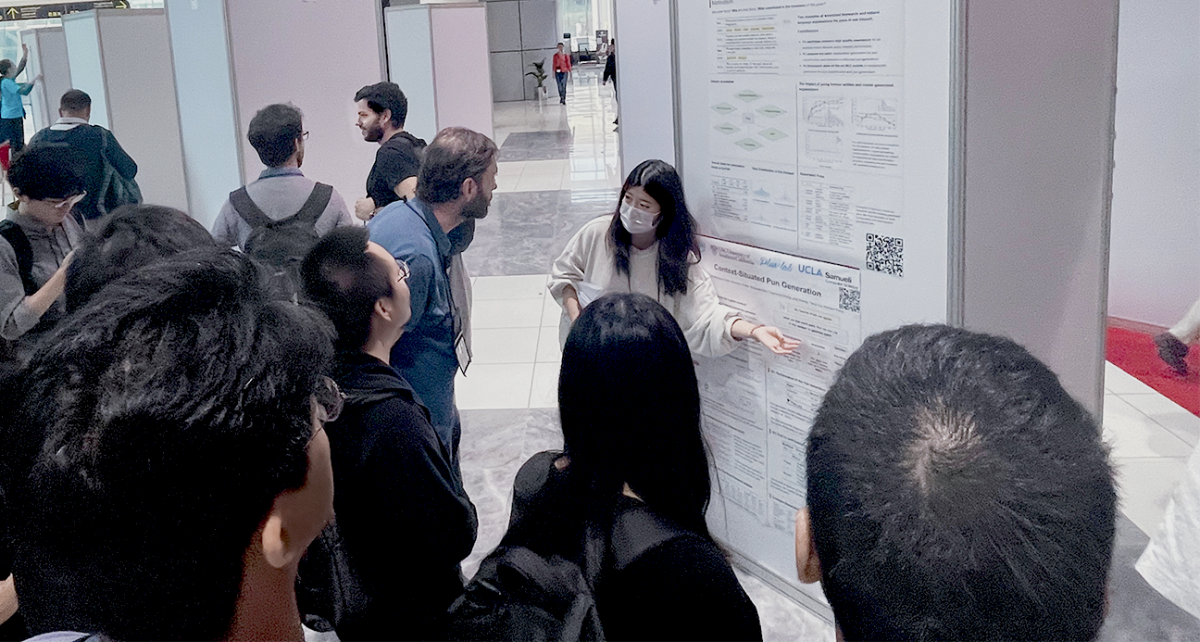
During the program, Sun is being mentored by Qian Hu, an applied scientist at Amazon Alexa AI, with whom she connects regularly to discuss her research.
“That is not only helpful for my career, but just having this connection with another smart person helps me shape my research in the right direction,” she says.
The Amazon Machine Learning Fellowship is a program offered annually to doctoral students by the USC + Amazon Center on Secure and Trusted Machine Learning, a joint research center focused on the development of new approaches to ML privacy, security, and trustworthiness. In addition to Sun, Sina Shaham and Yunhao Ge are also ML Fellows this academic year.
‘What did the sushi say to the bee?’
During her internship at Amazon last spring, Sun worked with Amazon scientists Alessandra Cervone, Anjali Narayan-Chen, Tagyoung Chung, Shuyang Gao, Jing Huang, Yang Liu, Shereen Oraby, and Amazon Visiting Academic Violet Peng on two papers that were accepted at the 2022 Conference on Empirical Methods in Natural Language Processing (EMNLP).
Are you excited about pun generation? In #EMNLP2022, we have two works accepted in the main conference:
— Jiao Sun ✈️ EMNLP 2022 (@sunjiao123sun_) December 8, 2022
1️⃣ Context-Situated Pun Generation 👉 a brand-new task!
2️⃣ ExPUNations: Augmenting Puns with Keywords and Explanations 👉 a new dataset!
Learn more! 🧵👇
Both works are done during my internship @AmazonScience with awesome @VioletNPeng @anjalisaa @shrnrby @Ale_Cervone @iuaaui Yang Liu, Tagyoung Chung and Jing Huang!
— Jiao Sun ✈️ EMNLP 2022 (@sunjiao123sun_) December 8, 2022
“During my internship, they gave me a lot of really precious feedback. And they have continued to support me, even after my internship ended.”
Both papers explore the challenging task of explaining humor to machines. Sun notes that we often take for granted the knowledge required to understand simple puns. But imagine having to explain a play on words to a non-native speaker or a small child.
“For machines to understand jokes, they need to learn from a huge knowledge base,” she says.
Sun and her coauthors first developed a dataset of pun keywords and explanations, which was appropriately named ExPUNations. She worked on an existing dataset of puns, asking annotators to evaluate whether a given text was intended to be a joke, how funny it was to them, and what about it was funny.
Take the joke: “What did the sushi say to the bee? ‘Wasabi.’” “If I were the annotator, I would say this is funny because wasabi sounds like ‘What’s up, bee?’ That's the funniness of it,” Sun says. The annotators were also asked to select the keywords of the pun. In this case, those would be “sushi,” “bee,” and “wasabi.”
“We collect not only an explanation of the pun itself but also the essential facts for a human to do the reasoning into that explanation,” Sun says. The result was an augmented dataset that can be used to train models to explain puns and also generate new puns based on keywords.
The second work Sun developed as an Amazon intern aimed to generate puns based on given contexts. She and her coauthors explain that previous pun-generation studies usually elect a given pun word as a starting point to generate an appropriate joke. In her research, instead, the starting point is the context, a given scenario in which the joke would take place. The initial goal is to identify pun words that would fit that context and then generate a pun appropriate to that scenario.
“After we have that pun word, we have the context and the pun word combined to generate a really funny pun,” Sun says.
Human evaluation showed that 69% of the pun words retrieved by the system could be used to generate context-situated puns. For plausible pairs of context and pun words, the system was able to generate successful puns 31% of the time.
Thanks to an Amazon travel grant, Sun was able to present her research in person in Abu Dhabi at EMNLP.
“This grant gave me the opportunity of traveling to what was my first in-person conference in my entire PhD,” she says. “It has been three years since we’ve been in this pandemic, so I really appreciate it. And I will be graduating soon, so it’s a great opportunity for me to meet my peers.”
Sun believes that this type of research could enhance people’s engagement during interactions with AI.
“Wouldn’t it be cool if you were talking with Alexa and it could understand the context and tell you a joke that was appropriate to that context?” she imagines.